Navigation Menu
Search code, repositories, users, issues, pull requests..., provide feedback.
We read every piece of feedback, and take your input very seriously.

Saved searches
Use saved searches to filter your results more quickly.
To see all available qualifiers, see our documentation .
- Notifications You must be signed in to change notification settings
An Open-Ended Embodied Agent with Large Language Models
MineDojo/Voyager
Folders and files, repository files navigation, voyager: an open-ended embodied agent with large language models.
[Website] [Arxiv] [PDF] [Tweet]
We introduce Voyager, the first LLM-powered embodied lifelong learning agent in Minecraft that continuously explores the world, acquires diverse skills, and makes novel discoveries without human intervention. Voyager consists of three key components: 1) an automatic curriculum that maximizes exploration, 2) an ever-growing skill library of executable code for storing and retrieving complex behaviors, and 3) a new iterative prompting mechanism that incorporates environment feedback, execution errors, and self-verification for program improvement. Voyager interacts with GPT-4 via blackbox queries, which bypasses the need for model parameter fine-tuning. The skills developed by Voyager are temporally extended, interpretable, and compositional, which compounds the agent’s abilities rapidly and alleviates catastrophic forgetting. Empirically, Voyager shows strong in-context lifelong learning capability and exhibits exceptional proficiency in playing Minecraft. It obtains 3.3× more unique items, travels 2.3× longer distances, and unlocks key tech tree milestones up to 15.3× faster than prior SOTA. Voyager is able to utilize the learned skill library in a new Minecraft world to solve novel tasks from scratch, while other techniques struggle to generalize.
In this repo, we provide Voyager code. This codebase is under MIT License .
Installation
Voyager requires Python ≥ 3.9 and Node.js ≥ 16.13.0. We have tested on Ubuntu 20.04, Windows 11, and macOS. You need to follow the instructions below to install Voyager.
Python Install
Node.js install.
In addition to the Python dependencies, you need to install the following Node.js packages:
Minecraft Instance Install
Voyager depends on Minecraft game. You need to install Minecraft game and set up a Minecraft instance.
Follow the instructions in Minecraft Login Tutorial to set up your Minecraft Instance.
Fabric Mods Install
You need to install fabric mods to support all the features in Voyager. Remember to use the correct Fabric version of all the mods.
Follow the instructions in Fabric Mods Install to install the mods.
Getting Started
Voyager uses OpenAI's GPT-4 as the language model. You need to have an OpenAI API key to use Voyager. You can get one from here .
After the installation process, you can run Voyager by:
- If you are running with Azure Login for the first time, it will ask you to follow the command line instruction to generate a config file.
- Select Singleplayer and press Create New World .
- Set Game Mode to Creative and Difficulty to Peaceful .
- After the world is created, press Esc key and press Open to LAN .
- Select Allow cheats: ON and press Start LAN World . You will see the bot join the world soon.
Resume from a checkpoint during learning
If you stop the learning process and want to resume from a checkpoint later, you can instantiate Voyager by:
Run Voyager for a specific task with a learned skill library
If you want to run Voyager for a specific task with a learned skill library, you should first pass the skill library directory to Voyager:
Then, you can run task decomposition. Notice: Occasionally, the task decomposition may not be logical. If you notice the printed sub-goals are flawed, you can rerun the decomposition.
Finally, you can run the sub-goals with the learned skill library:
For all valid skill libraries, see Learned Skill Libraries .
If you have any questions, please check our FAQ first before opening an issue.
Paper and Citation
If you find our work useful, please consider citing us!
Disclaimer: This project is strictly for research purposes, and not an official product from NVIDIA.
Contributors 12
- JavaScript 66.5%
- Python 33.5%
Behold Nvidia's Giant New Voyager Building
The graphics and AI company's 750,000-square-foot building is designed to give employees a good place to work. CNET got the exclusive photographic tour.

Nvidia Voyager Building's Base Camp
Nvidia's Voyager building is designed to be a place where employees are eager to show up for work. Immediately after entering the 750,000-square-foot building at the graphics and AI chipmaker's Santa Clara, California, campus, you see its "base camp" a reception area. It's at the foot of the darker "mountain" that climbs upward behind it.

Approaching Nvidia Voyager Building
The walkway leading from Nvidia's older Endeavor building to the newer Voyager is lined with trees and shaded by solar panels on aerial structures called the "trellis."
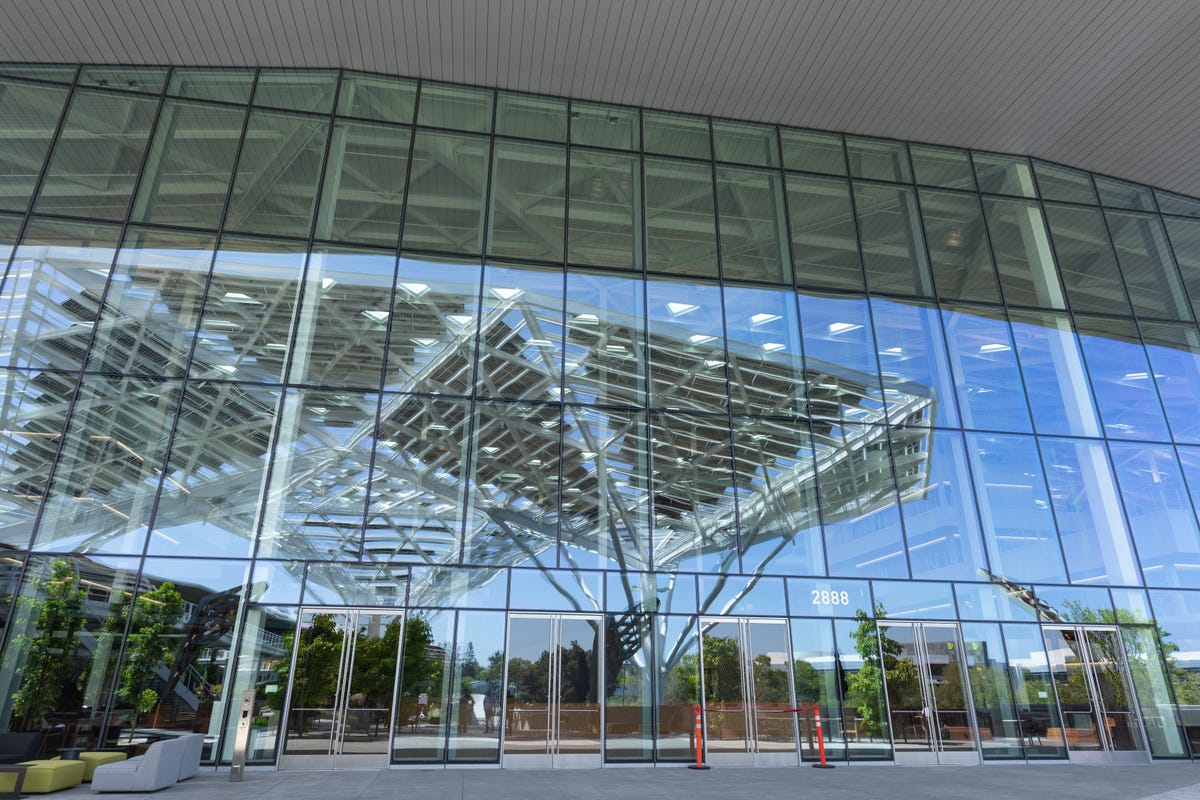
Nvidia Voyager Building Front Facade
The towering glass front of Nvidia's Voyager building reflects the "trellis" outdoors that provides shade to the front of the building.
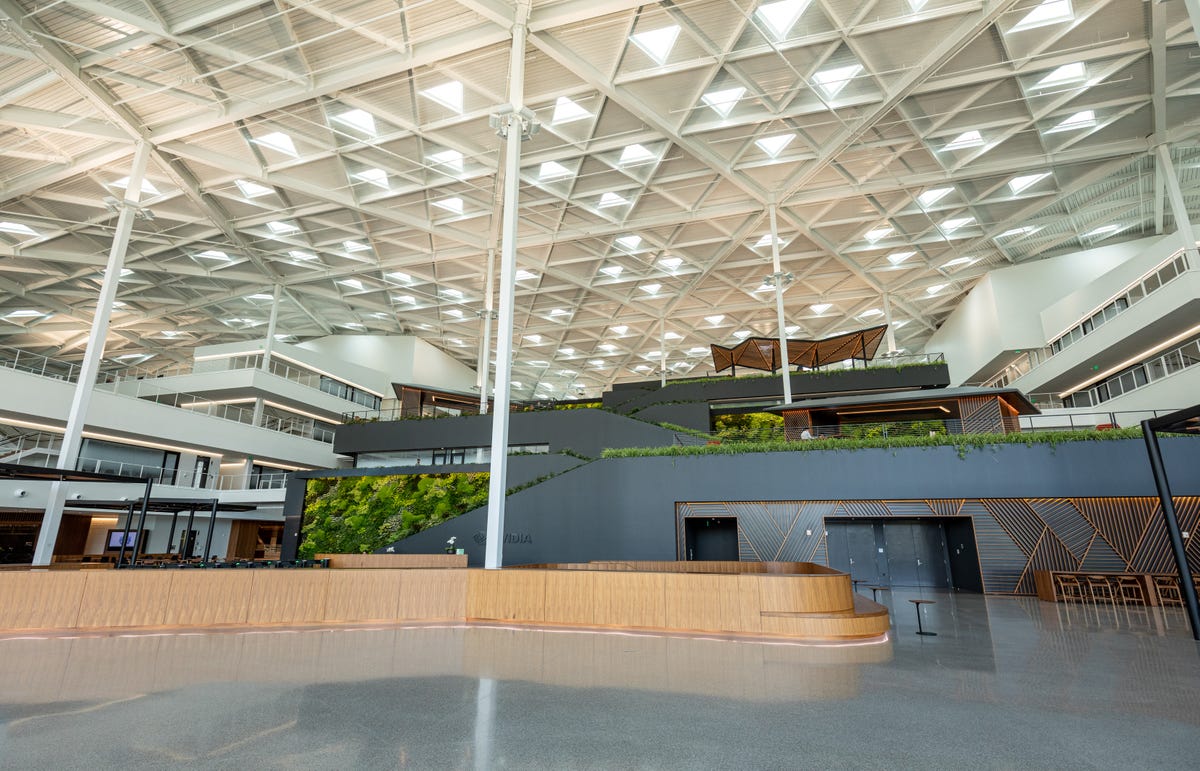
Nvidia Voyager Building's Mountain
The central part of Voyager is the "mountain," where employees can meet, work and gaze at the view. A stairway leads up the mountain's front face, and "valleys" to either side separate it from more conventional offices.
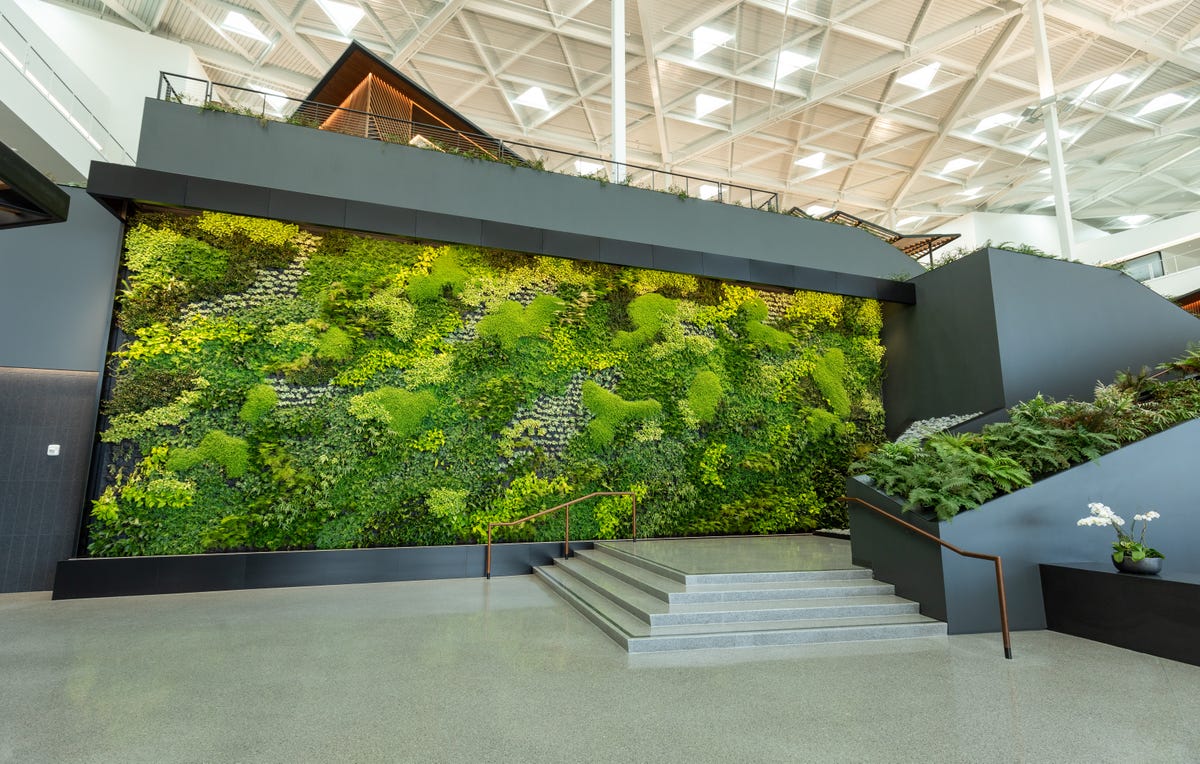
Nvidia Voyager's Green Walls
In Nvidia's Voyager building, walls covered with native plants give the mountain a more organic look, freshen the air and absorb sound.

Nvidia Endeavor and Voyager Buildings From Above
Nvidia's Santa Clara headquarters includes its 500,000-square-foot Endeavor building, left, and newer 750,000-square-foot Voyager to the right. A private walkway connects Endeavor to other Nvidia buildings out of view to the right.
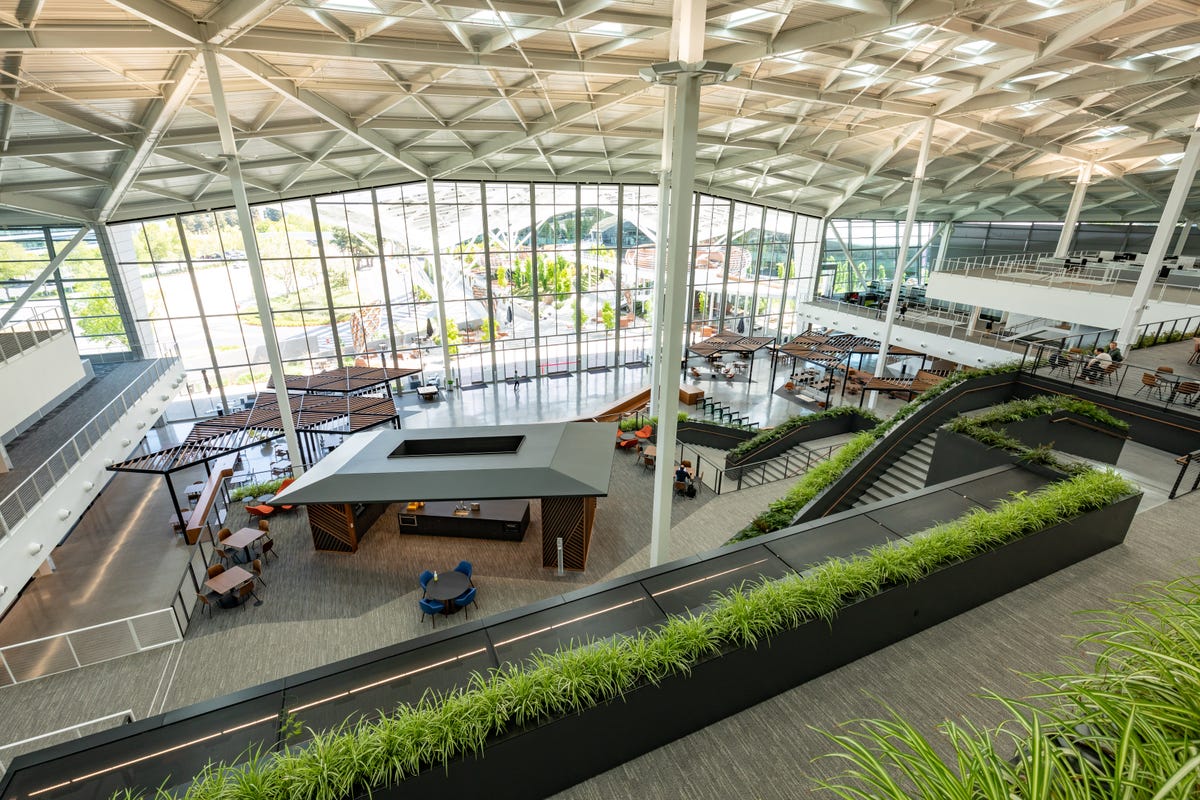
Nvidia Voyager Building
From the top of the Nvidia Voyager building's mountain, you can see the stairway, the "base camp" reception area and the building's glass front.
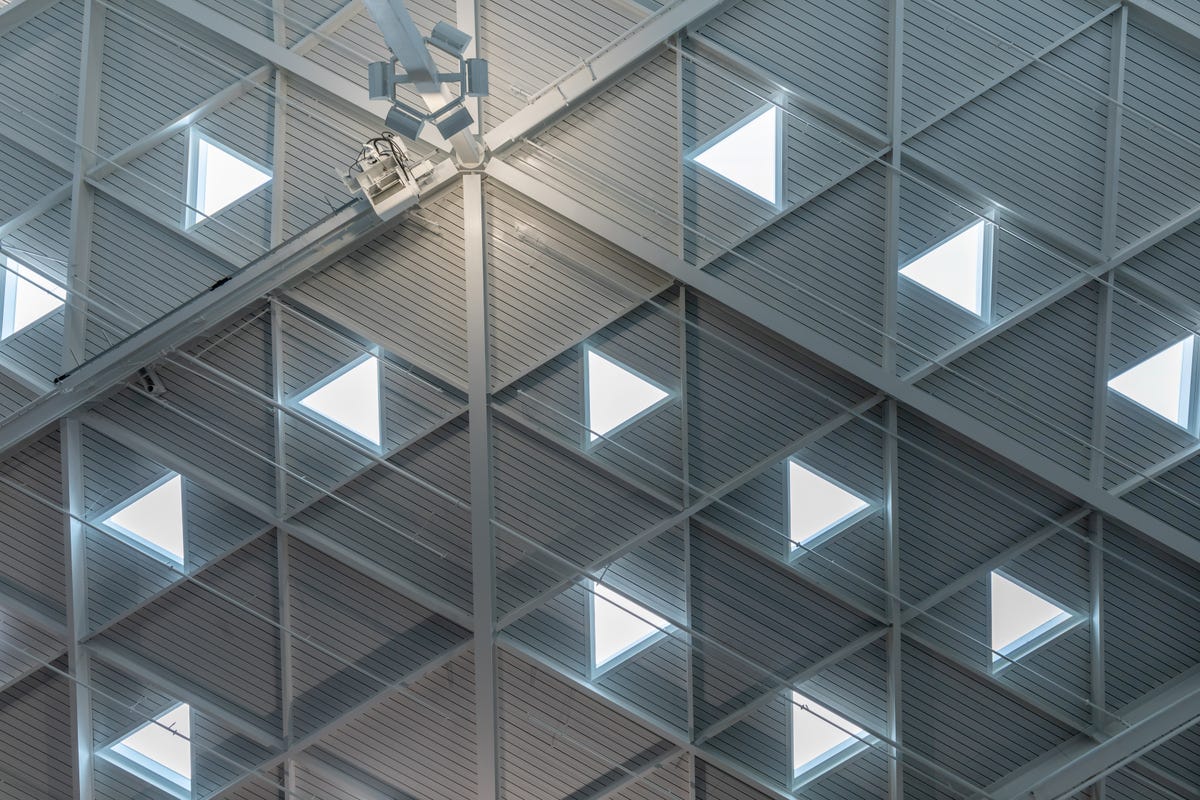
Nvidia Voyager Building Roof
Far overhead in Nvidia's Voyager building is a roof pierced with many triangular skylights. The geometrical patterns are a nod to the wireframes at the heart of Nvidia's computer graphics business, but the effect is used sparingly compared with the overwhelmingly polygonal styling of Nvidia's earlier Endeavor building next door.
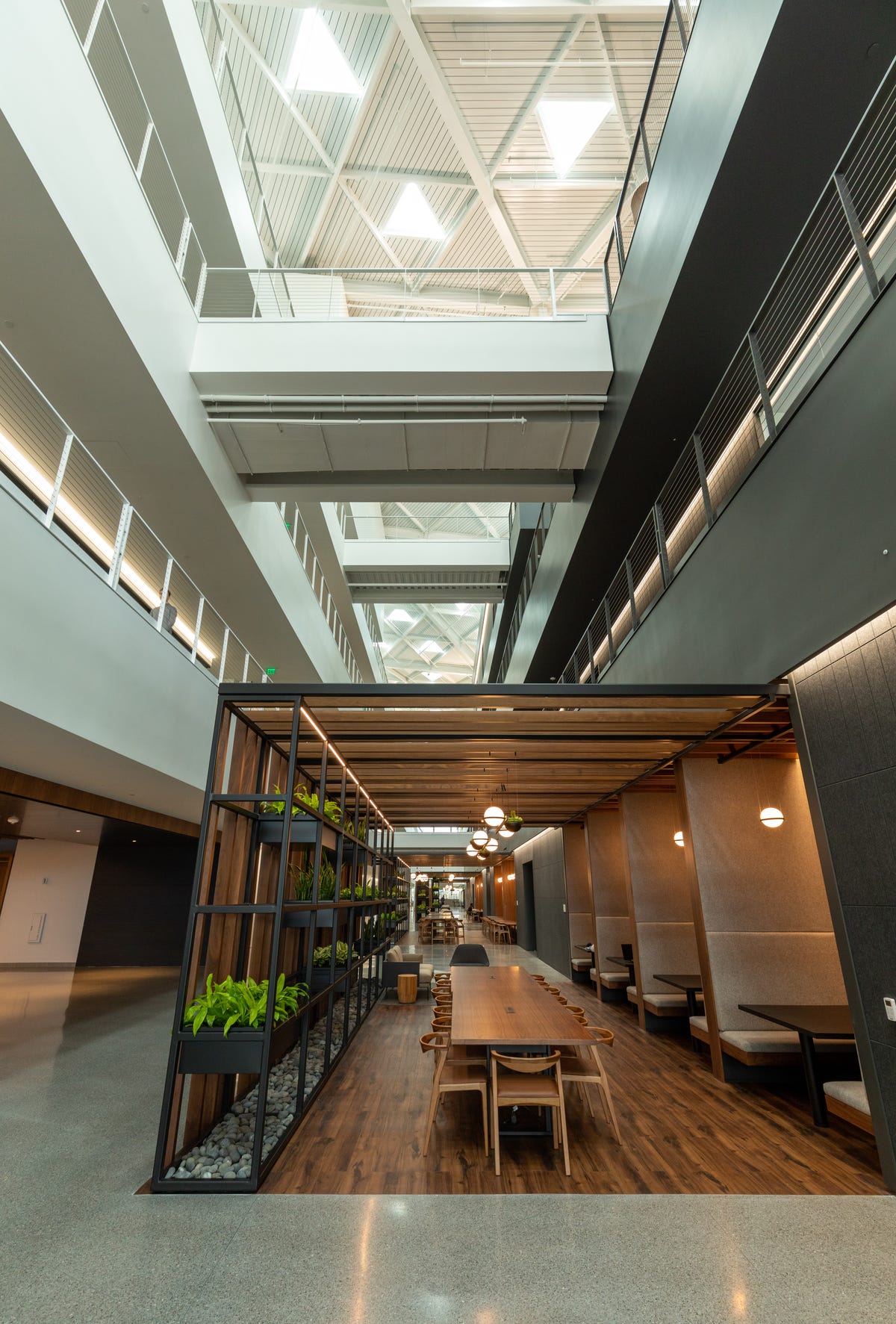
Nvidia Voyager Valleys
"Valleys" divide the mountain, right, from more conventional offices while allowing natural light to penetrate to the ground floor. Booths and tables are open for employees to meet or eat lunch.

Nvidia Voyager Building's Volcanic Plug
Atop the Voyager building's mountain is a multifaceted black structure reminiscent of a basalt from an extinct volcano. Nvidia had to reshape it several times to get the facets to show properly.

Nvidia Voyager Building, Back of the Mountain
The back of Voyager features an amphitheater where employees can watch events like company meetings.

Nvidia Voyager Building's Caldera
A long live-edge table is at the center of a cozy recessed area called the caldera near the top of the mountain in Nvidia's Voyager building. In real-world geophysics, a caldera is a sunken crater left after an eruption empties a volcano's magma chamber.
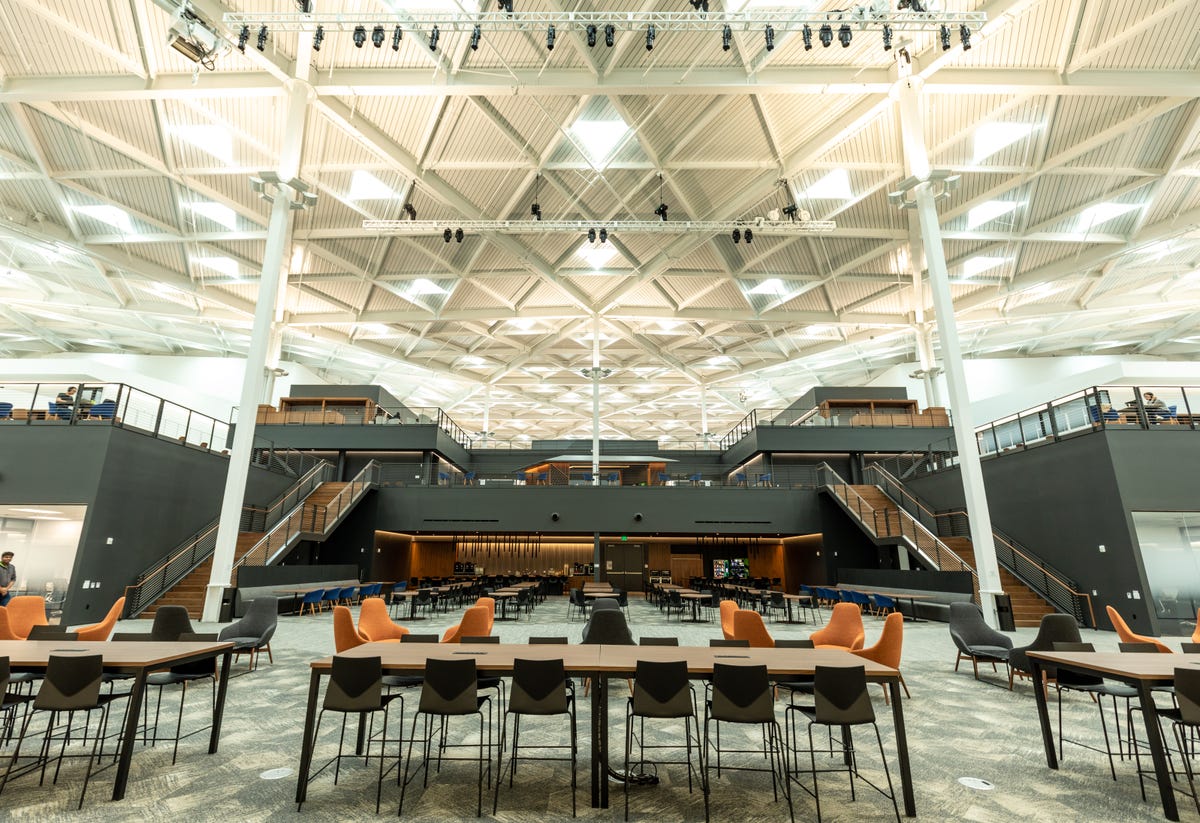
This view looks upward from the stage area of the amphitheater up the back of the "mountain" in Nvidia's Voyager building.
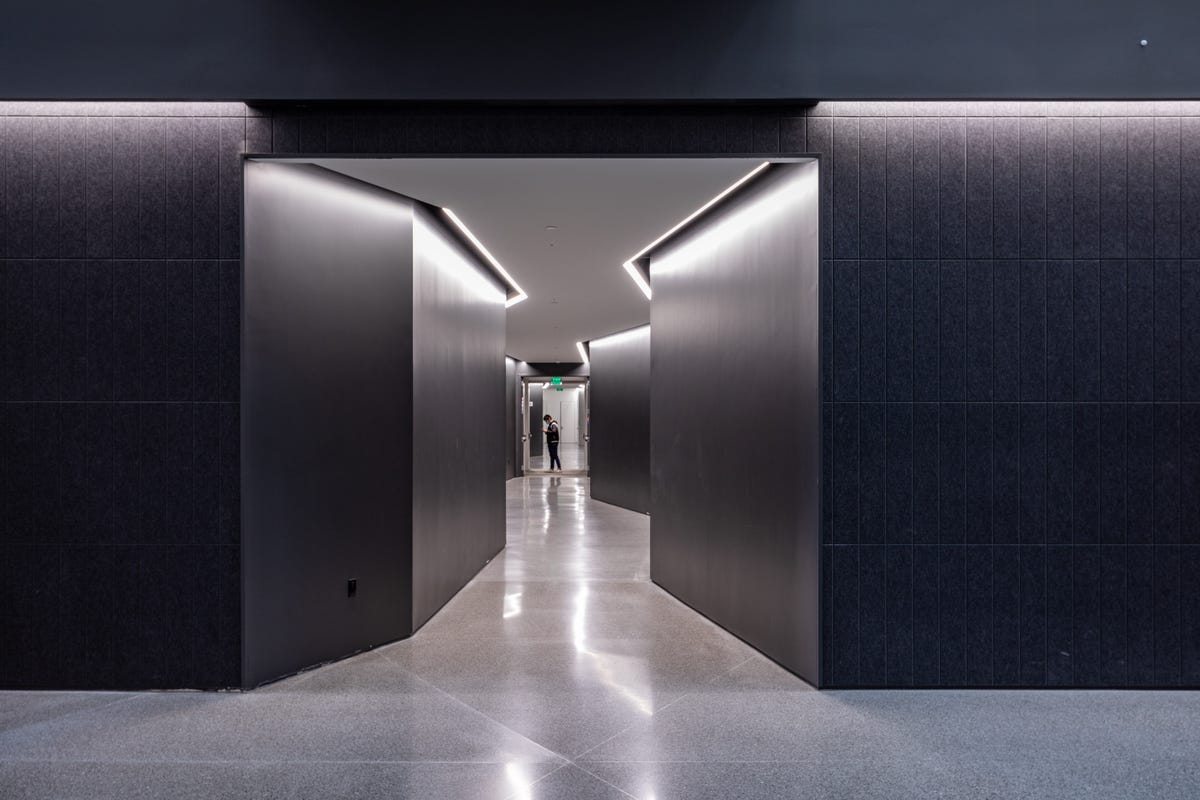
Under the Mountain in Nvidia Voyager
This almost subterranean channel tunnels through the mountain like a lava tube.
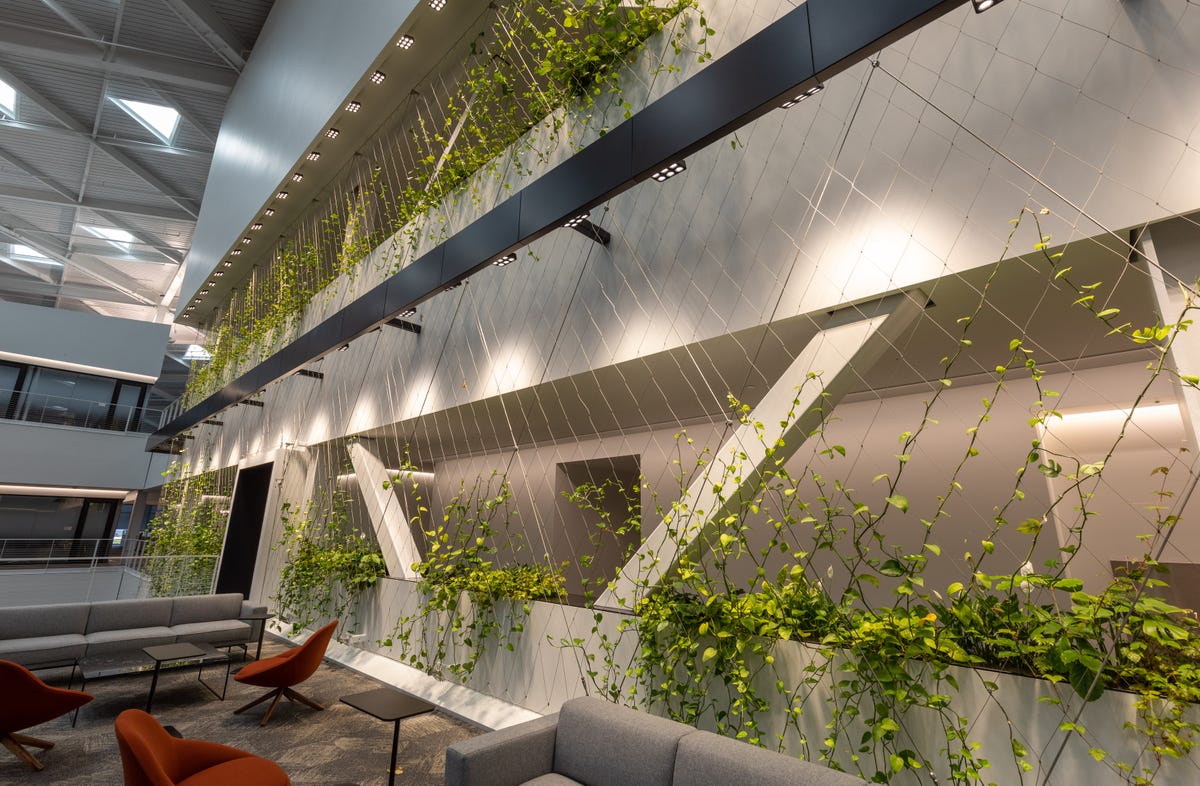
Nvidia Voyager Building Verdure
Creeping plants are trained to grow up wires to provide a green backdrop for events held on the back of the mountain area of Nvidia's Voyager building.
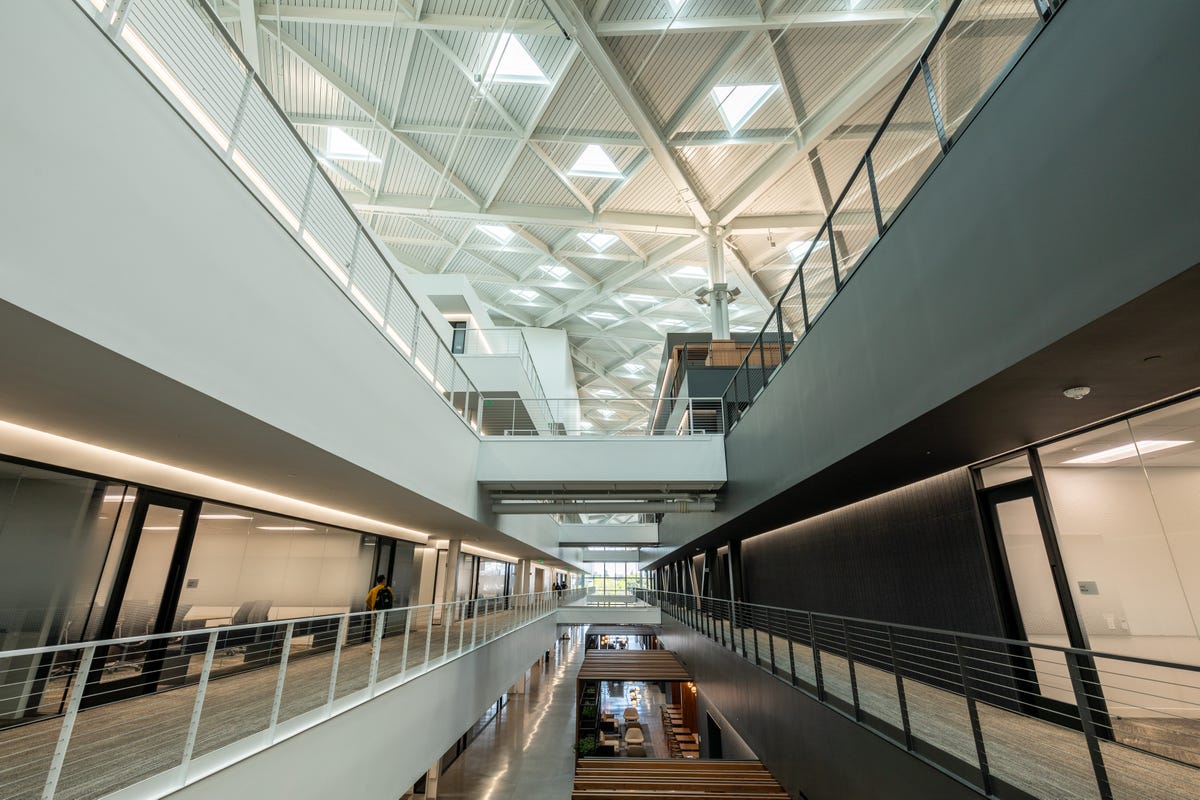
Nvidia Voyager Building Valley
Nvidia's Voyager building uses different colors to distinguish the dark mountain from the lighter conventional offices on the other side of the "valley."
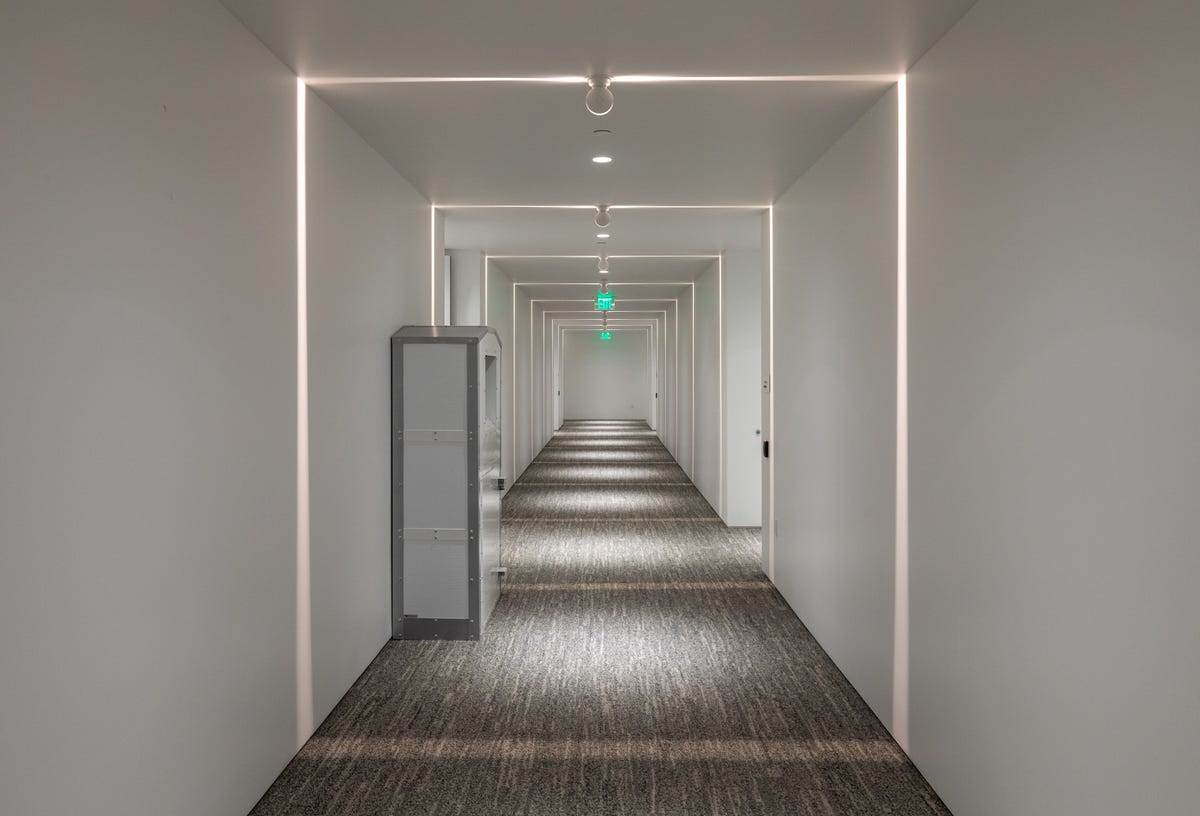
Nvidia Voyager Building Tunnels
Unusual lighting gives otherwise ordinary corridors a fresh look deep beneath the mountain at the center of Nvidia's Voyager building.
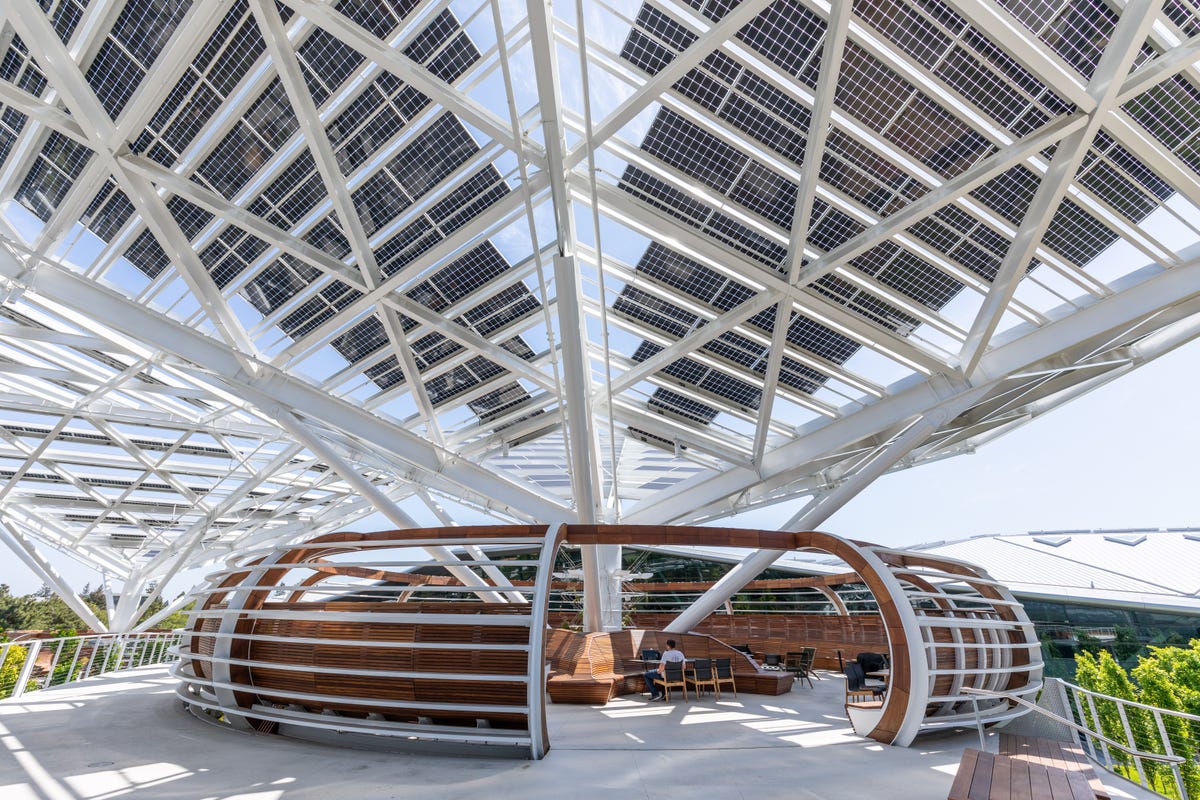
Nvidia Voyager Building Bird Nest
Outside Nvidia's Voyager building are elevated "bird nests" where people can work and meet.
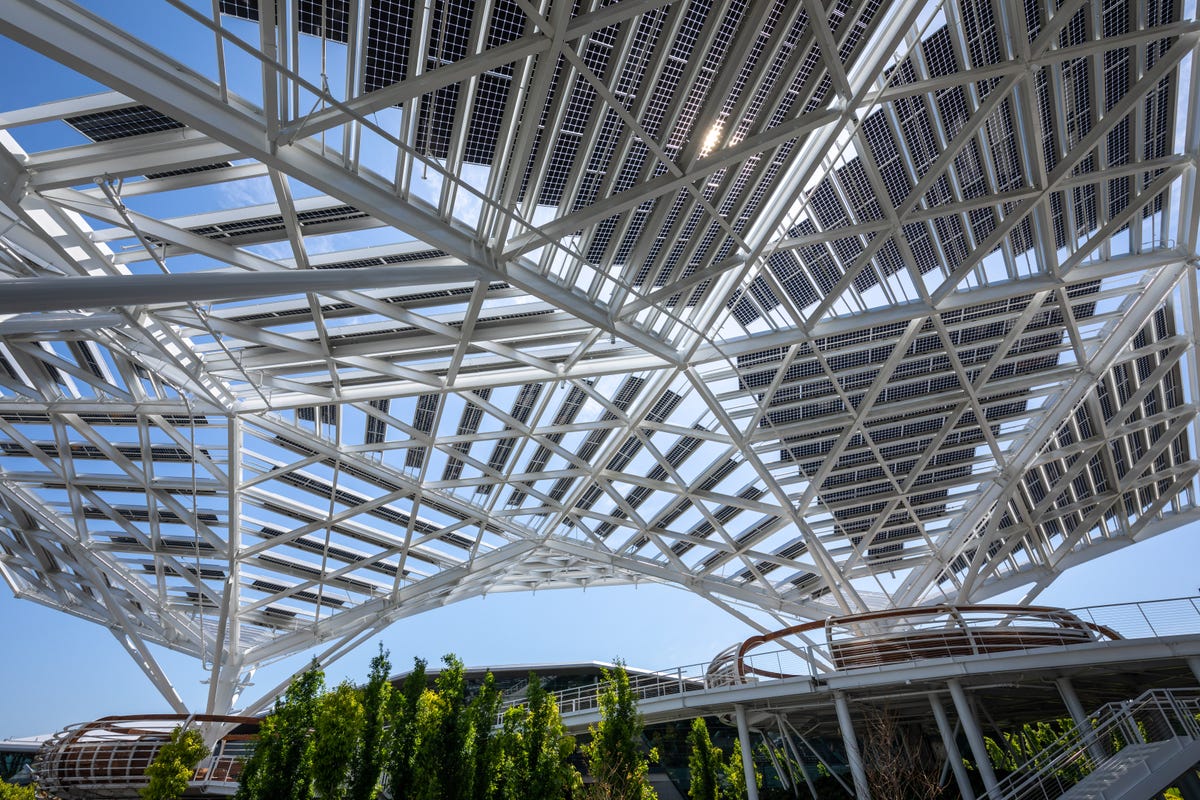
Nvidia Voyager Building's Trellis
Outside Nvidia's Voyager building is the "trellis," a canopy covered with solar panels. They're packed more thickly to the right to shade the front glass facade of the building. The panels turned out to be more susceptible to winds than expected, requiring stronger supports.

Nvidia Voyager Building Gardens
Four acres of garden separate Nvidia's Voyager building, right, from the earlier Endeavor to the left.
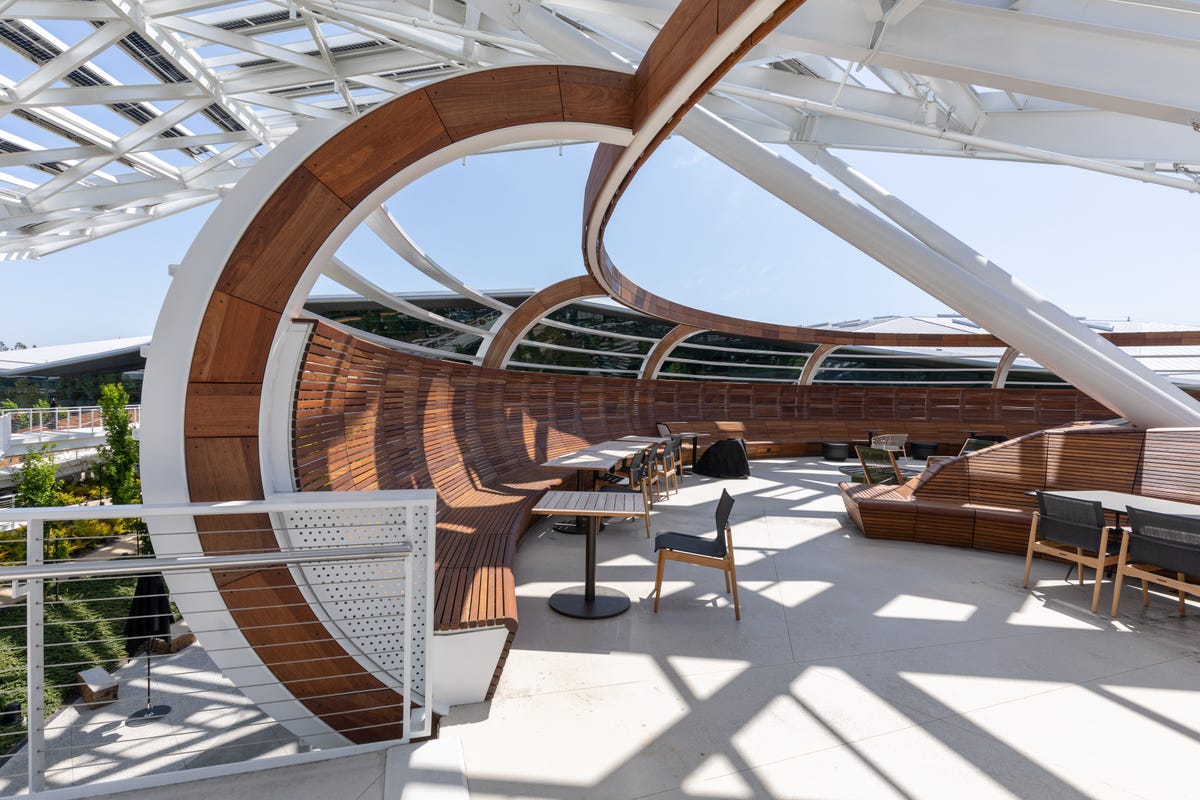
Voyager's "bird nests" are equipped with tables, benches and Wi-Fi.

A stairway leads up the front face of the "mountain" at the center of Nvidia's Voyager building in Santa Clara, California.
More Galleries
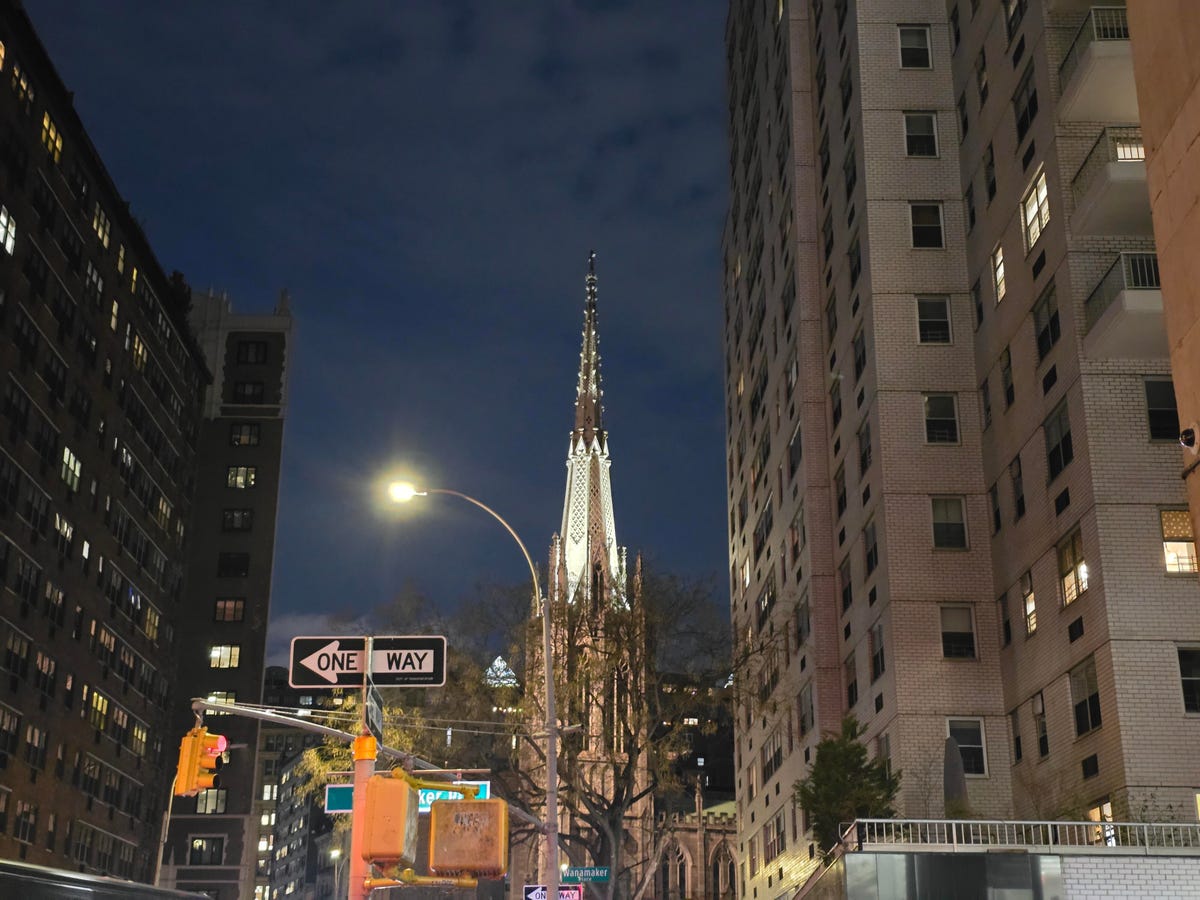
My Favorite Shots From the Galaxy S24 Ultra's Camera
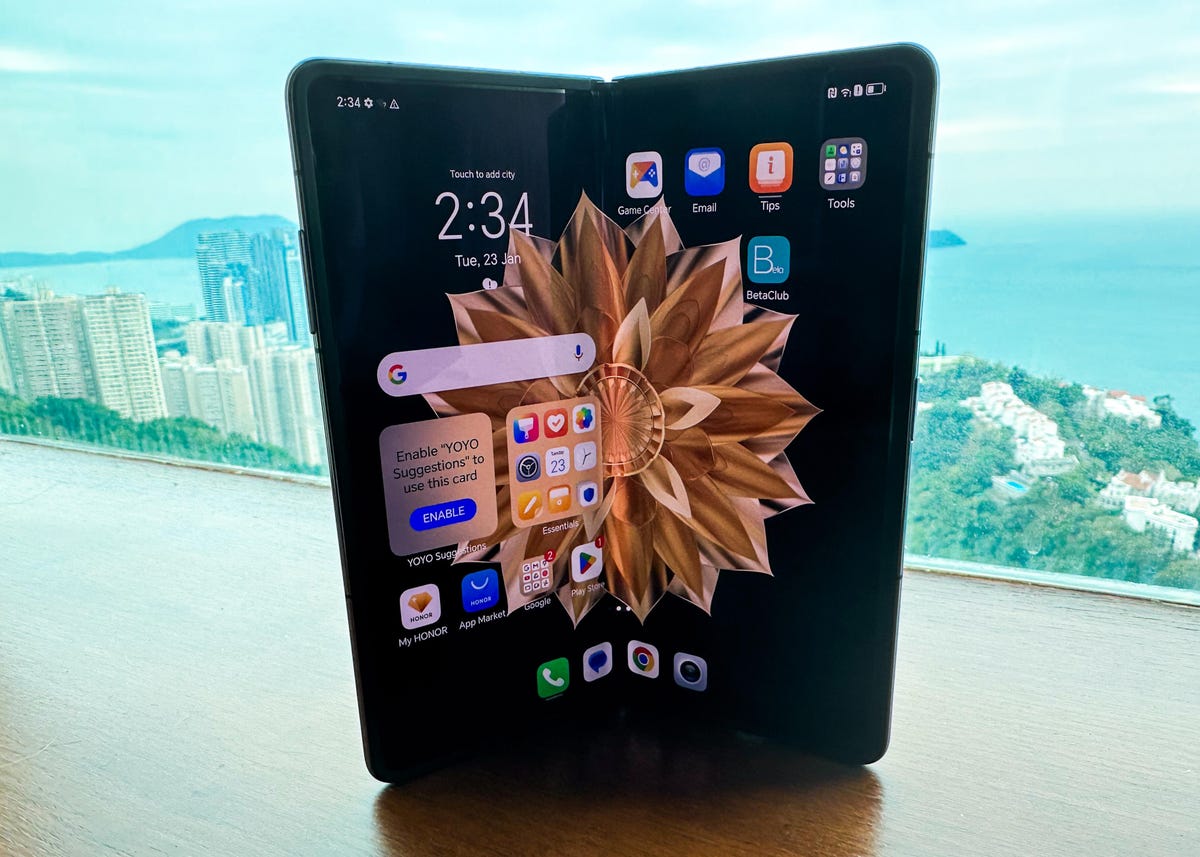
Honor's Magic V2 Foldable Is Lighter Than Samsung's Galaxy S24 Ultra
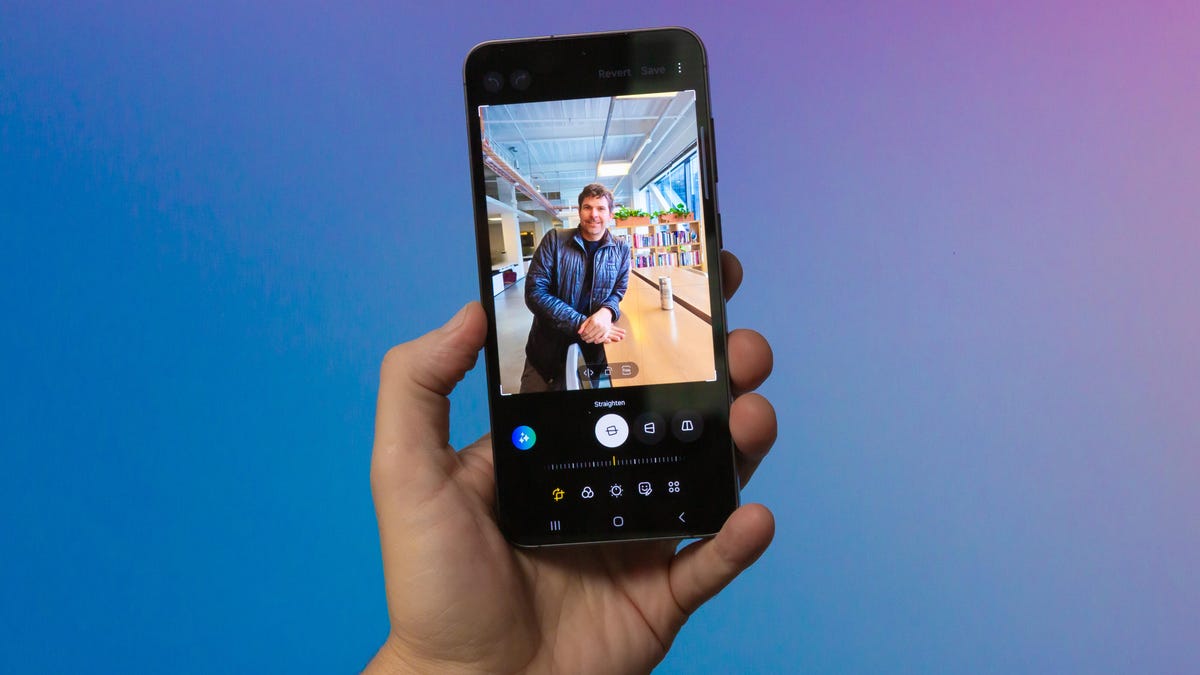
The Samsung Galaxy S24 and S24 Plus Looks Sweet in Aluminum
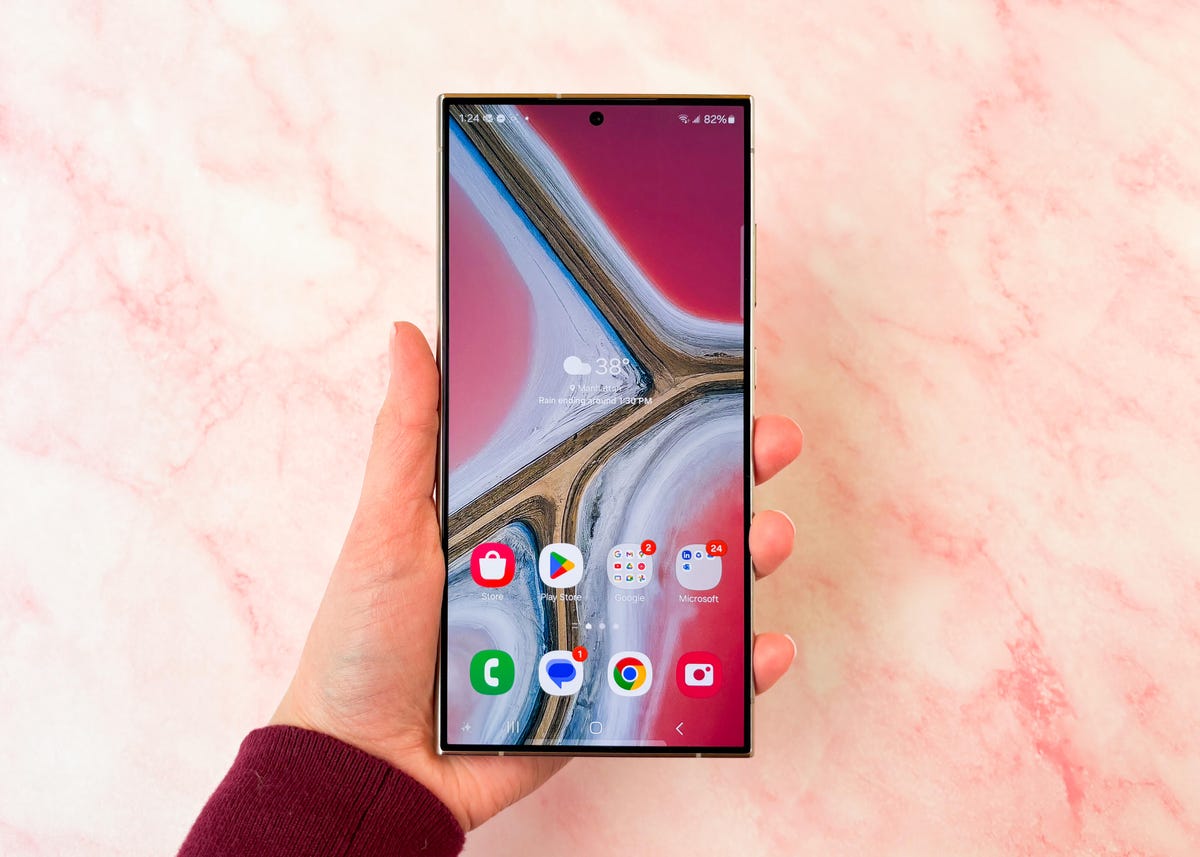
Samsung's Galaxy S24 Ultra Now Has a Titanium Design
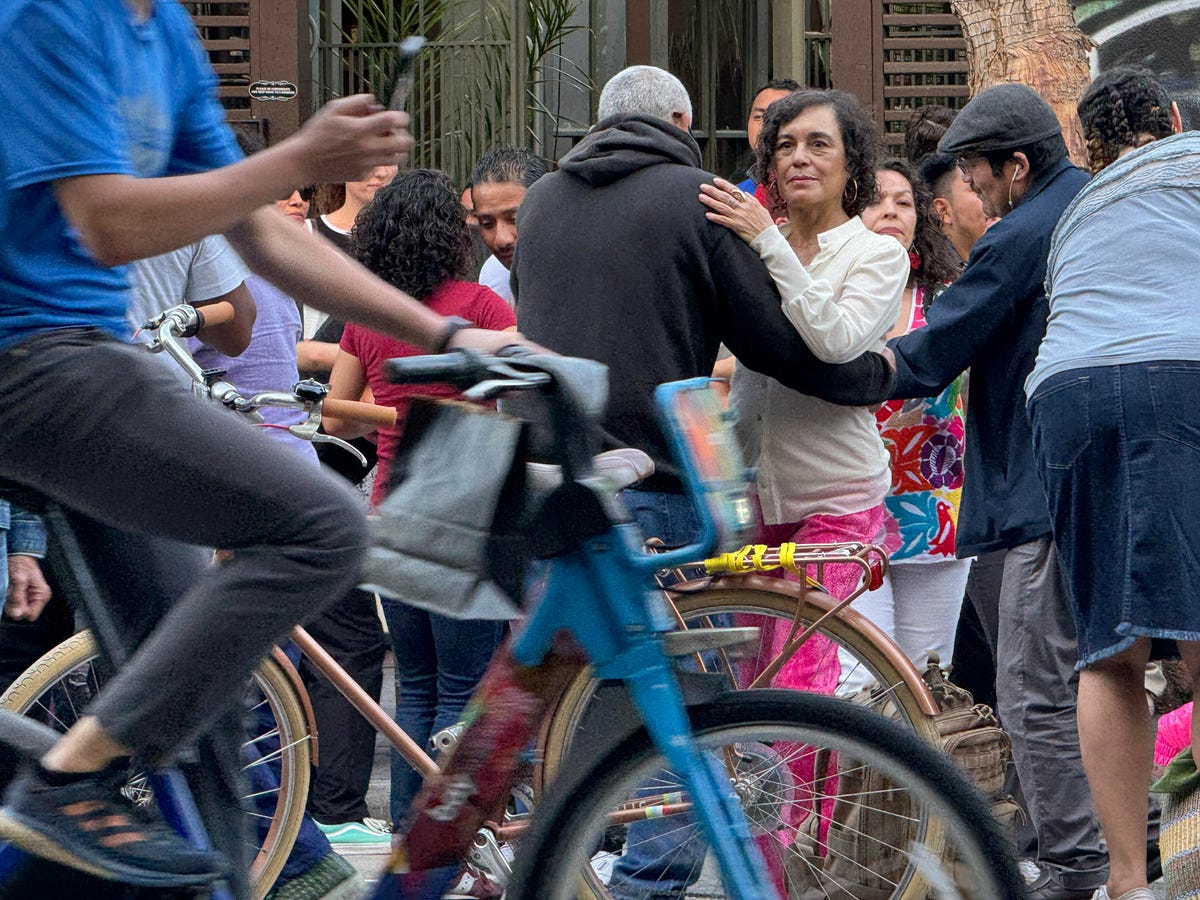
I Took 600+ Photos With the iPhone 15 Pro and Pro Max. Look at My Favorites

Do You Know About These 17 Hidden iOS 17 Features?
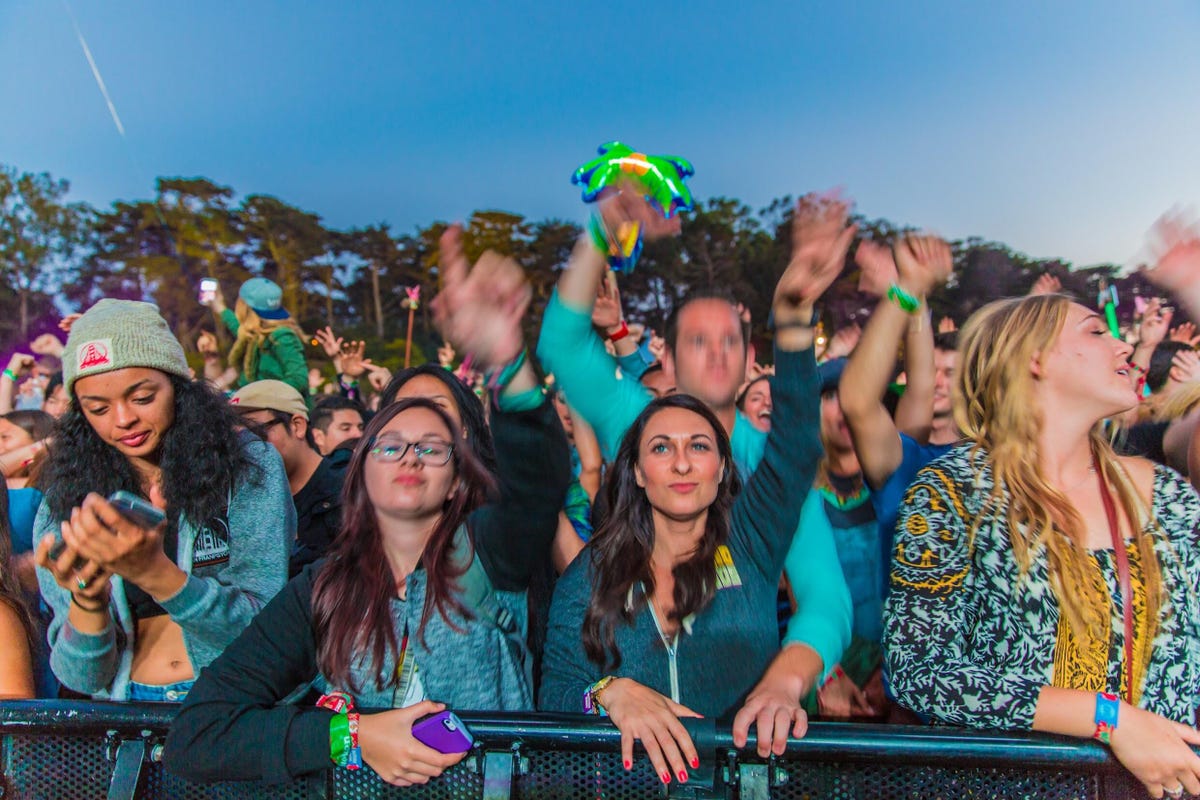
AI or Not AI: Can You Spot the Real Photos?
Voyager: An Open-Ended Embodied Agent with Large Language Models
We introduce Voyager, the first LLM-powered embodied lifelong learning agent in Minecraft that continuously explores the world, acquires diverse skills, and makes novel discoveries without human intervention. Voyager consists of three key components: 1) an automatic curriculum that maximizes exploration, 2) an ever-growing skill library of executable code for storing and retrieving complex behaviors, and 3) a new iterative prompting mechanism that incorporates environment feedback, execution errors, and self-verification for program improvement. Voyager interacts with GPT-4 via blackbox queries, which bypasses the need for model parameter fine-tuning. The skills developed by Voyager are temporally extended, interpretable, and compositional, which compounds the agent's abilities rapidly and alleviates catastrophic forgetting. Empirically, Voyager shows strong in-context lifelong learning capability and exhibits exceptional proficiency in playing Minecraft. It obtains 3.3x more unique items, travels 2.3x longer distances, and unlocks key tech tree milestones up to 15.3x faster than prior SOTA. Voyager is able to utilize the learned skill library in a new Minecraft world to solve novel tasks from scratch, while other techniques struggle to generalize.
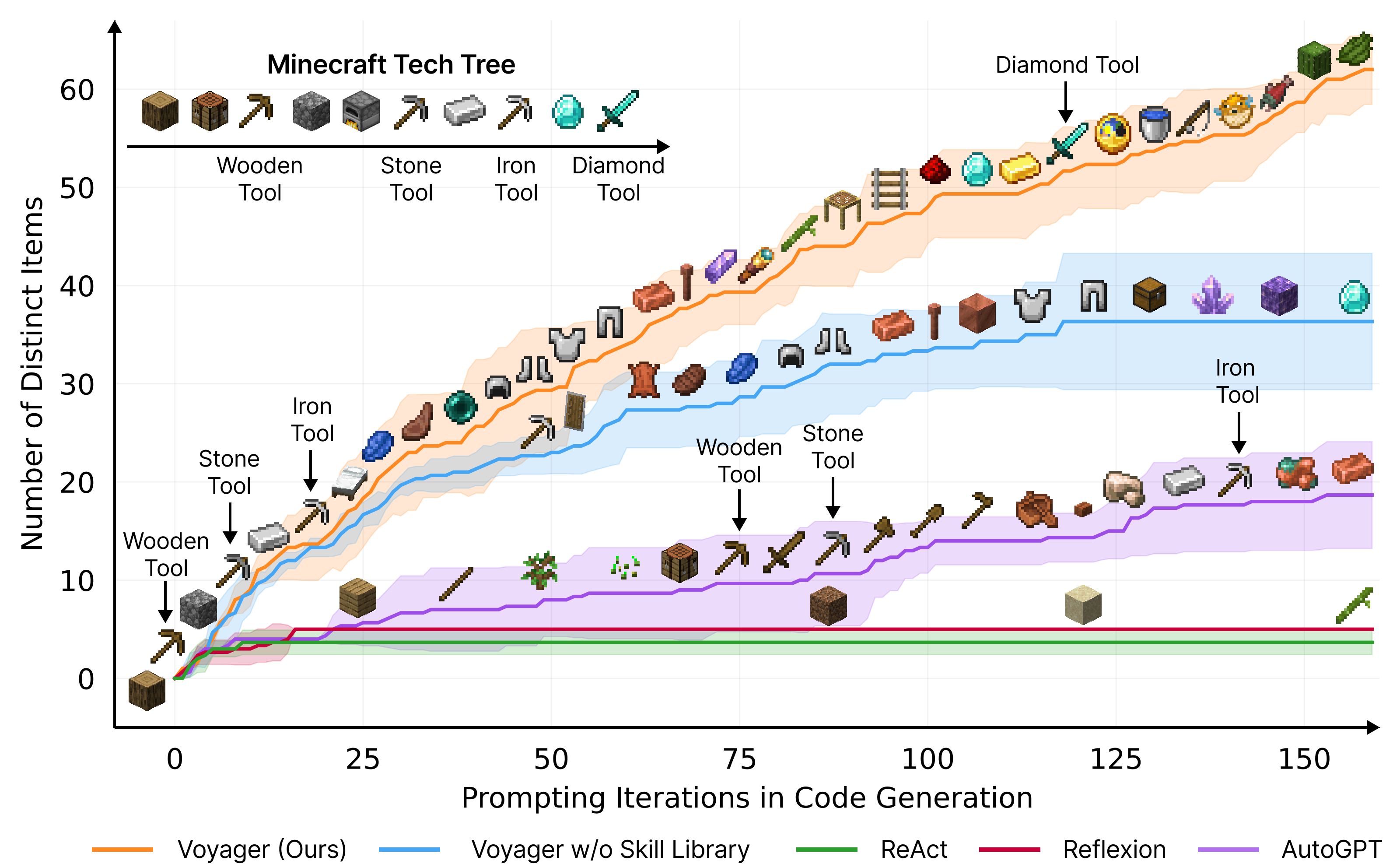
Introduction
Voyager components.
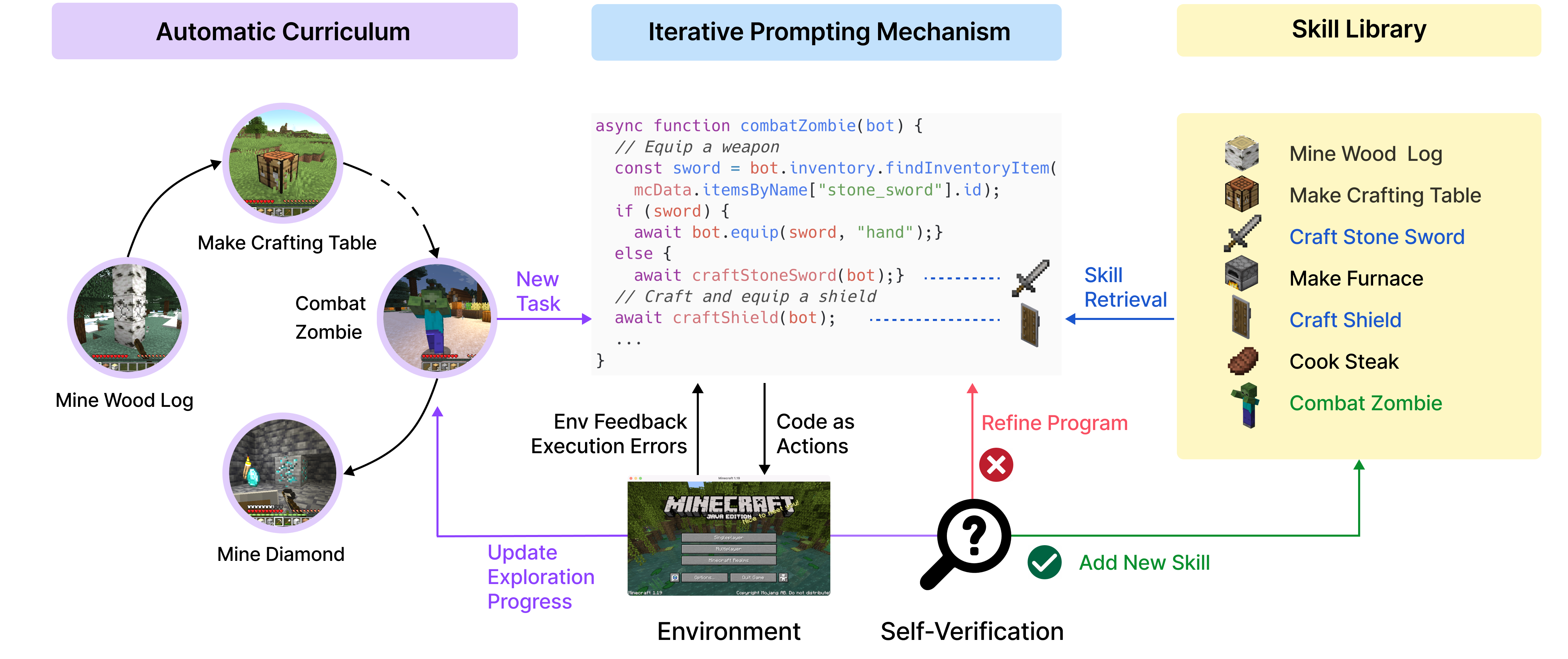
Automatic Curriculum
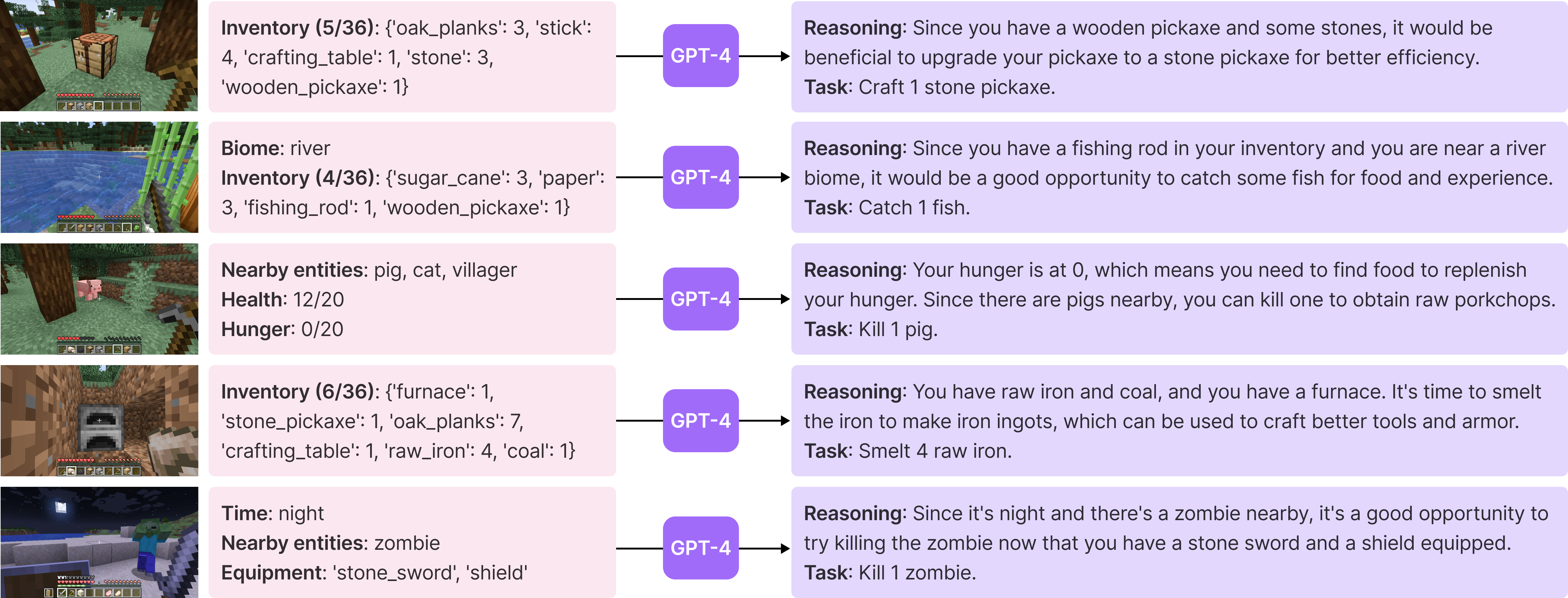
Skill Library
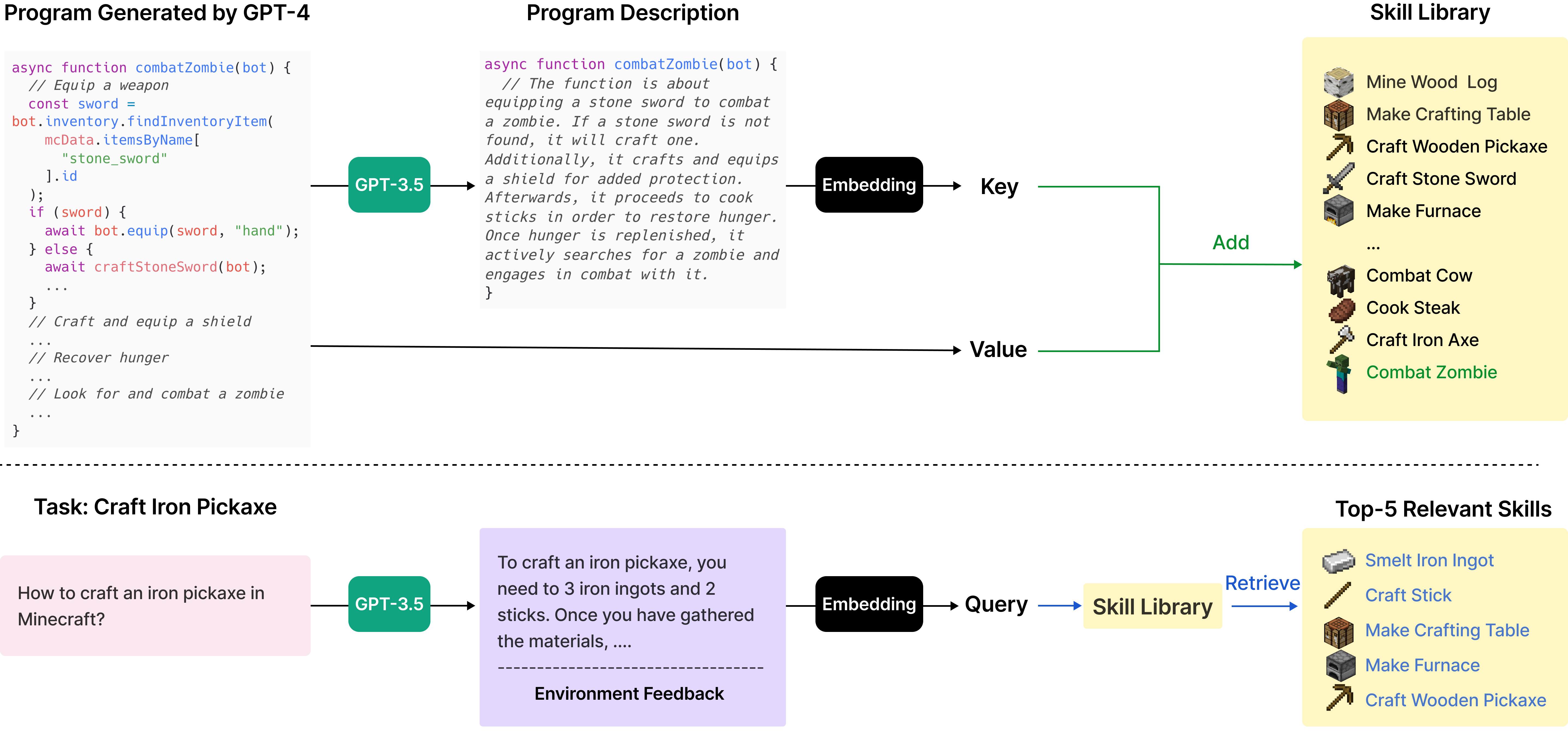
Iterative Prompting Mechanism
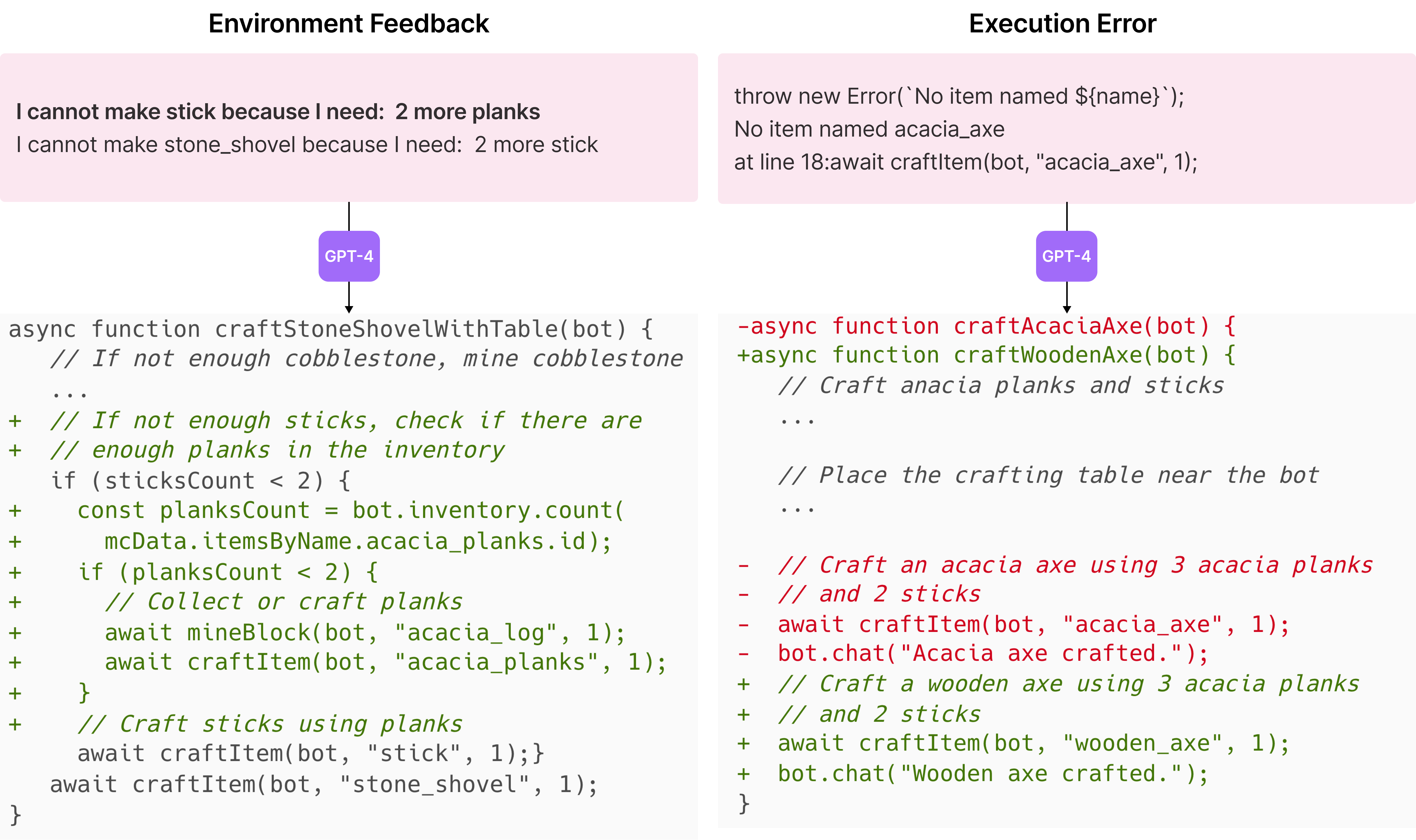
Experiments
We systematically evaluate Voyager and baselines on their exploration performance, tech tree mastery, map coverage, and zero-shot generalization capability to novel tasks in a new world.
Significantly Better Exploration
Tech tree mastery.

Extensive Map Traversal
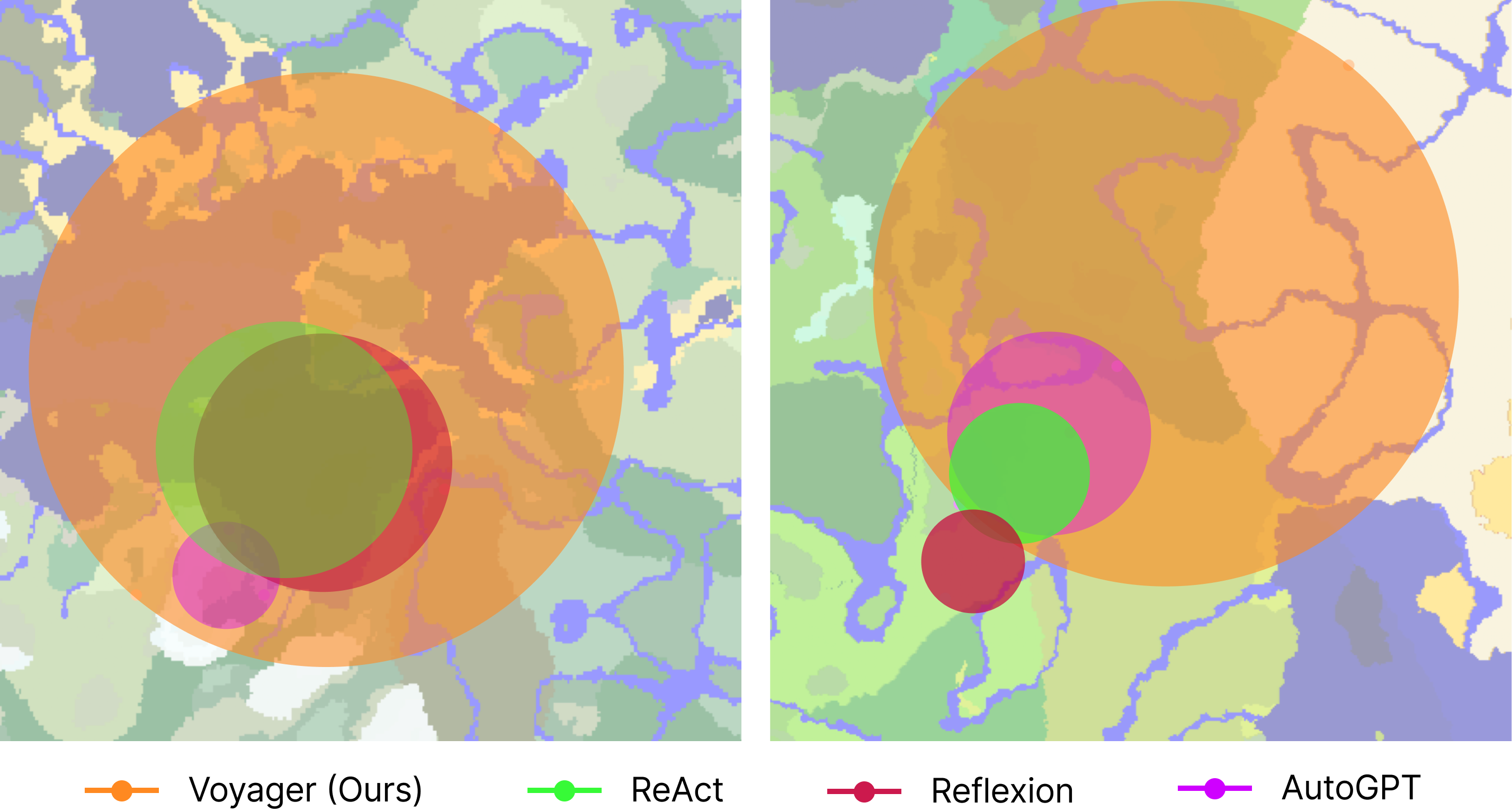
Efficient Zero-Shot Generalization to Unseen Tasks
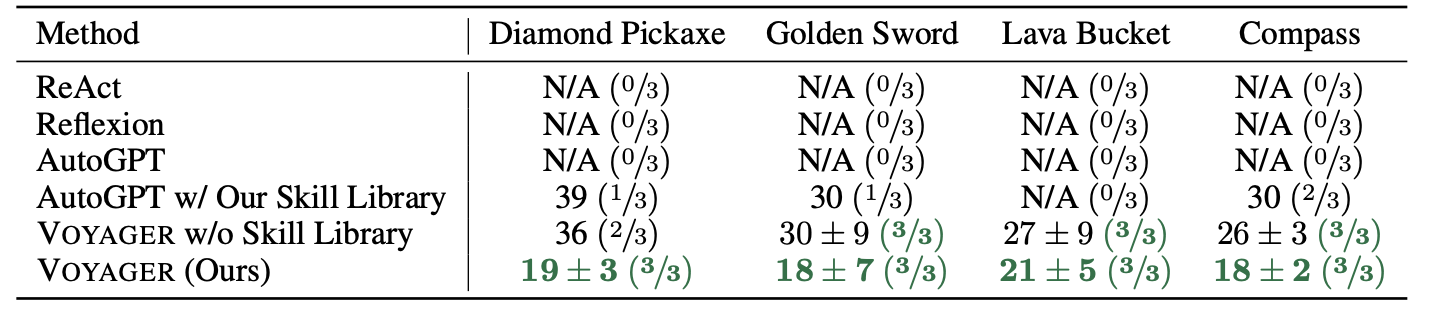
Ablation Studies
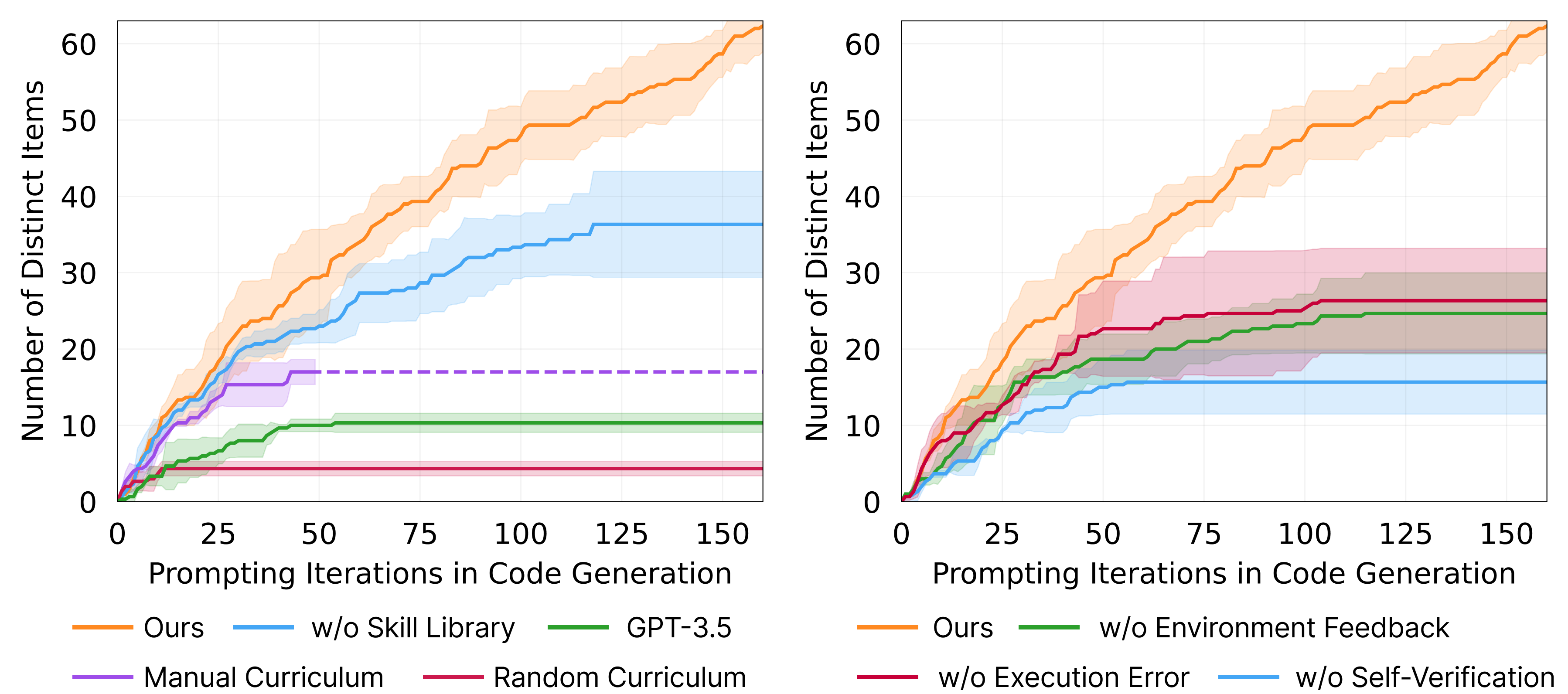
In this work, we introduce Voyager, the first LLM-powered embodied lifelong learning agent, which leverages GPT-4 to explore the world continuously, develop increasingly sophisticated skills, and make new discoveries consistently without human intervention. Voyager exhibits superior performance in discovering novel items, unlocking the Minecraft tech tree, traversing diverse terrains, and applying its learned skill library to unseen tasks in a newly instantiated world. Voyager serves as a starting point to develop powerful generalist agents without tuning the model parameters.
Media Coverage
"They Plugged GPT-4 Into Minecraft—and Unearthed New Potential for AI. The bot plays the video game by tapping the text generator to pick up new skills, suggesting that the tech behind ChatGPT could automate many workplace tasks." - Will Knight, WIRED "The Voyager project shows, however, that by pairing GPT-4’s abilities with agent software that stores sequences that work and remembers what does not, developers can achieve stunning results." - John Koetsier, Forbes "Voyager, the GTP-4 bot that plays Minecraft autonomously and better than anyone else" - Ruetir "This AI used GPT-4 to become an expert Minecraft player" - Devin Coldewey, TechCrunch Coverage Index: [Atmarkit] [Career Engine] [Crast.net] [Daily Top Feeds] [Entrepreneur en Espanol] [Finance Jxyuging] [Forbes] [Forbes Argentina] [Gaming Deputy] [Gearrice] [Haberik] [Head Topics] [InfoQ] [ITmedia News] [Mark Tech Post] [Medium] [MSN] [Note] [Noticias de Hoy] [Ruetir] [Stock HK] [Tech Tribune France] [TechCrunch] [TechBeezer] [Toutiao] [US Times Post] [VN Explorer] [WIRED] [Zaker]
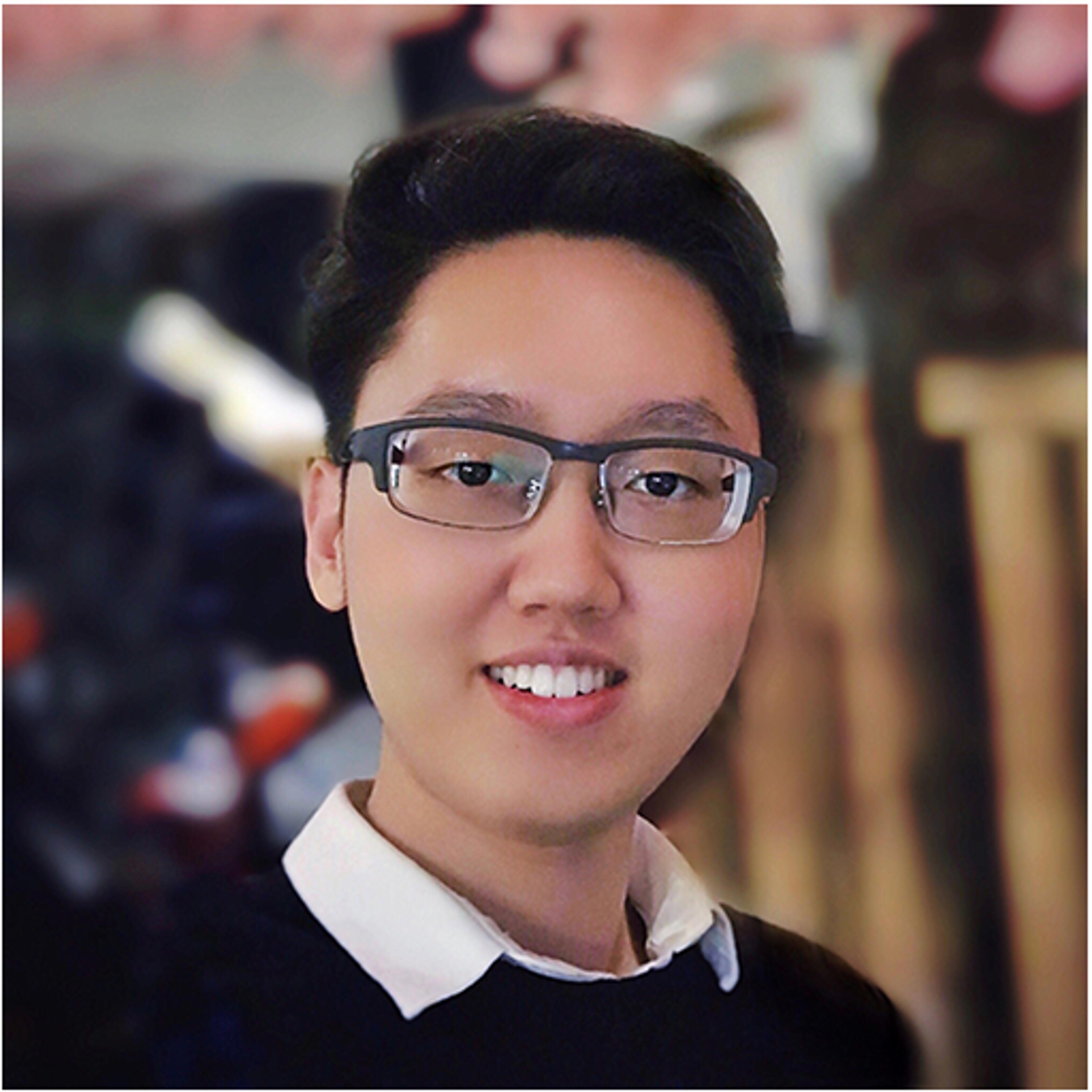
Nvidia Shares First Look Inside Massive New 'Voyager' Building
Voyager joins Endeavor to form Nvidia's Santa Clara HQ.
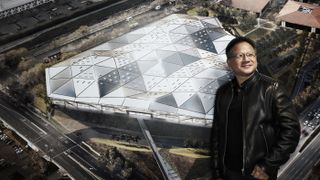
Nvidia recently opened up its 750,000 sq ft Voyager building. Consumer electronics news site CNet enjoyed access to the "colossal new building" which forms a major part of Nvidia's Santa Clara HQ.
With the completion of Voyager, joining the similarly impressive Endeavor, the office, meetings, and conference space have effectively been doubled. You may have twigged - yes, these two massive structures are named after Star Trek starships.
There was talk of Voyager and Endeavor being joined by a footbridge, wittily named the SLI Bridge, but that isn't mentioned in CNet's description. Between the two massifs, we see a four acre garden area with a trellis structure above dotted with solar panels. There are gaps in the trellis to provide light and shade for a plentiful array of benches, tables, and other social spaces beneath. In some images, you will see raised circular meeting 'nests' too.
Venturing into Voyager, a visitor can see the 'base camp' reception area. This area sits at the foot of a 'mountain' structure which has several tiers, interspersed with gardens, seating areas, cafes, offices, and so on. The mountain doesn't quite reach the pinnacle of the roof giving an impression of a big and airy open space – though you are indoors. The roof is interspersed with triangular natural light cutouts, which will be appreciated by the vegetation and humans alike.
The move away from boxy cubicle structures and work life permeates the whole building. Apparently, Nvidia CEO Jensen Huang wanted every employee working in Voyager to have a view, and work among "living walls, natural light, and towering windows." There are abundant outside and shared gathering spaces for working out of any allocated personal office space too.
There is an interesting reason for the impressive new Voyager building existing, other than being an HQ to impress in the manner and scale of rivals like Google , Oracle, and Apple. The space should entice employees who have become ingrained in WFH back to the office some more, and help Nvidia gain new talent - and keep them on board. However, the CNet report didn't mention one of the biggest draws of a welcoming workplace – the quality of the cafeteria.
For more pictures of Voyager, you can check out the source article. Nvidia has a gallery of renders and photos of both Voyager and Endeavor, too.
Stay On the Cutting Edge: Get the Tom's Hardware Newsletter
Get Tom's Hardware's best news and in-depth reviews, straight to your inbox.
Mark Tyson is a news editor at Tom's Hardware. He enjoys covering the full breadth of PC tech; from business and semiconductor design to products approaching the edge of reason.
Flexible Fiber LEDs made with perovskite quantum wires should enable advanced wearable displays and other technologies
The Royal Mint, official maker of British coins, begins mining gold from motherboards — a 40,000 square feet facility yields half a ton of gold per year
AMD RX 7600 XT vs RX 6750 XT GPU faceoff: Two $300 AMD cards duke it out for mainstream supremacy
- giorgiog Great, another "open" workspace - when will executives 'get' that engineers need a quiet, distraction free place (i.e. private offices) to work on hard problems for extended periods of time? It's like none of these people understand what 'flow' is. Reply
- View All 1 Comment
Most Popular
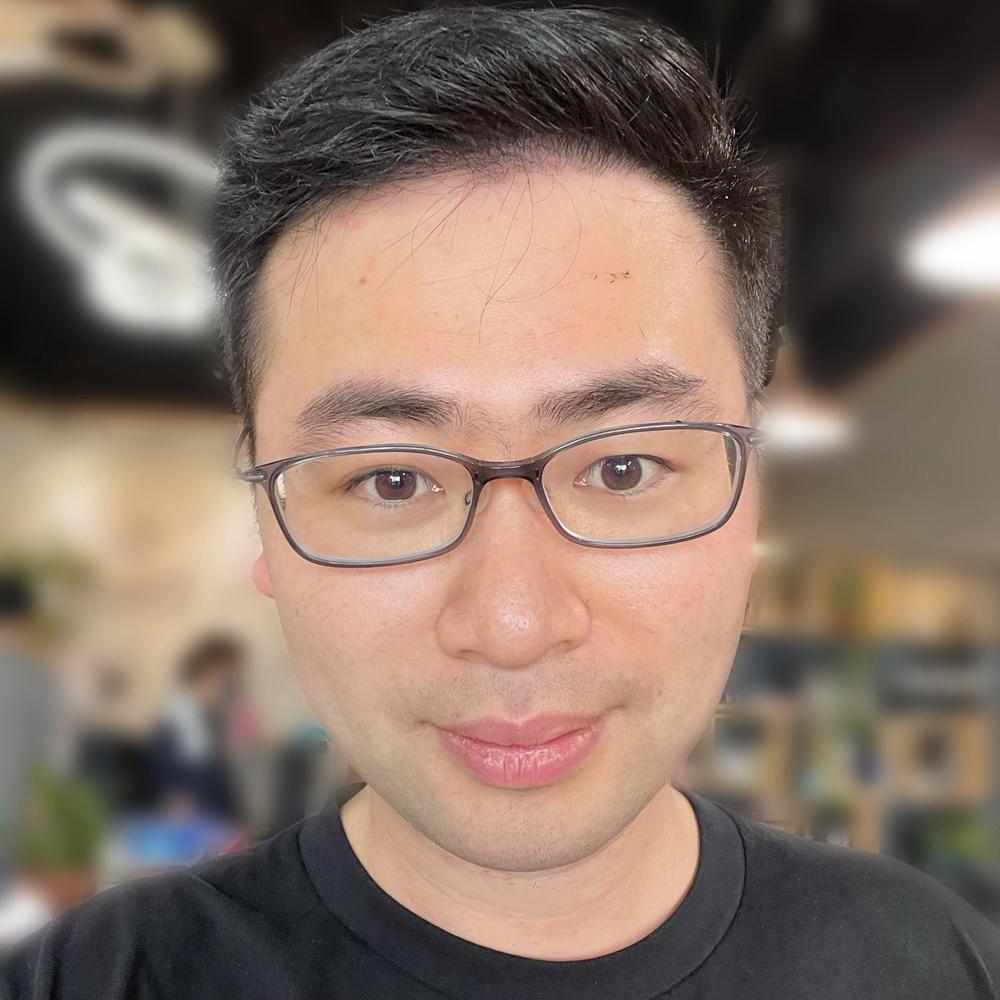
Linxi "Jim" Fan
Senior research scientist lead of ai agents, follow @drjimfan, hello there.
I am a Senior Research Scientist at NVIDIA and Lead of AI Agents Initiative. My mission is to build generally capable agents across physical worlds (robotics) and virtual worlds (games, simulation) . I share insights about AI research & industry extensively on Twitter/X and LinkedIn . Welcome to follow me!
My research explores the bleeding edge of multimodal foundation models, reinforcement learning, computer vision, and large-scale systems. I obtained my Ph.D. degree at Stanford Vision Lab , advised by Prof. Fei-Fei Li . Previously, I interned at OpenAI (w/ Ilya Sutskever and Andrej Karpathy), Baidu AI Labs (w/ Andrew Ng and Dario Amodei), and MILA (w/ Yoshua Bengio). I graduated as the Valedictorian of Class 2016 and received the Illig Medal at Columbia University.
I spearheaded Voyager (the first AI agent that plays Minecraft proficiently and bootstraps its capabilities continuously), MineDojo (open-ended agent learning by watching 100,000s of Minecraft YouTube videos), Eureka (a 5-finger robot hand doing extremely dexterous tasks like pen spinning), and VIMA (one of the earliest multimodal foundation models for robot manipulation). MineDojo won the Outstanding Paper Award at NeurIPS 2022. My works have been widely featured in news media, such as New York Times, Forbes, MIT Technology Review, TechCrunch, The WIRED, VentureBeat, etc.
Fun fact: I was OpenAI’s very first intern in 2016 . During that summer, I worked on World of Bits , an agent that perceives the web browser in pixels and outputs keyboard/mouse control. It was way before LLM became a thing at OpenAI. Good old times!

Research Highlights
Media Coverage
Publications.
Visit my Google Scholar page for a comprehensive listing!
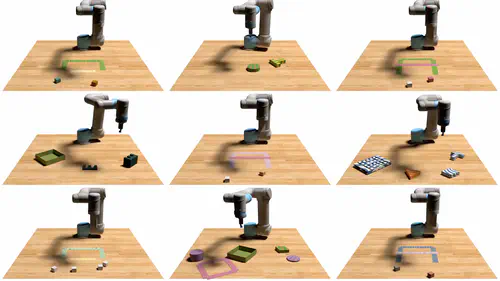
- Conducting bleeding edge research on foundation models for general-purpose autonomous agents .
- Leading the MineDojo effort for open-ended agent learning in Minecraft.
- Mentoring interns on diverse research topics.
- Collaborating with universities: Stanford, Berkeley, Caltech, MIT, UW, etc.
- Proposed SECANT , a state-of-the-art policy learning algorithm for zero-shot generalization of visual agents to novel environments.
- Paper published at ICML 2021 .
- Created SURREAL , an open-source, full-stack, and high-performance distributed reinforcement learning (RL) framework for large-scale robot learning.
- Paper published at CoRL 2018 . Best Presentation Award finalist.
- Doctoral advisor: Prof. Fei-Fei Li .
- Ph.D. Thesis “ Training and Deploying Visual Agents at Scale ”.
- Co-designed World of Bits , an open-domain platform for teaching AI to use the web browser. World of Bits was part of the OpenAI Universe initiative.
- Paper published at ICML 2017 .
- Systematically analyzed and proposed novel variants of the Ladder Network, a strong semi-supervised deep learning technique.
- Mentored by Turing Award Laureate Yoshua Bengio .
- Paper published at ICML 2016 .
- Co-developed DeepSpeech 2 , a large-scale end-to-end system that achieved world-class performance on English and Chinese speech recognition.
- Mentored by Dario Amodei , Adam Coates , and Andrew Ng .
- DeepSpeech and derivative works have been featured in various media: MIT Technology Review , TechCrunch , Forbes , NPR , VentureBeats , etc.
- Columbia NLP Group , advised by Prof. Michael Collins. Studied kernel methods for speech recognition. Paper published in Journal of Machine Learning Research .
- Columbia Vision Lab , advised by Prof. Shree Nayar. Implemented a computer vision system in Matlab to infer astrophysics parameters from galactic images.
- Columbia CRIS Lab , advised by Prof. Venkat Venkatasubramanian. Developed ML and NLP techniques to automate ontology curation for pharmaceutical engineering. Paper published in Computers & Chemical Engineering .
- [email protected]
- Welcome to DM me!

NVIDIA Voyager
- Civil design and survey for entitlement of NVIDIA’s newest addition to the 36-acre campus redevelopment project.
- Provided engineering and surveying to support construction of the first two project phases: Endeavor & Voyager.
- LEED Gold certified.
- Project awarded 2022 SVBJ Structures honoree for Interiors Project.
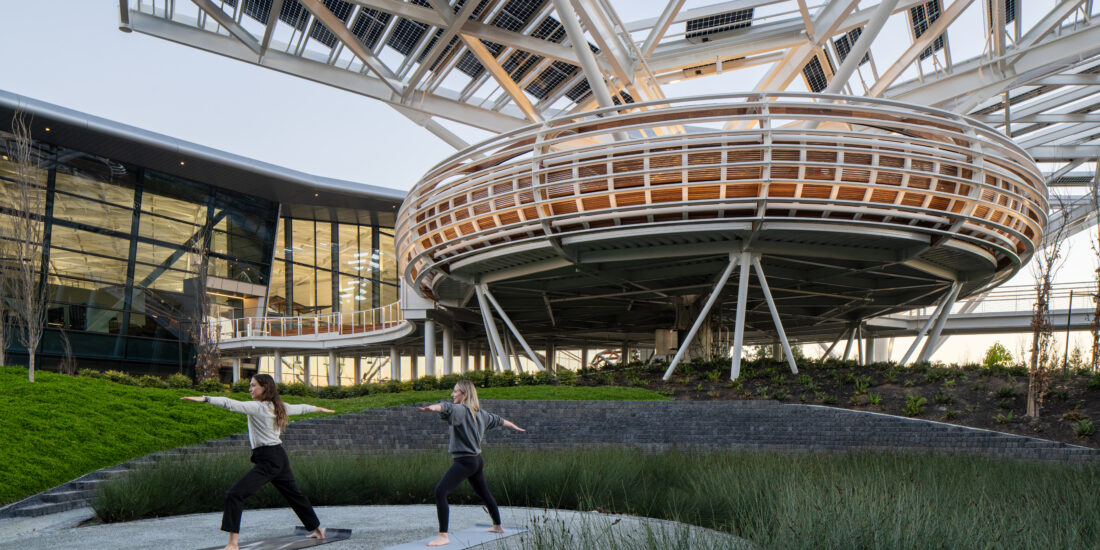
NVIDIA's newest addition to their headquarters, deemed Voyager, shares its wild, distinctly NVIDIA design inspired by the Pacific Northwest’s lush, mountainous terrain. The 750,000 sq ft building is designed to give employees an enjoyable and collaborative place to work. During the development of plans and during construction, Kier + Wright worked closely with NVIDIA, the general contractor, and the City of Santa Clara to ensure that public infrastructure was maintained while existing utilities were relocated, and new utilities were constructed. During the first phase of this corporate campus, Endeavor, K+W minimized the amount of rework that was done to coordinate and plan for the second phase, Voyager. The biggest challenge faced was modifications to the Endeavor project, specifically the locations of the biotreatment ponds that were relocated to the courtyard located between these two projects. K+W relocated four biotreatment ponds that were engineered for Endeavor, and seamlessly integrated them into the courtyard between these two campuses for an optimal approach that would work for both Endeavor and Voyager. These modifications were accomplished by having high levels of communication and coordination with NVIDIA, the City of Santa Clara, and the rest of the project team. The integration of biotreatment ponds, zero harvesting or infiltration throughout the project helped contribute to Voyager's LEED Gold certification. K+W also designed and implemented a retaining wall around the site to help minimize the project’s earthwork. This helped save the client money and raised the site to reduce the exported dirt. Expansion was designed with the vision and goal for future expansion while creating a cohesive campus that encourages collaboration inside and outside as well as employee health and wellness through sustainable features.
To learn more about this project, please view the video above.
NVIDIA Voyager Santa Clara, CA
- Corporate Campus
- Civil Engineering
- Site Development
- Utility Design
- Sustainability
- Street + Roadway
- Construction Administration
- Land Surveying
- Topographic Survey
- Construction Staking
- ALTA Land Title Survey
Silicon Valley Business Journal | Nvidia Voyager is the 2022 Structures honoree for Interiors Project
Tom’s Hardware | Nvidia Shares First Look Inside Massive New ‘Voyager’ Building
Venture Beat | Nvidia became a $1 trillion company thanks to AI. Look inside its lavish ‘Star Trek’-inspired HQ
Yahoo/Dornob | Nvidia’s Voyager HQ Seduces WFH Employees Back with a Dazzling Design
Related Projects
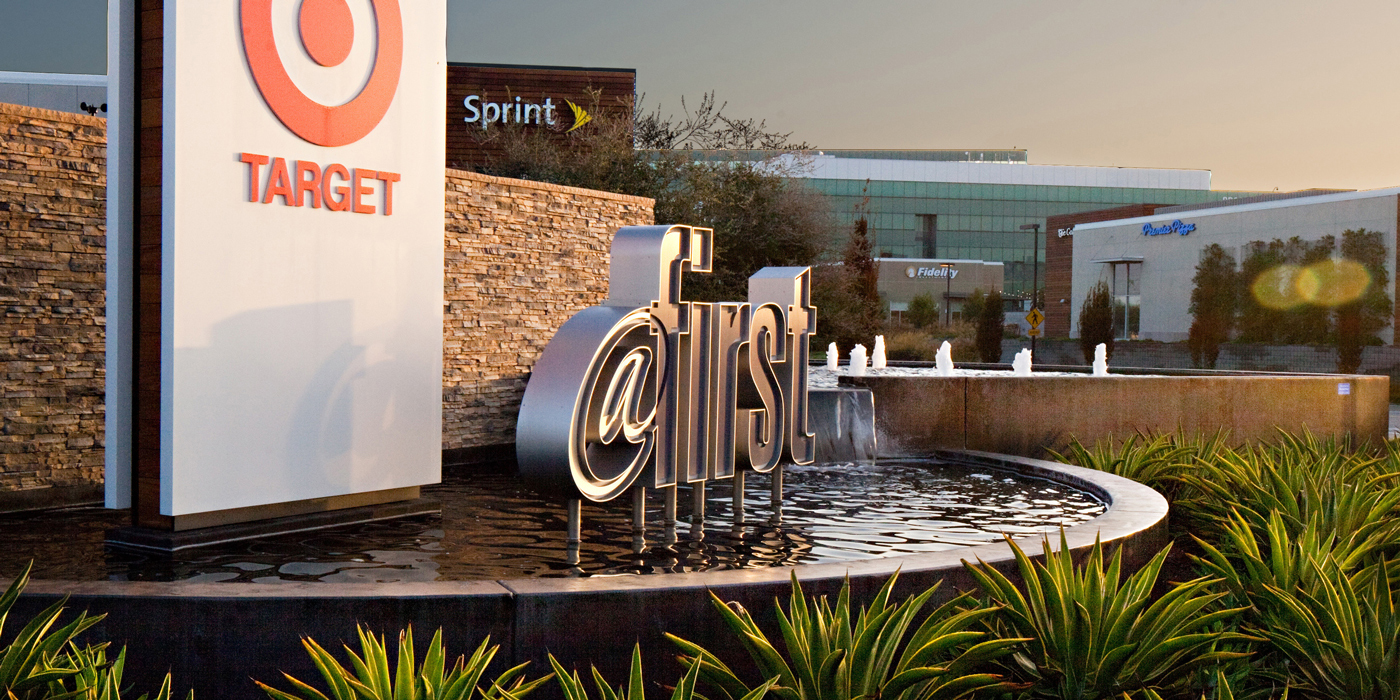
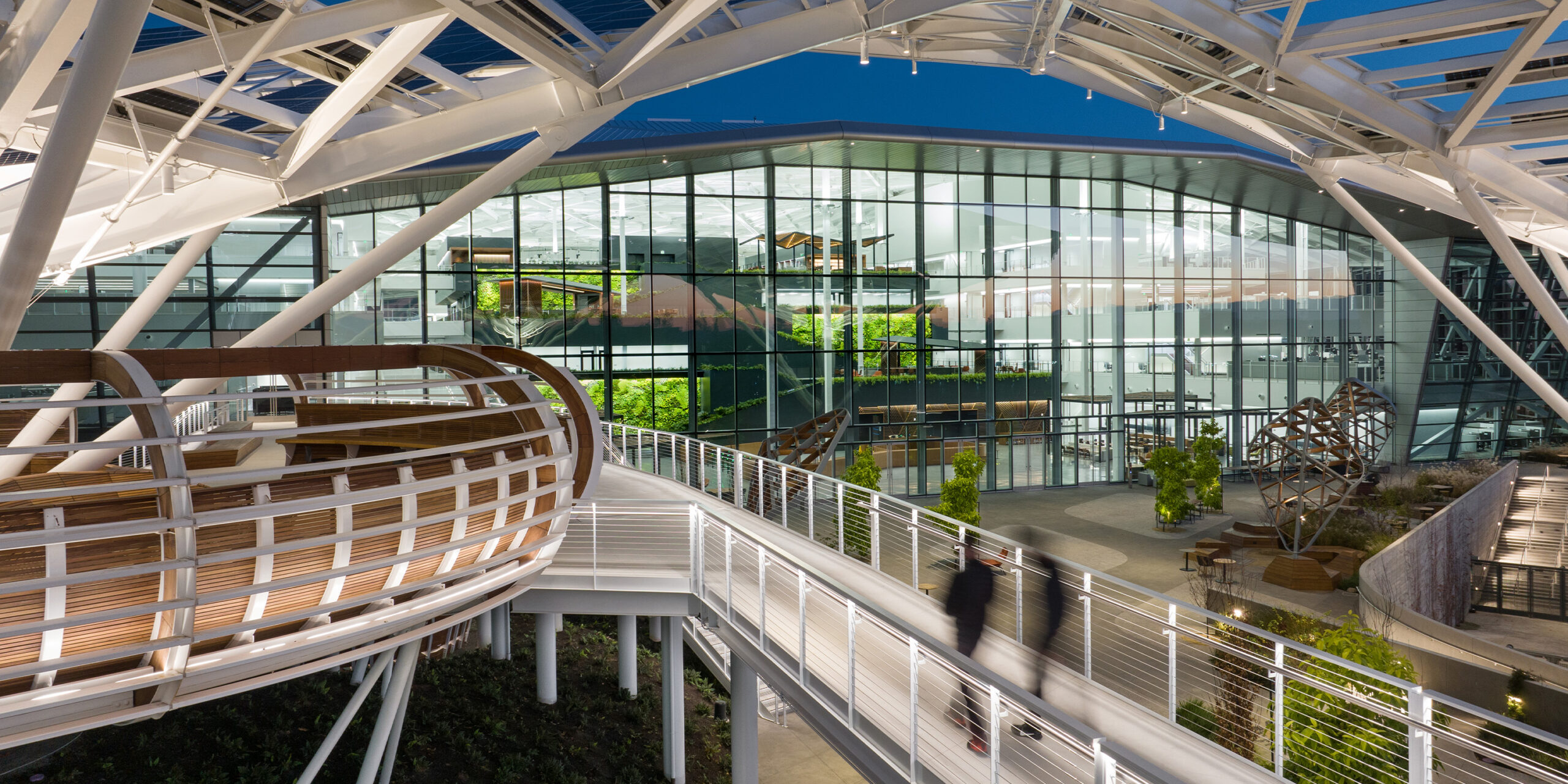
Nvidia – Voyager
Voyager is Nvidia’s latest 750,000 square foot addition to the 500,000 square foot Endeavor building, bringing the total headquarters to 1.25 million square feet. Possessing the same cosmic, distinct design as the first building, Voyager is linked to Endeavor by a treelined walkway shaded by a trellis of photovoltaic panels.
The 4-story, 68′ tall Voyager structure has a sloped glass curtain wall perimeter to allow abundant natural light, expansive views, and live plants to build a strong connection to nature, blurring the boundary between inside and outside. Enclos’ 170,000 square foot scope of work includes the glazed facets comprised of 14′ by 4′ glass cassette panels attached to horizontal secondary steel supports between the primary steel columns. The double-paned insulated glass units mitigate heat gain, glare, and shadow while permitting sufficient daylight to the interior for occupant comfort. At the main entrance, 7′ wide by 13′ tall vertical cassette units attach to architecturally exposed structural steel horizontal supports between primary columns. The main entrance wall also incorporates entry doors.
Enclos was responsible for the design-assist, testing and mock-up requirements, procurement, and construction of the façade.
Designed for LEED Gold certification, natural light is the primary lighting source during the day, supporting the 40% energy-saving goals.
project specs
Facade area:, general contractor:, facade consultant:, related projects.
One Tabor Center
Nortel Networks
Bloomberg Tower
Spectrum Terrace
Voyager : An Open-Ended Embodied Agent with Large Language Models
We introduce Voyager , the first LLM-powered embodied lifelong learning agent in Minecraft that continuously explores the world, acquires diverse skills, and makes novel discoveries without human intervention. Voyager consists of three key components: 1) an automatic curriculum that maximizes exploration, 2) an ever-growing skill library of executable code for storing and retrieving complex behaviors, and 3) a new iterative prompting mechanism that incorporates environment feedback, execution errors, and self-verification for program improvement. Voyager interacts with GPT-4 via blackbox queries, which bypasses the need for model parameter fine-tuning. The skills developed by Voyager are temporally extended, interpretable, and compositional, which compounds the agent’s abilities rapidly and alleviates catastrophic forgetting. Empirically, Voyager shows strong in-context lifelong learning capability and exhibits exceptional proficiency in playing Minecraft. It obtains 3.3 × 3.3\times more unique items, travels 2.3 × 2.3\times longer distances, and unlocks key tech tree milestones up to 15.3 × 15.3\times faster than prior SOTA. Voyager is able to utilize the learned skill library in a new Minecraft world to solve novel tasks from scratch, while other techniques struggle to generalize.
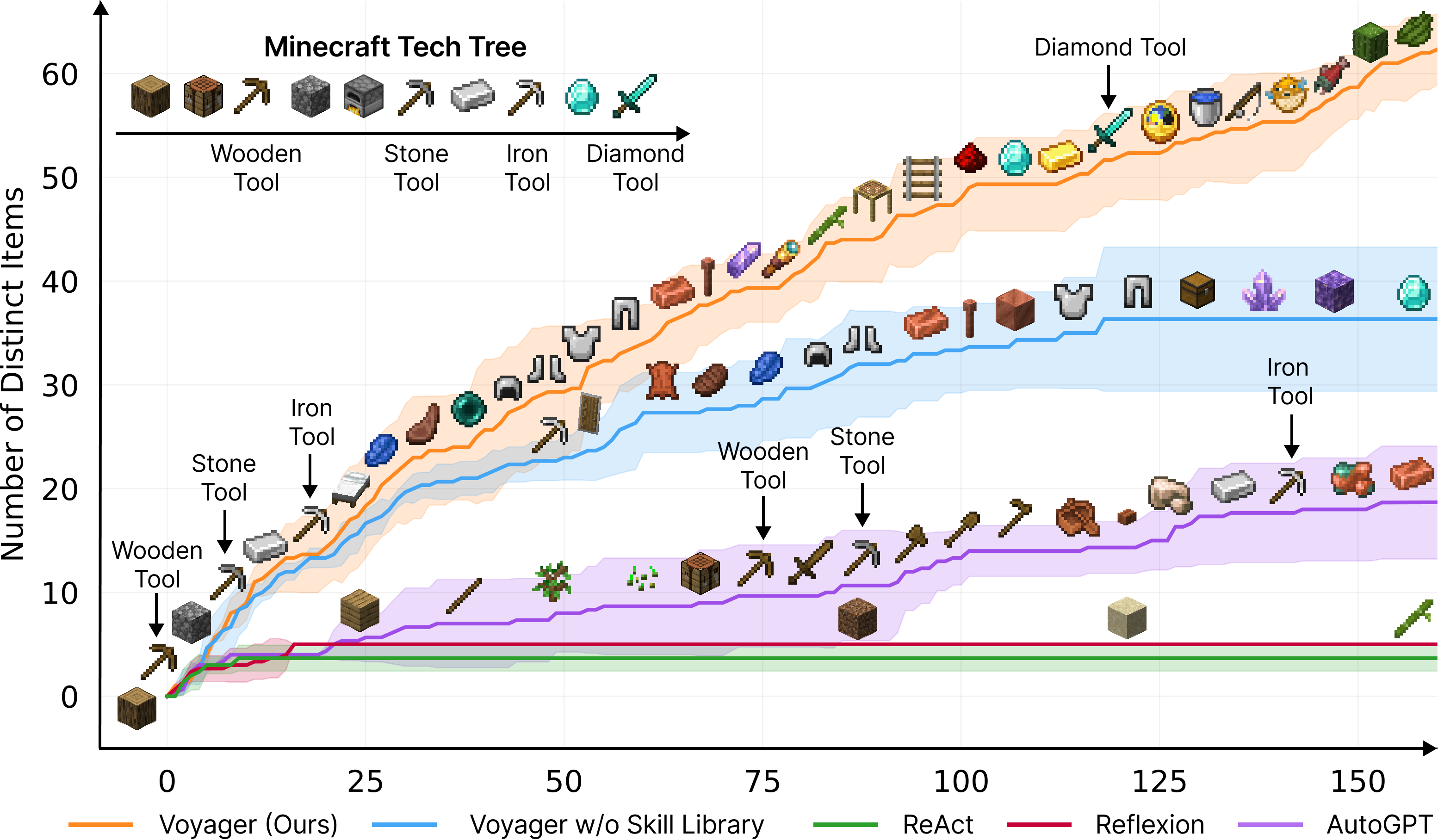
1 Introduction
Building generally capable embodied agents that continuously explore, plan, and develop new skills in open-ended worlds is a grand challenge for the AI community [ 1 , 2 , 3 , 4 , 5 ] . Classical approaches employ reinforcement learning (RL) [ 6 , 7 ] and imitation learning [ 8 , 9 , 10 ] that operate on primitive actions, which could be challenging for systematic exploration [ 11 , 12 , 13 , 14 , 15 ] , interpretability [ 16 , 17 , 18 ] , and generalization [ 19 , 20 , 21 ] . Recent advances in large language model (LLM) based agents harness the world knowledge encapsulated in pre-trained LLMs to generate consistent action plans or executable policies [ 16 , 22 , 19 ] . They are applied to embodied tasks like games and robotics [ 23 , 24 , 25 , 26 , 27 ] , as well as NLP tasks without embodiment [ 28 , 29 , 30 ] . However, these agents are not lifelong learners that can progressively acquire, update, accumulate, and transfer knowledge over extended time spans [ 31 , 32 ] .
Let us consider Minecraft as an example. Unlike most other games studied in AI [ 33 , 34 , 10 ] , Minecraft does not impose a predefined end goal or a fixed storyline but rather provides a unique playground with endless possibilities [ 23 ] . Minecraft requires players to explore vast, procedurally generated 3D terrains and unlock a tech tree using gathered resources. Human players typically start by learning the basics, such as mining wood and cooking food, before advancing to more complex tasks like combating monsters and crafting diamond tools. We argue that an effective lifelong learning agent should have similar capabilities as human players: (1) propose suitable tasks based on its current skill level and world state, e.g., learn to harvest sand and cactus before iron if it finds itself in a desert rather than a forest; (2) refine skills based on environmental feedback and commit mastered skills to memory for future reuse in similar situations (e.g. fighting zombies is similar to fighting spiders); (3) continually explore the world and seek out new tasks in a self-driven manner.
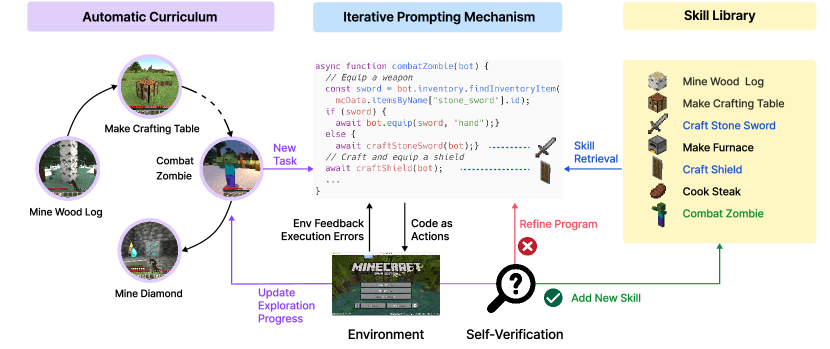
Towards these goals, we introduce Voyager , the first LLM-powered embodied lifelong learning agent to drive exploration, master a wide range of skills, and make new discoveries continually without human intervention in Minecraft. Voyager is made possible through three key modules (Fig. 2 ): 1) an automatic curriculum that maximizes exploration; 2) a skill library for storing and retrieving complex behaviors; and 3) a new iterative prompting mechanism that generates executable code for embodied control. We opt to use code as the action space instead of low-level motor commands because programs can naturally represent temporally extended and compositional actions [ 16 , 22 ] , which are essential for many long-horizon tasks in Minecraft. Voyager interacts with a blackbox LLM (GPT-4 [ 35 ] ) through prompting and in-context learning [ 36 , 37 , 38 ] . Our approach bypasses the need for model parameter access and explicit gradient-based training or finetuning.
More specifically, Voyager attempts to solve progressively harder tasks proposed by the automatic curriculum , which takes into account the exploration progress and the agent’s state. The curriculum is generated by GPT-4 based on the overarching goal of “discovering as many diverse things as possible”. This approach can be perceived as an in-context form of novelty search [ 39 , 40 ] . Voyager incrementally builds a skill library by storing the action programs that help solve a task successfully. Each program is indexed by the embedding of its description, which can be retrieved in similar situations in the future. Complex skills can be synthesized by composing simpler programs, which compounds Voyager ’s capabilities rapidly over time and alleviates catastrophic forgetting in other continual learning methods [ 31 , 32 ] .
However, LLMs struggle to produce the correct action code consistently in one shot [ 41 ] . To address this challenge, we propose an iterative prompting mechanism that: (1) executes the generated program to obtain observations from the Minecraft simulation (such as inventory listing and nearby creatures) and error trace from the code interpreter (if any); (2) incorporates the feedback into GPT-4’s prompt for another round of code refinement; and (3) repeats the process until a self-verification module confirms the task completion, at which point we commit the program to the skill library (e.g., craftStoneShovel() and combatZombieWithSword() ) and query the automatic curriculum for the next milestone (Fig. 2 ).
Empirically, Voyager demonstrates strong in-context lifelong learning capabilities. It can construct an ever-growing skill library of action programs that are reusable, interpretable, and generalizable to novel tasks. We evaluate Voyager systematically against other LLM-based agent techniques (e.g., ReAct [ 29 ] , Reflexion [ 30 ] , AutoGPT [ 28 ] ) in MineDojo [ 23 ] , an open-source Minecraft AI framework. Voyager outperforms prior SOTA by obtaining 3.3 × 3.3\times more unique items, unlocking key tech tree milestones up to 15.3 × 15.3\times faster, and traversing 2.3 × 2.3\times longer distances. We further demonstrate that Voyager is able to utilize the learned skill library in a new Minecraft world to solve novel tasks from scratch, while other methods struggle to generalize.
Voyager consists of three novel components: (1) an automatic curriculum (Sec. 2.1 ) that suggests objectives for open-ended exploration, (2) a skill library (Sec. 2.2 ) for developing increasingly complex behaviors, and (3) an iterative prompting mechanism (Sec. 2.3 ) that generates executable code for embodied control. Full prompts are presented in Appendix, Sec. A .
2.1 Automatic Curriculum

Embodied agents encounter a variety of objectives with different complexity levels in open-ended environments. An automatic curriculum offers numerous benefits for open-ended exploration, ensuring a challenging but manageable learning process, fostering curiosity-driven intrinsic motivation for agents to learn and explore, and encouraging the development of general and flexible problem-solving strategies [ 42 , 43 , 44 ] . Our automatic curriculum capitalizes on the internet-scale knowledge contained within GPT-4 by prompting it to provide a steady stream of new tasks or challenges. The curriculum unfolds in a bottom-up fashion, allowing for considerable adaptability and responsiveness to the exploration progress and the agent’s current state (Fig. 3 ). As Voyager progresses to harder self-driven goals, it naturally learns a variety of skills, such as “mining a diamond”.
The input prompt to GPT-4 consists of several components:
Directives encouraging diverse behaviors and imposing constraints , such as “ My ultimate goal is to discover as many diverse things as possible ... The next task should not be too hard since I may not have the necessary resources or have learned enough skills to complete it yet. ”;
The agent’s current state , including inventory, equipment, nearby blocks and entities, biome, time, health and hunger bars, and position;
Previously completed and failed tasks , reflecting the agent’s current exploration progress and capabilities frontier;
Additional context : We also leverage GPT-3.5 to self-ask questions based on the agent’s current state and exploration progress and self-answer questions. We opt to use GPT-3.5 instead of GPT-4 for standard NLP tasks due to budgetary considerations.
2.2 Skill Library
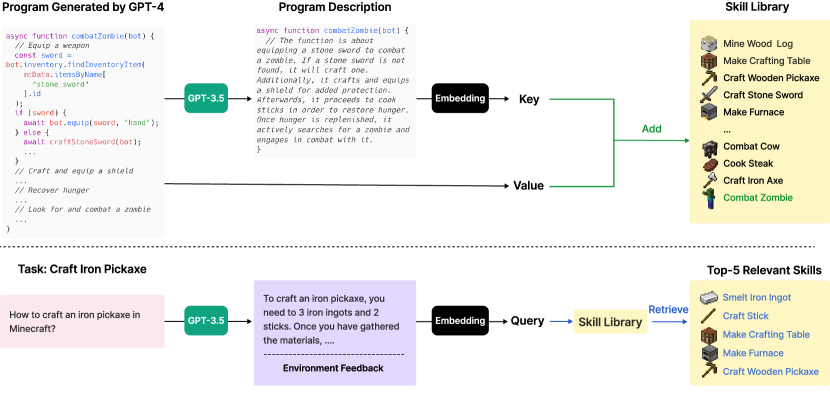
With the automatic curriculum consistently proposing increasingly complex tasks, it is essential to have a skill library that serves as a basis for learning and evolution. Inspired by the generality, interpretability, and universality of programs [ 45 ] , we represent each skill with executable code that scaffolds temporally extended actions for completing a specific task proposed by the automatic curriculum.
The input prompt to GPT-4 consists of the following components:
Guidelines for code generation , such as “ Your function will be reused for building more complex functions. Therefore, you should make it generic and reusable. ”;
Control primitive APIs, and relevant skills retrieved from the skill library, which are crucial for in-context learning [ 36 , 37 , 38 ] to work well;
The generated code from the last round, environment feedback, execution errors, and critique , based on which GPT-4 can self-improve (Sec. 2.3 );
Chain-of-thought prompting [ 46 ] to do reasoning before code generation.
We iteratively refine the program through a novel iterative prompting mechanism (Sec. 2.3 ), incorporate it into the skill library as a new skill, and index it by the embedding of its description (Fig. 4 , top). For skill retrieval, we query the skill library with the embedding of self-generated task plans and environment feedback (Fig. 4 , bottom). By continuously expanding and refining the skill library, Voyager can learn, adapt, and excel in a wide spectrum of tasks, consistently pushing the boundaries of its capabilities in the open world.
2.3 Iterative Prompting Mechanism
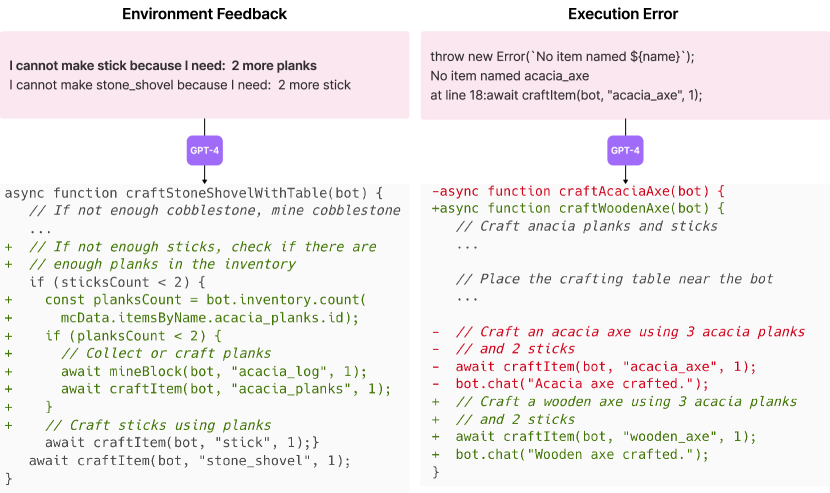
We introduce an iterative prompting mechanism for self-improvement through three types of feedback:
Environment feedback , which illustrates the intermediate progress of program execution (Fig. 5 , left). For example, “ I cannot make an iron chestplate because I need: 7 more iron ingots ” highlights the cause of failure in crafting an iron chestplate. We use bot.chat() inside control primitive APIs to generate environment feedback and prompt GPT-4 to use this function as well during code generation;
Execution errors from the program interpreter that reveal any invalid operations or syntax errors in programs, which are valuable for bug fixing (Fig. 5 , right);
Self-verification for checking task success. Instead of manually coding success checkers for each new task proposed by the automatic curriculum, we instantiate another GPT-4 agent for self-verification. By providing Voyager ’s current state and the task to GPT-4, we ask it to act as a critic [ 47 , 48 , 49 ] and inform us whether the program achieves the task. In addition, if the task fails, it provides a critique by suggesting how to complete the task (Fig. 6 ). Hence, our self-verification is more comprehensive than self-reflection [ 30 ] by both checking success and reflecting on mistakes.
During each round of code generation, we execute the generated program to obtain environment feedback and execution errors from the code interpreter, which are incorporated into GPT-4’s prompt for the next round of code refinement. This iterative process repeats until self-verification validates the task’s completion, at which point we add this new skill to the skill library and ask the automatic curriculum for a new objective (Fig. 2 ). If the agent gets stuck after 4 rounds of code generation, then we query the curriculum for another task. This iterative prompting approach significantly improves program synthesis for embodied control, enabling Voyager to continuously acquire diverse skills without human intervention.
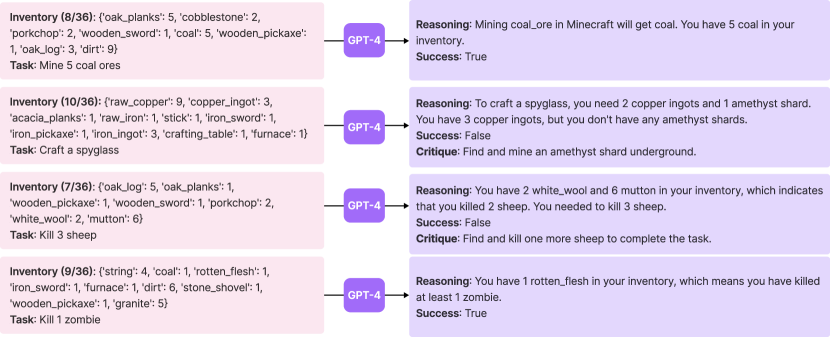
3 Experiments
3.1 experimental setup.
We leverage OpenAI’s gpt-4-0314 [ 35 ] and gpt-3.5-turbo-0301 [ 50 ] APIs for text completion, along with text-embedding-ada-002 [ 51 ] API for text embedding. We set all temperatures to 0 except for the automatic curriculum, which uses temperature = = 0.1 to encourage task diversity. Our simulation environment is built on top of MineDojo [ 23 ] and leverages Mineflayer [ 52 ] JavaScript APIs for motor controls. See Appendix, Sec. B.1 for more details.
3.2 Baselines
Because there is no LLM-based agents that work out of the box for Minecraft, we make our best effort to select a number of representative algorithms as baselines. These methods are originally designed only for NLP tasks without embodiment, therefore we have to re-interpret them to be executable in MineDojo and compatible with our experimental setting:
ReAct [ 29 ] uses chain-of-thought prompting [ 46 ] by generating both reasoning traces and action plans with LLMs. We provide it with our environment feedback and the agent states as observations.
Reflexion [ 30 ] is built on top of ReAct [ 29 ] with self-reflection to infer more intuitive future actions. We provide it with execution errors and our self-verification module.
AutoGPT [ 28 ] is a popular software tool that automates NLP tasks by decomposing a high-level goal into multiple subgoals and executing them in a ReAct-style loop. We re-implement AutoGPT by using GPT-4 to do task decomposition and provide it with the agent states, environment feedback, and execution errors as observations for subgoal execution. Compared with Voyager , AutoGPT lacks the skill library for accumulating knowledge, self-verification for assessing task success, and automatic curriculum for open-ended exploration.
Note that we do not directly compare with prior methods that take Minecraft screen pixels as input and output low-level controls [ 53 , 54 , 55 ] . It would not be an apple-to-apple comparison, because we rely on the high-level Mineflayer [ 52 ] API to control the agent. Our work’s focus is on pushing the limits of GPT-4 for lifelong embodied agent learning, rather than solving the 3D perception or sensorimotor control problems. Voyager is orthogonal and can be combined with gradient-based approaches like VPT [ 8 ] as long as the controller provides a code API. We make a system-level comparison between Voyager and prior Minecraft agents in Table. A.2 .
3.3 Evaluation Results
We systematically evaluate Voyager and baselines on their exploration performance, tech tree mastery, map coverage, and zero-shot generalization capability to novel tasks in a new world.
Significantly better exploration. Results of exploration performance are shown in Fig. 1 . Voyager ’s superiority is evident in its ability to consistently make new strides, discovering 63 unique items within 160 prompting iterations, 3.3 × 3.3\times many novel items compared to its counterparts. On the other hand, AutoGPT lags considerably in discovering new items, while ReAct and Reflexion struggle to make significant progress, given the abstract nature of the open-ended exploration goal that is challenging to execute without an appropriate curriculum.
Consistent tech tree mastery. The Minecraft tech tree tests the agent’s ability to craft and use a hierarchy of tools. Progressing through this tree (wooden tool → → \rightarrow stone tool → → \rightarrow iron tool → → \rightarrow diamond tool) requires the agent to master systematic and compositional skills. Compared with baselines, Voyager unlocks the wooden level 15.3 × 15.3\times faster (in terms of the prompting iterations), the stone level 8.5 × 8.5\times faster, the iron level 6.4 × 6.4\times faster, and Voyager is the only one to unlock the diamond level of the tech tree (Fig. 2 and Table. 1 ). This underscores the effectiveness of the automatic curriculum, which consistently presents challenges of suitable complexity to facilitate the agent’s progress.
Extensive map traversal. Voyager is able to navigate distances 2.3 × 2.3\times longer compared to baselines by traversing a variety of terrains, while the baseline agents often find themselves confined to local areas, which significantly hampers their capacity to discover new knowledge (Fig. 7 ).
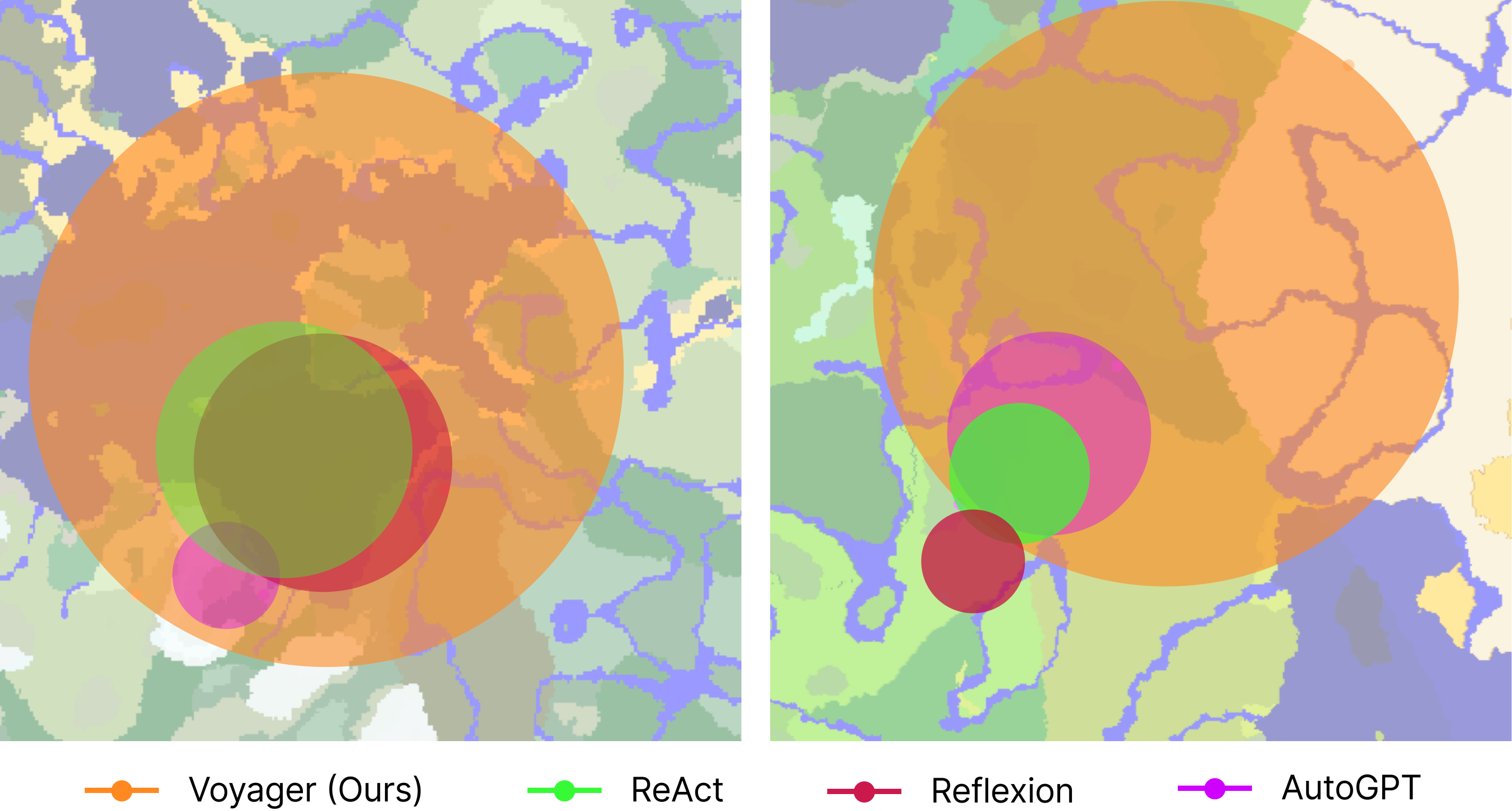
Efficient zero-shot generalization to unseen tasks. To evaluate zero-shot generalization, we clear the agent’s inventory, reset it to a newly instantiated world, and test it with unseen tasks. For both Voyager and AutoGPT, we utilize GPT-4 to break down the task into a series of subgoals. Table. 2 and Fig. 8 show Voyager can consistently solve all the tasks, while baselines cannot solve any task within 50 prompting iterations. What’s interesting to note is that our skill library constructed from lifelong learning not only enhances Voyager ’s performance but also gives a boost to AutoGPT. This demonstrates that the skill library serves as a versatile tool that can be readily employed by other methods, effectively acting as a plug-and-play asset to enhance performance.
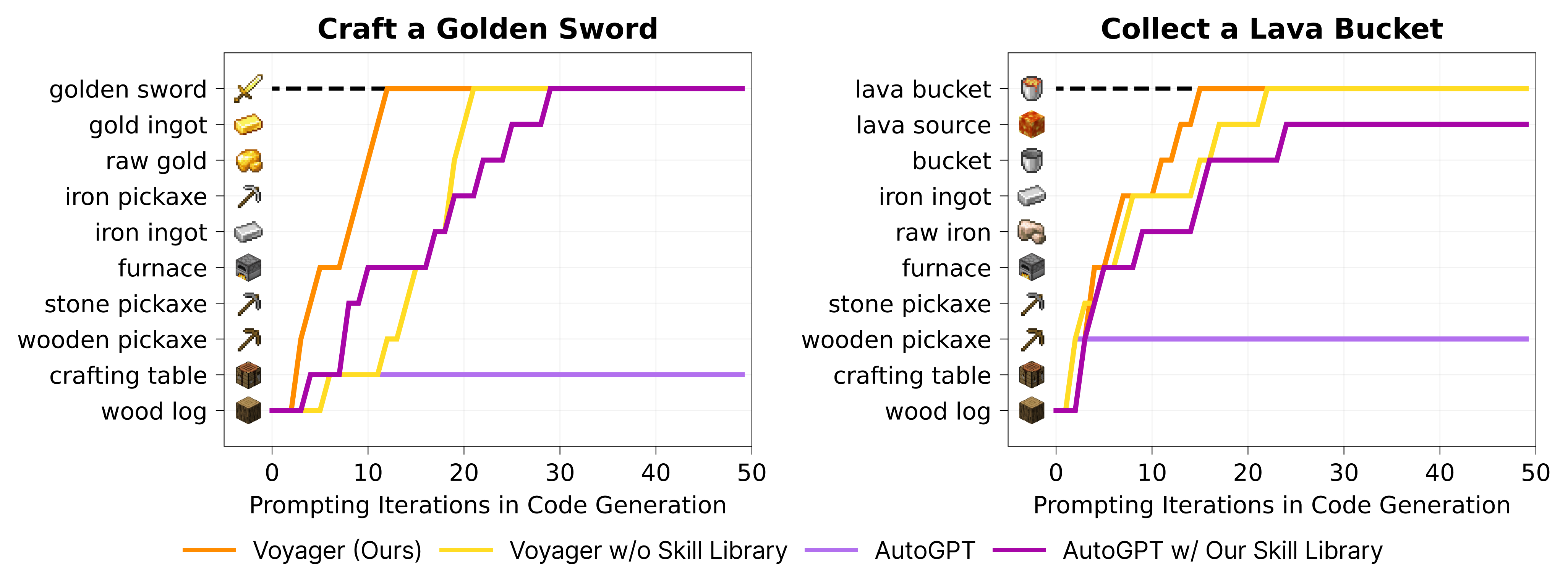

3.4 Ablation Studies
We ablate 6 design choices (automatic curriculum, skill library, environment feedback, execution errors, self-verification, and GPT-4 for code generation) in Voyager and study their impact on exploration performance (see Appendix, Sec. B.3 for details of each ablated variant). Results are shown in Fig. 9 . We highlight the key findings below:
Automatic curriculum is crucial for the agent’s consistent progress. The discovered item count drops by 93 % percent 93 93\% if the curriculum is replaced with a random one, because certain tasks may be too challenging if attempted out of order. On the other hand, a manually designed curriculum requires significant Minecraft-specific expertise, and does not take into account the agent’s live situation. It falls short in the experimental results compared to our automatic curriculum.
Voyager w/o skill library exhibits a tendency to plateau in the later stages. This underscores the pivotal role that the skill library plays in Voyager . It helps create more complex actions and steadily pushes the agent’s boundaries by encouraging new skills to be built upon older ones.
Self-verification is the most important among all the feedback types . Removing the module leads to a significant drop ( − 73 % percent 73 -73\% ) in the discovered item count. Self-verification serves as a critical mechanism to decide when to move on to a new task or reattempt a previously unsuccessful task.
GPT-4 significantly outperforms GPT-3.5 in code generation and obtains 5.7 × 5.7\times more unique items, as GPT-4 exhibits a quantum leap in coding abilities. This finding corroborates recent studies in the literature [ 56 , 57 ] .
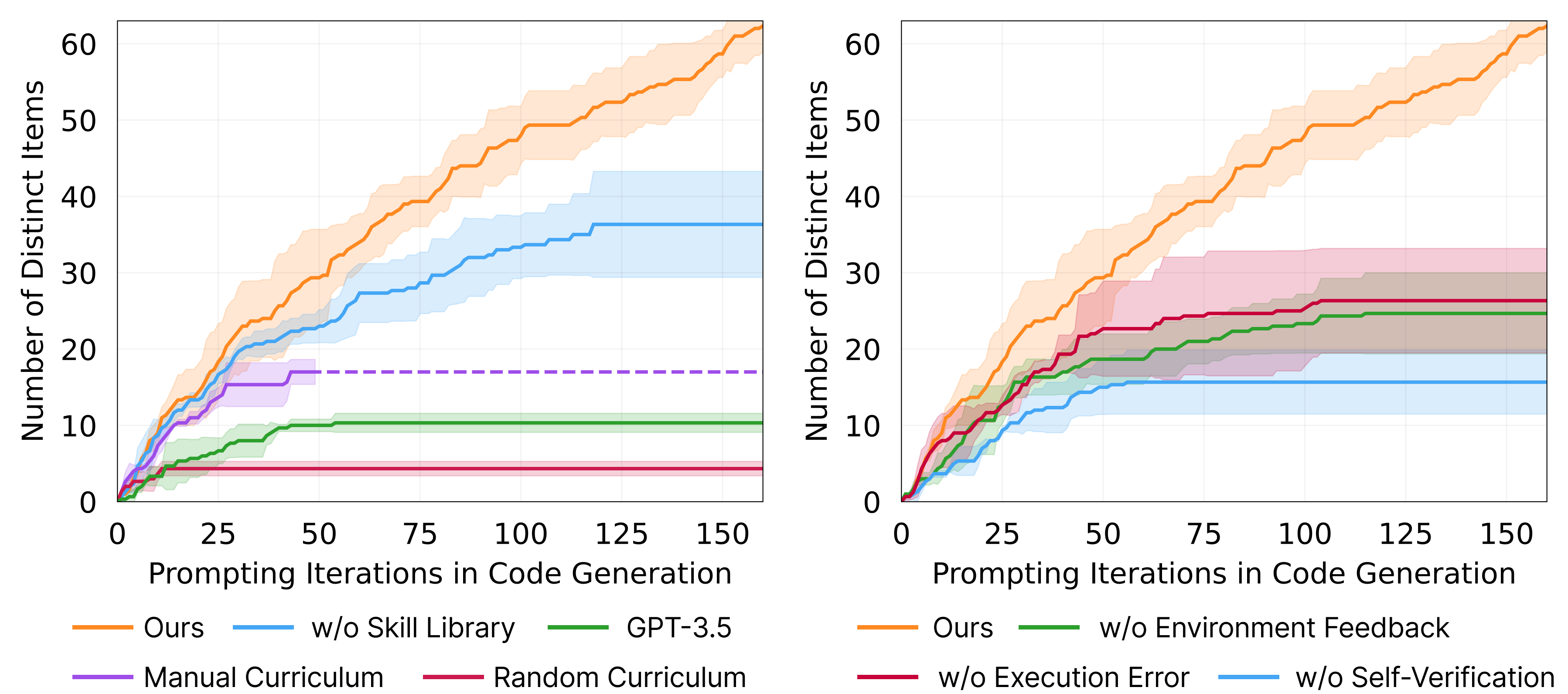
3.5 Multimodal Feedback from Humans
Voyager does not currently support visual perception, because the available version of GPT-4 API is text-only at the time of this writing. However, Voyager has the potential to be augmented by multimodal perception models [ 58 , 59 ] to achieve more impressive tasks. We demonstrate that given human feedback, Voyager is able to construct complex 3D structures in Minecraft, such as a Nether Portal and a house (Fig. 10 ). There are two ways to integrate human feedback:
Human as a critic (equivalent to Voyager ’s self-verification module): humans provide visual critique to Voyager , allowing it to modify the code from the previous round. This feedback is essential for correcting certain errors in the spatial details of a 3D structure that Voyager cannot perceive directly.
Human as a curriculum (equivalent to Voyager ’s automatic curriculum module): humans break down a complex building task into smaller steps, guiding Voyager to complete them incrementally. This approach improves Voyager ’s ability to handle more sophisticated 3D construction tasks.
4 Limitations and Future Work
Cost. The GPT-4 API incurs significant costs. It is 15 × 15\times more expensive than GPT-3.5. Nevertheless, Voyager requires the quantum leap in code generation quality from GPT-4 (Fig. 9 ), which GPT-3.5 and open-source LLMs cannot provide [ 60 ] .
Inaccuracies. Despite the iterative prompting mechanism, there are still cases where the agent gets stuck and fails to generate the correct skill. The automatic curriculum has the flexibility to reattempt this task at a later time. Occasionally, self-verification module may also fail, such as not recognizing spider string as a success signal of beating a spider.
Hallucinations. The automatic curriculum occasionally proposes unachievable tasks. For example, it may ask the agent to craft a “copper sword" or “copper chestplate", which are items that do not exist within the game. Hallucinations also occur during the code generation process. For instance, GPT-4 tends to use cobblestone as a fuel input, despite being an invalid fuel source in the game. Additionally, it may call functions absent in the provided control primitive APIs, leading to code execution errors.
We are confident that improvements in the GPT API models as well as novel techniques for finetuning open-source LLMs will overcome these limitations in the future.
5 Related work
Decision-making agents in minecraft..
Minecraft is an open-ended 3D world with incredibly flexible game mechanics supporting a broad spectrum of activities. Built upon notable Minecraft benchmarks [ 23 , 61 , 62 , 63 , 64 , 65 ] , Minecraft learning algorithms can be divided into two categories: 1) Low-level controller: Many prior efforts leverage hierarchical reinforcement learning to learn from human demonstrations [ 66 , 67 , 68 ] . Kanitscheider et al. [ 14 ] design a curriculum based on success rates, but its objectives are limited to curated items. MineDojo [ 23 ] and VPT [ 8 ] utilize YouTube videos for large-scale pre-training. DreamerV3 [ 69 ] , on the other hand, learns a world model to explore the environment and collect diamonds. 2) High-level planner: Volum et al. [ 70 ] leverage few-shot prompting with Codex [ 41 ] to generate executable policies, but they require additional human interaction. Recent works leverage LLMs as a high-level planner in Minecraft by decomposing a high-level task into several subgoals following Minecraft recipes [ 55 , 53 , 71 ] , thus lacking full exploration flexibility. Like these latter works, Voyager also uses LLMs as a high-level planner by prompting GPT-4 and utilizes Mineflayer [ 52 ] as a low-level controller following Volum et al. [ 70 ] . Unlike prior works, Voyager employs an automatic curriculum that unfolds in a bottom-up manner, driven by curiosity, and therefore enables open-ended exploration.
Large Language Models for Agent Planning.
Inspired by the strong emergent capabilities of LLMs, such as zero-shot prompting and complex reasoning [ 72 , 37 , 38 , 36 , 73 , 74 ] , embodied agent research [ 75 , 76 , 77 , 78 ] has witnessed a significant increase in the utilization of LLMs for planning purposes. Recent efforts can be roughly classified into two groups. 1) Large language models for robot learning: Many prior works apply LLMs to generate subgoals for robot planning [ 27 , 27 , 25 , 79 , 80 ] . Inner Monologue [ 26 ] incorporates environment feedback for robot planning with LLMs. Code as Policies [ 16 ] and ProgPrompt [ 22 ] directly leverage LLMs to generate executable robot policies. VIMA [ 19 ] and PaLM-E [ 59 ] fine-tune pre-trained LLMs to support multimodal prompts. 2) Large language models for text agents: ReAct [ 29 ] leverages chain-of-thought prompting [ 46 ] and generates both reasoning traces and task-specific actions with LLMs. Reflexion [ 30 ] is built upon ReAct [ 29 ] with self-reflection to enhance reasoning. AutoGPT [ 28 ] is a popular tool that automates NLP tasks by crafting a curriculum of multiple subgoals for completing a high-level goal while incorporating ReAct [ 29 ] ’s reasoning and acting loops. DERA [ 81 ] frames a task as a dialogue between two GPT-4 [ 35 ] agents. Generative Agents [ 82 ] leverages ChatGPT [ 50 ] to simulate human behaviors by storing agents’ experiences as memories and retrieving those for planning, but its agent actions are not executable. SPRING [ 83 ] is a concurrent work that uses GPT-4 to extract game mechanics from game manuals, based on which it answers questions arranged in a directed acyclic graph and predicts the next action. All these works lack a skill library for developing more complex behaviors, which are crucial components for the success of Voyager in lifelong learning.
Code Generation with Execution.
Code generation has been a longstanding challenge in NLP [ 41 , 84 , 85 , 73 , 37 ] , with various works leveraging execution results to improve program synthesis. Execution-guided approaches leverage intermediate execution outcomes to guide program search [ 86 , 87 , 88 ] . Another line of research utilizes majority voting to choose candidates based on their execution performance [ 89 , 90 ] . Additionally, LEVER [ 91 ] trains a verifier to distinguish and reject incorrect programs based on execution results. CLAIRIFY [ 92 ] , on the other hand, generates code for planning chemistry experiments and makes use of a rule-based verifier to iteratively provide error feedback to LLMs. Voyager distinguishes itself from these works by integrating environment feedback, execution errors, and self-verification (to assess task success) into an iterative prompting mechanism for embodied control.
6 Conclusion
In this work, we introduce Voyager , the first LLM-powered embodied lifelong learning agent, which leverages GPT-4 to explore the world continuously, develop increasingly sophisticated skills, and make new discoveries consistently without human intervention. Voyager exhibits superior performance in discovering novel items, unlocking the Minecraft tech tree, traversing diverse terrains, and applying its learned skill library to unseen tasks in a newly instantiated world. Voyager serves as a starting point to develop powerful generalist agents without tuning the model parameters.
7 Broader Impacts
Our research is conducted within Minecraft, a safe and harmless 3D video game environment. While Voyager is designed to be generally applicable to other domains, such as robotics, its application to physical robots would require additional attention and the implementation of safety constraints by humans to ensure responsible and secure deployment.
8 Acknowledgements
We are extremely grateful to Ziming Zhu, Kaiyu Yang, Rafał Kocielnik, Colin White, Or Sharir, Sahin Lale, De-An Huang, Jean Kossaifi, Yuncong Yang, Charles Zhang, Minchao Huang, and many other colleagues and friends for their helpful feedback and insightful discussions. This work is done during Guanzhi Wang’s internship at NVIDIA. Guanzhi Wang is supported by the Kortschak fellowship in Computing and Mathematical Sciences at Caltech.
- [1] Eric Kolve, Roozbeh Mottaghi, Winson Han, Eli VanderBilt, Luca Weihs, Alvaro Herrasti, Daniel Gordon, Yuke Zhu, Abhinav Gupta, and Ali Farhadi. Ai2-thor: An interactive 3d environment for visual ai. arXiv preprint arXiv: Arxiv-1712.05474 , 2017.
- [2] Manolis Savva, Jitendra Malik, Devi Parikh, Dhruv Batra, Abhishek Kadian, Oleksandr Maksymets, Yili Zhao, Erik Wijmans, Bhavana Jain, Julian Straub, Jia Liu, and Vladlen Koltun. Habitat: A platform for embodied AI research. In 2019 IEEE/CVF International Conference on Computer Vision, ICCV 2019, Seoul, Korea (South), October 27 - November 2, 2019 , pages 9338–9346. IEEE, 2019.
- [3] Yuke Zhu, Josiah Wong, Ajay Mandlekar, and Roberto Martín-Martín. robosuite: A modular simulation framework and benchmark for robot learning. arXiv preprint arXiv: Arxiv-2009.12293 , 2020.
- [4] Fei Xia, William B. Shen, Chengshu Li, Priya Kasimbeg, Micael Tchapmi, Alexander Toshev, Li Fei-Fei, Roberto Martín-Martín, and Silvio Savarese. Interactive gibson benchmark (igibson 0.5): A benchmark for interactive navigation in cluttered environments. arXiv preprint arXiv: Arxiv-1910.14442 , 2019.
- [5] Bokui Shen, Fei Xia, Chengshu Li, Roberto Martín-Martín, Linxi Fan, Guanzhi Wang, Claudia Pérez-D’Arpino, Shyamal Buch, Sanjana Srivastava, Lyne P. Tchapmi, Micael E. Tchapmi, Kent Vainio, Josiah Wong, Li Fei-Fei, and Silvio Savarese. igibson 1.0: a simulation environment for interactive tasks in large realistic scenes. arXiv preprint arXiv: Arxiv-2012.02924 , 2020.
- [6] Jens Kober, J Andrew Bagnell, and Jan Peters. Reinforcement learning in robotics: A survey. The International Journal of Robotics Research , 32(11):1238–1274, 2013.
- [7] Kai Arulkumaran, Marc Peter Deisenroth, Miles Brundage, and Anil Anthony Bharath. Deep reinforcement learning: A brief survey. IEEE Signal Processing Magazine , 34(6):26–38, 2017.
- [8] Bowen Baker, Ilge Akkaya, Peter Zhokhov, Joost Huizinga, Jie Tang, Adrien Ecoffet, Brandon Houghton, Raul Sampedro, and Jeff Clune. Video pretraining (vpt): Learning to act by watching unlabeled online videos. arXiv preprint arXiv: Arxiv-2206.11795 , 2022.
- [9] DeepMind Interactive Agents Team, Josh Abramson, Arun Ahuja, Arthur Brussee, Federico Carnevale, Mary Cassin, Felix Fischer, Petko Georgiev, Alex Goldin, Mansi Gupta, Tim Harley, Felix Hill, Peter C Humphreys, Alden Hung, Jessica Landon, Timothy Lillicrap, Hamza Merzic, Alistair Muldal, Adam Santoro, Guy Scully, Tamara von Glehn, Greg Wayne, Nathaniel Wong, Chen Yan, and Rui Zhu. Creating multimodal interactive agents with imitation and self-supervised learning. arXiv preprint arXiv: Arxiv-2112.03763 , 2021.
- [10] Oriol Vinyals, Igor Babuschkin, Junyoung Chung, Michael Mathieu, Max Jaderberg, Wojciech M Czarnecki, Andrew Dudzik, Aja Huang, Petko Georgiev, Richard Powell, et al. Alphastar: Mastering the real-time strategy game starcraft ii. DeepMind blog , 2, 2019.
- [11] Adrien Ecoffet, Joost Huizinga, Joel Lehman, Kenneth O. Stanley, and Jeff Clune. Go-explore: a new approach for hard-exploration problems. arXiv preprint arXiv: Arxiv-1901.10995 , 2019.
- [12] Joost Huizinga and Jeff Clune. Evolving multimodal robot behavior via many stepping stones with the combinatorial multiobjective evolutionary algorithm. Evolutionary computation , 30(2):131–164, 2022.
- [13] Rui Wang, Joel Lehman, Aditya Rawal, Jiale Zhi, Yulun Li, Jeffrey Clune, and Kenneth O. Stanley. Enhanced POET: open-ended reinforcement learning through unbounded invention of learning challenges and their solutions. In Proceedings of the 37th International Conference on Machine Learning, ICML 2020, 13-18 July 2020, Virtual Event , volume 119 of Proceedings of Machine Learning Research , pages 9940–9951. PMLR, 2020.
- [14] Ingmar Kanitscheider, Joost Huizinga, David Farhi, William Hebgen Guss, Brandon Houghton, Raul Sampedro, Peter Zhokhov, Bowen Baker, Adrien Ecoffet, Jie Tang, Oleg Klimov, and Jeff Clune. Multi-task curriculum learning in a complex, visual, hard-exploration domain: Minecraft. arXiv preprint arXiv: Arxiv-2106.14876 , 2021.
- [15] Michael Dennis, Natasha Jaques, Eugene Vinitsky, Alexandre M. Bayen, Stuart Russell, Andrew Critch, and Sergey Levine. Emergent complexity and zero-shot transfer via unsupervised environment design. In Hugo Larochelle, Marc’Aurelio Ranzato, Raia Hadsell, Maria-Florina Balcan, and Hsuan-Tien Lin, editors, Advances in Neural Information Processing Systems 33: Annual Conference on Neural Information Processing Systems 2020, NeurIPS 2020, December 6-12, 2020, virtual , 2020.
- [16] Jacky Liang, Wenlong Huang, Fei Xia, Peng Xu, Karol Hausman, Brian Ichter, Pete Florence, and Andy Zeng. Code as policies: Language model programs for embodied control. arXiv preprint arXiv: Arxiv-2209.07753 , 2022.
- [17] Shao-Hua Sun, Te-Lin Wu, and Joseph J. Lim. Program guided agent. In 8th International Conference on Learning Representations, ICLR 2020, Addis Ababa, Ethiopia, April 26-30, 2020 . OpenReview.net, 2020.
- [18] Zelin Zhao, Karan Samel, Binghong Chen, and Le Song. Proto: Program-guided transformer for program-guided tasks. In Marc’Aurelio Ranzato, Alina Beygelzimer, Yann N. Dauphin, Percy Liang, and Jennifer Wortman Vaughan, editors, Advances in Neural Information Processing Systems 34: Annual Conference on Neural Information Processing Systems 2021, NeurIPS 2021, December 6-14, 2021, virtual , pages 17021–17036, 2021.
- [19] Yunfan Jiang, Agrim Gupta, Zichen Zhang, Guanzhi Wang, Yongqiang Dou, Yanjun Chen, Li Fei-Fei, Anima Anandkumar, Yuke Zhu, and Linxi (Jim) Fan. Vima: General robot manipulation with multimodal prompts. ARXIV.ORG , 2022.
- [20] Mohit Shridhar, Lucas Manuelli, and Dieter Fox. Cliport: What and where pathways for robotic manipulation. arXiv preprint arXiv: Arxiv-2109.12098 , 2021.
- [21] Linxi Fan, Guanzhi Wang, De-An Huang, Zhiding Yu, Li Fei-Fei, Yuke Zhu, and Animashree Anandkumar. SECANT: self-expert cloning for zero-shot generalization of visual policies. In Marina Meila and Tong Zhang, editors, Proceedings of the 38th International Conference on Machine Learning, ICML 2021, 18-24 July 2021, Virtual Event , volume 139 of Proceedings of Machine Learning Research , pages 3088–3099. PMLR, 2021.
- [22] Ishika Singh, Valts Blukis, Arsalan Mousavian, Ankit Goyal, Danfei Xu, Jonathan Tremblay, Dieter Fox, Jesse Thomason, and Animesh Garg. Progprompt: Generating situated robot task plans using large language models. arXiv preprint arXiv: Arxiv-2209.11302 , 2022.
- [23] Linxi Fan, Guanzhi Wang, Yunfan Jiang, Ajay Mandlekar, Yuncong Yang, Haoyi Zhu, Andrew Tang, De-An Huang, Yuke Zhu, and Anima Anandkumar. Minedojo: Building open-ended embodied agents with internet-scale knowledge. arXiv preprint arXiv: Arxiv-2206.08853 , 2022.
- [24] Andy Zeng, Adrian Wong, Stefan Welker, Krzysztof Choromanski, Federico Tombari, Aveek Purohit, Michael Ryoo, Vikas Sindhwani, Johnny Lee, Vincent Vanhoucke, and Pete Florence. Socratic models: Composing zero-shot multimodal reasoning with language. arXiv preprint arXiv: Arxiv-2204.00598 , 2022.
- [25] Michael Ahn, Anthony Brohan, Noah Brown, Yevgen Chebotar, Omar Cortes, Byron David, Chelsea Finn, Keerthana Gopalakrishnan, Karol Hausman, Alex Herzog, Daniel Ho, Jasmine Hsu, Julian Ibarz, Brian Ichter, Alex Irpan, Eric Jang, Rosario Jauregui Ruano, Kyle Jeffrey, Sally Jesmonth, Nikhil J Joshi, Ryan Julian, Dmitry Kalashnikov, Yuheng Kuang, Kuang-Huei Lee, Sergey Levine, Yao Lu, Linda Luu, Carolina Parada, Peter Pastor, Jornell Quiambao, Kanishka Rao, Jarek Rettinghouse, Diego Reyes, Pierre Sermanet, Nicolas Sievers, Clayton Tan, Alexander Toshev, Vincent Vanhoucke, Fei Xia, Ted Xiao, Peng Xu, Sichun Xu, and Mengyuan Yan. Do as i can, not as i say: Grounding language in robotic affordances. arXiv preprint arXiv: Arxiv-2204.01691 , 2022.
- [26] Wenlong Huang, Fei Xia, Ted Xiao, Harris Chan, Jacky Liang, Pete Florence, Andy Zeng, Jonathan Tompson, Igor Mordatch, Yevgen Chebotar, Pierre Sermanet, Noah Brown, Tomas Jackson, Linda Luu, Sergey Levine, Karol Hausman, and Brian Ichter. Inner monologue: Embodied reasoning through planning with language models. arXiv preprint arXiv: Arxiv-2207.05608 , 2022.
- [27] Wenlong Huang, Pieter Abbeel, Deepak Pathak, and Igor Mordatch. Language models as zero-shot planners: Extracting actionable knowledge for embodied agents. In Kamalika Chaudhuri, Stefanie Jegelka, Le Song, Csaba Szepesvári, Gang Niu, and Sivan Sabato, editors, International Conference on Machine Learning, ICML 2022, 17-23 July 2022, Baltimore, Maryland, USA , volume 162 of Proceedings of Machine Learning Research , pages 9118–9147. PMLR, 2022.
- [28] Significant-gravitas/auto-gpt: An experimental open-source attempt to make gpt-4 fully autonomous., 2023.
- [29] Shunyu Yao, Jeffrey Zhao, Dian Yu, Nan Du, Izhak Shafran, Karthik Narasimhan, and Yuan Cao. React: Synergizing reasoning and acting in language models. arXiv preprint arXiv: Arxiv-2210.03629 , 2022.
- [30] Noah Shinn, Beck Labash, and Ashwin Gopinath. Reflexion: an autonomous agent with dynamic memory and self-reflection. arXiv preprint arXiv: Arxiv-2303.11366 , 2023.
- [31] German Ignacio Parisi, Ronald Kemker, Jose L. Part, Christopher Kanan, and Stefan Wermter. Continual lifelong learning with neural networks: A review. Neural Networks , 113:54–71, 2019.
- [32] Liyuan Wang, Xingxing Zhang, Hang Su, and Jun Zhu. A comprehensive survey of continual learning: Theory, method and application. arXiv preprint arXiv: Arxiv-2302.00487 , 2023.
- [33] Volodymyr Mnih, Koray Kavukcuoglu, David Silver, Alex Graves, Ioannis Antonoglou, Daan Wierstra, and Martin Riedmiller. Playing atari with deep reinforcement learning. arXiv preprint arXiv: Arxiv-1312.5602 , 2013.
- [34] OpenAI, :, Christopher Berner, Greg Brockman, Brooke Chan, Vicki Cheung, Przemysław Dębiak, Christy Dennison, David Farhi, Quirin Fischer, Shariq Hashme, Chris Hesse, Rafal Józefowicz, Scott Gray, Catherine Olsson, Jakub Pachocki, Michael Petrov, Henrique P. d. O. Pinto, Jonathan Raiman, Tim Salimans, Jeremy Schlatter, Jonas Schneider, Szymon Sidor, Ilya Sutskever, Jie Tang, Filip Wolski, and Susan Zhang. Dota 2 with large scale deep reinforcement learning. arXiv preprint arXiv: Arxiv-1912.06680 , 2019.
- [35] OpenAI. Gpt-4 technical report. arXiv preprint arXiv: Arxiv-2303.08774 , 2023.
- [36] Jason Wei, Yi Tay, Rishi Bommasani, Colin Raffel, Barret Zoph, Sebastian Borgeaud, Dani Yogatama, Maarten Bosma, Denny Zhou, Donald Metzler, Ed H. Chi, Tatsunori Hashimoto, Oriol Vinyals, Percy Liang, Jeff Dean, and William Fedus. Emergent abilities of large language models. arXiv preprint arXiv: Arxiv-2206.07682 , 2022.
- [37] Tom B. Brown, Benjamin Mann, Nick Ryder, Melanie Subbiah, Jared Kaplan, Prafulla Dhariwal, Arvind Neelakantan, Pranav Shyam, Girish Sastry, Amanda Askell, Sandhini Agarwal, Ariel Herbert-Voss, Gretchen Krueger, Tom Henighan, Rewon Child, Aditya Ramesh, Daniel M. Ziegler, Jeffrey Wu, Clemens Winter, Christopher Hesse, Mark Chen, Eric Sigler, Mateusz Litwin, Scott Gray, Benjamin Chess, Jack Clark, Christopher Berner, Sam McCandlish, Alec Radford, Ilya Sutskever, and Dario Amodei. Language models are few-shot learners. In Hugo Larochelle, Marc’Aurelio Ranzato, Raia Hadsell, Maria-Florina Balcan, and Hsuan-Tien Lin, editors, Advances in Neural Information Processing Systems 33: Annual Conference on Neural Information Processing Systems 2020, NeurIPS 2020, December 6-12, 2020, virtual , 2020.
- [38] Colin Raffel, Noam Shazeer, Adam Roberts, Katherine Lee, Sharan Narang, Michael Matena, Yanqi Zhou, Wei Li, and Peter J. Liu. Exploring the limits of transfer learning with a unified text-to-text transformer. J. Mach. Learn. Res. , 21:140:1–140:67, 2020.
- [39] Benjamin Eysenbach, Abhishek Gupta, Julian Ibarz, and Sergey Levine. Diversity is all you need: Learning skills without a reward function. In 7th International Conference on Learning Representations, ICLR 2019, New Orleans, LA, USA, May 6-9, 2019 . OpenReview.net, 2019.
- [40] Edoardo Conti, Vashisht Madhavan, Felipe Petroski Such, Joel Lehman, Kenneth O. Stanley, and Jeff Clune. Improving exploration in evolution strategies for deep reinforcement learning via a population of novelty-seeking agents. In Samy Bengio, Hanna M. Wallach, Hugo Larochelle, Kristen Grauman, Nicolò Cesa-Bianchi, and Roman Garnett, editors, Advances in Neural Information Processing Systems 31: Annual Conference on Neural Information Processing Systems 2018, NeurIPS 2018, December 3-8, 2018, Montréal, Canada , pages 5032–5043, 2018.
- [41] Mark Chen, Jerry Tworek, Heewoo Jun, Qiming Yuan, Henrique Ponde de Oliveira Pinto, Jared Kaplan, Harri Edwards, Yuri Burda, Nicholas Joseph, Greg Brockman, Alex Ray, Raul Puri, Gretchen Krueger, Michael Petrov, Heidy Khlaaf, Girish Sastry, Pamela Mishkin, Brooke Chan, Scott Gray, Nick Ryder, Mikhail Pavlov, Alethea Power, Lukasz Kaiser, Mohammad Bavarian, Clemens Winter, Philippe Tillet, Felipe Petroski Such, Dave Cummings, Matthias Plappert, Fotios Chantzis, Elizabeth Barnes, Ariel Herbert-Voss, William Hebgen Guss, Alex Nichol, Alex Paino, Nikolas Tezak, Jie Tang, Igor Babuschkin, Suchir Balaji, Shantanu Jain, William Saunders, Christopher Hesse, Andrew N. Carr, Jan Leike, Josh Achiam, Vedant Misra, Evan Morikawa, Alec Radford, Matthew Knight, Miles Brundage, Mira Murati, Katie Mayer, Peter Welinder, Bob McGrew, Dario Amodei, Sam McCandlish, Ilya Sutskever, and Wojciech Zaremba. Evaluating large language models trained on code. arXiv preprint arXiv: Arxiv-2107.03374 , 2021.
- [42] Rui Wang, Joel Lehman, Jeff Clune, and Kenneth O. Stanley. Paired open-ended trailblazer (poet): Endlessly generating increasingly complex and diverse learning environments and their solutions. arXiv preprint arXiv: Arxiv-1901.01753 , 2019.
- [43] Rémy Portelas, Cédric Colas, Lilian Weng, Katja Hofmann, and Pierre-Yves Oudeyer. Automatic curriculum learning for deep RL: A short survey. In Christian Bessiere, editor, Proceedings of the Twenty-Ninth International Joint Conference on Artificial Intelligence, IJCAI 2020 , pages 4819–4825. ijcai.org, 2020.
- [44] Sébastien Forestier, Rémy Portelas, Yoan Mollard, and Pierre-Yves Oudeyer. Intrinsically motivated goal exploration processes with automatic curriculum learning. The Journal of Machine Learning Research , 23(1):6818–6858, 2022.
- [45] Kevin Ellis, Catherine Wong, Maxwell Nye, Mathias Sable-Meyer, Luc Cary, Lucas Morales, Luke Hewitt, Armando Solar-Lezama, and Joshua B. Tenenbaum. Dreamcoder: Growing generalizable, interpretable knowledge with wake-sleep bayesian program learning. arXiv preprint arXiv: Arxiv-2006.08381 , 2020.
- [46] Jason Wei, Xuezhi Wang, Dale Schuurmans, Maarten Bosma, Ed Chi, Quoc Le, and Denny Zhou. Chain of thought prompting elicits reasoning in large language models. arXiv preprint arXiv: Arxiv-2201.11903 , 2022.
- [47] Volodymyr Mnih, Adrià Puigdomènech Badia, Mehdi Mirza, Alex Graves, Timothy P. Lillicrap, Tim Harley, David Silver, and Koray Kavukcuoglu. Asynchronous methods for deep reinforcement learning. In Maria-Florina Balcan and Kilian Q. Weinberger, editors, Proceedings of the 33nd International Conference on Machine Learning, ICML 2016, New York City, NY, USA, June 19-24, 2016 , volume 48 of JMLR Workshop and Conference Proceedings , pages 1928–1937. JMLR.org, 2016.
- [48] John Schulman, Filip Wolski, Prafulla Dhariwal, Alec Radford, and Oleg Klimov. Proximal policy optimization algorithms. arXiv preprint arXiv: Arxiv-1707.06347 , 2017.
- [49] Timothy P. Lillicrap, Jonathan J. Hunt, Alexander Pritzel, Nicolas Heess, Tom Erez, Yuval Tassa, David Silver, and Daan Wierstra. Continuous control with deep reinforcement learning. In Yoshua Bengio and Yann LeCun, editors, 4th International Conference on Learning Representations, ICLR 2016, San Juan, Puerto Rico, May 2-4, 2016, Conference Track Proceedings , 2016.
- [50] Introducing chatgpt, 2022.
- [51] New and improved embedding model, 2022.
- [52] PrismarineJS. Prismarinejs/mineflayer: Create minecraft bots with a powerful, stable, and high level javascript api., 2013.
- [53] Kolby Nottingham, Prithviraj Ammanabrolu, Alane Suhr, Yejin Choi, Hanna Hajishirzi, Sameer Singh, and Roy Fox. Do embodied agents dream of pixelated sheep?: Embodied decision making using language guided world modelling. ARXIV.ORG , 2023.
- [54] Shaofei Cai, Zihao Wang, Xiaojian Ma, Anji Liu, and Yitao Liang. Open-world multi-task control through goal-aware representation learning and adaptive horizon prediction. arXiv preprint arXiv: Arxiv-2301.10034 , 2023.
- [55] Zihao Wang, Shaofei Cai, Anji Liu, Xiaojian Ma, and Yitao Liang. Describe, explain, plan and select: Interactive planning with large language models enables open-world multi-task agents. arXiv preprint arXiv: Arxiv-2302.01560 , 2023.
- [56] Sébastien Bubeck, Varun Chandrasekaran, Ronen Eldan, Johannes Gehrke, Eric Horvitz, Ece Kamar, Peter Lee, Yin Tat Lee, Yuanzhi Li, Scott Lundberg, Harsha Nori, Hamid Palangi, Marco Tulio Ribeiro, and Yi Zhang. Sparks of artificial general intelligence: Early experiments with gpt-4. arXiv preprint arXiv: Arxiv-2303.12712 , 2023.
- [57] Yiheng Liu, Tianle Han, Siyuan Ma, Jiayue Zhang, Yuanyuan Yang, Jiaming Tian, Hao He, Antong Li, Mengshen He, Zhengliang Liu, Zihao Wu, Dajiang Zhu, Xiang Li, Ning Qiang, Dingang Shen, Tianming Liu, and Bao Ge. Summary of chatgpt/gpt-4 research and perspective towards the future of large language models. arXiv preprint arXiv: Arxiv-2304.01852 , 2023.
- [58] Shikun Liu, Linxi Fan, Edward Johns, Zhiding Yu, Chaowei Xiao, and Anima Anandkumar. Prismer: A vision-language model with an ensemble of experts. arXiv preprint arXiv: Arxiv-2303.02506 , 2023.
- [59] Danny Driess, Fei Xia, Mehdi S. M. Sajjadi, Corey Lynch, Aakanksha Chowdhery, Brian Ichter, Ayzaan Wahid, Jonathan Tompson, Quan Vuong, Tianhe Yu, Wenlong Huang, Yevgen Chebotar, Pierre Sermanet, Daniel Duckworth, Sergey Levine, Vincent Vanhoucke, Karol Hausman, Marc Toussaint, Klaus Greff, Andy Zeng, Igor Mordatch, and Pete Florence. Palm-e: An embodied multimodal language model. arXiv preprint arXiv: Arxiv-2303.03378 , 2023.
- [60] Hugo Touvron, Thibaut Lavril, Gautier Izacard, Xavier Martinet, Marie-Anne Lachaux, Timothée Lacroix, Baptiste Rozière, Naman Goyal, Eric Hambro, Faisal Azhar, Aurelien Rodriguez, Armand Joulin, Edouard Grave, and Guillaume Lample. Llama: Open and efficient foundation language models. arXiv preprint arXiv: Arxiv-2302.13971 , 2023.
- [61] William H. Guss, Brandon Houghton, Nicholay Topin, Phillip Wang, Cayden Codel, Manuela Veloso, and Ruslan Salakhutdinov. Minerl: A large-scale dataset of minecraft demonstrations. In Sarit Kraus, editor, Proceedings of the Twenty-Eighth International Joint Conference on Artificial Intelligence, IJCAI 2019, Macao, China, August 10-16, 2019 , pages 2442–2448. ijcai.org, 2019.
- [62] William H. Guss, Cayden Codel, Katja Hofmann, Brandon Houghton, Noboru Kuno, Stephanie Milani, Sharada Mohanty, Diego Perez Liebana, Ruslan Salakhutdinov, Nicholay Topin, Manuela Veloso, and Phillip Wang. The minerl 2019 competition on sample efficient reinforcement learning using human priors. arXiv preprint arXiv: Arxiv-1904.10079 , 2019.
- [63] William H. Guss, Mario Ynocente Castro, Sam Devlin, Brandon Houghton, Noboru Sean Kuno, Crissman Loomis, Stephanie Milani, Sharada Mohanty, Keisuke Nakata, Ruslan Salakhutdinov, John Schulman, Shinya Shiroshita, Nicholay Topin, Avinash Ummadisingu, and Oriol Vinyals. The minerl 2020 competition on sample efficient reinforcement learning using human priors. arXiv preprint arXiv: Arxiv-2101.11071 , 2021.
- [64] Anssi Kanervisto, Stephanie Milani, Karolis Ramanauskas, Nicholay Topin, Zichuan Lin, Junyou Li, Jianing Shi, Deheng Ye, Qiang Fu, Wei Yang, Weijun Hong, Zhongyue Huang, Haicheng Chen, Guangjun Zeng, Yue Lin, Vincent Micheli, Eloi Alonso, François Fleuret, Alexander Nikulin, Yury Belousov, Oleg Svidchenko, and Aleksei Shpilman. Minerl diamond 2021 competition: Overview, results, and lessons learned. arXiv preprint arXiv: Arxiv-2202.10583 , 2022.
- [65] Matthew Johnson, Katja Hofmann, Tim Hutton, and David Bignell. The malmo platform for artificial intelligence experimentation. In Subbarao Kambhampati, editor, Proceedings of the Twenty-Fifth International Joint Conference on Artificial Intelligence, IJCAI 2016, New York, NY, USA, 9-15 July 2016 , pages 4246–4247. IJCAI/AAAI Press, 2016.
- [66] Zichuan Lin, Junyou Li, Jianing Shi, Deheng Ye, Qiang Fu, and Wei Yang. Juewu-mc: Playing minecraft with sample-efficient hierarchical reinforcement learning. arXiv preprint arXiv: Arxiv-2112.04907 , 2021.
- [67] Hangyu Mao, Chao Wang, Xiaotian Hao, Yihuan Mao, Yiming Lu, Chengjie Wu, Jianye Hao, Dong Li, and Pingzhong Tang. Seihai: A sample-efficient hierarchical ai for the minerl competition. arXiv preprint arXiv: Arxiv-2111.08857 , 2021.
- [68] Alexey Skrynnik, Aleksey Staroverov, Ermek Aitygulov, Kirill Aksenov, Vasilii Davydov, and Aleksandr I. Panov. Hierarchical deep q-network from imperfect demonstrations in minecraft. Cogn. Syst. Res. , 65:74–78, 2021.
- [69] Danijar Hafner, Jurgis Pasukonis, Jimmy Ba, and Timothy Lillicrap. Mastering diverse domains through world models. arXiv preprint arXiv: Arxiv-2301.04104 , 2023.
- [70] Ryan Volum, Sudha Rao, Michael Xu, Gabriel DesGarennes, Chris Brockett, Benjamin Van Durme, Olivia Deng, Akanksha Malhotra, and Bill Dolan. Craft an iron sword: Dynamically generating interactive game characters by prompting large language models tuned on code. In Proceedings of the 3rd Wordplay: When Language Meets Games Workshop (Wordplay 2022) , pages 25–43, Seattle, United States, 2022. Association for Computational Linguistics.
- [71] Haoqi Yuan, Chi Zhang, Hongcheng Wang, Feiyang Xie, Penglin Cai, Hao Dong, and Zongqing Lu. Plan4mc: Skill reinforcement learning and planning for open-world minecraft tasks. arXiv preprint arXiv: 2303.16563 , 2023.
- [72] Rishi Bommasani, Drew A. Hudson, Ehsan Adeli, Russ Altman, Simran Arora, Sydney von Arx, Michael S. Bernstein, Jeannette Bohg, Antoine Bosselut, Emma Brunskill, Erik Brynjolfsson, Shyamal Buch, Dallas Card, Rodrigo Castellon, Niladri Chatterji, Annie Chen, Kathleen Creel, Jared Quincy Davis, Dora Demszky, Chris Donahue, Moussa Doumbouya, Esin Durmus, Stefano Ermon, John Etchemendy, Kawin Ethayarajh, Li Fei-Fei, Chelsea Finn, Trevor Gale, Lauren Gillespie, Karan Goel, Noah Goodman, Shelby Grossman, Neel Guha, Tatsunori Hashimoto, Peter Henderson, John Hewitt, Daniel E. Ho, Jenny Hong, Kyle Hsu, Jing Huang, Thomas Icard, Saahil Jain, Dan Jurafsky, Pratyusha Kalluri, Siddharth Karamcheti, Geoff Keeling, Fereshte Khani, Omar Khattab, Pang Wei Koh, Mark Krass, Ranjay Krishna, Rohith Kuditipudi, Ananya Kumar, Faisal Ladhak, Mina Lee, Tony Lee, Jure Leskovec, Isabelle Levent, Xiang Lisa Li, Xuechen Li, Tengyu Ma, Ali Malik, Christopher D. Manning, Suvir Mirchandani, Eric Mitchell, Zanele Munyikwa, Suraj Nair, Avanika Narayan, Deepak Narayanan, Ben Newman, Allen Nie, Juan Carlos Niebles, Hamed Nilforoshan, Julian Nyarko, Giray Ogut, Laurel Orr, Isabel Papadimitriou, Joon Sung Park, Chris Piech, Eva Portelance, Christopher Potts, Aditi Raghunathan, Rob Reich, Hongyu Ren, Frieda Rong, Yusuf Roohani, Camilo Ruiz, Jack Ryan, Christopher Ré, Dorsa Sadigh, Shiori Sagawa, Keshav Santhanam, Andy Shih, Krishnan Srinivasan, Alex Tamkin, Rohan Taori, Armin W. Thomas, Florian Tramèr, Rose E. Wang, William Wang, Bohan Wu, Jiajun Wu, Yuhuai Wu, Sang Michael Xie, Michihiro Yasunaga, Jiaxuan You, Matei Zaharia, Michael Zhang, Tianyi Zhang, Xikun Zhang, Yuhui Zhang, Lucia Zheng, Kaitlyn Zhou, and Percy Liang. On the opportunities and risks of foundation models. arXiv preprint arXiv: Arxiv-2108.07258 , 2021.
- [73] Aakanksha Chowdhery, Sharan Narang, Jacob Devlin, Maarten Bosma, Gaurav Mishra, Adam Roberts, Paul Barham, Hyung Won Chung, Charles Sutton, Sebastian Gehrmann, Parker Schuh, Kensen Shi, Sasha Tsvyashchenko, Joshua Maynez, Abhishek Rao, Parker Barnes, Yi Tay, Noam Shazeer, Vinodkumar Prabhakaran, Emily Reif, Nan Du, Ben Hutchinson, Reiner Pope, James Bradbury, Jacob Austin, Michael Isard, Guy Gur-Ari, Pengcheng Yin, Toju Duke, Anselm Levskaya, Sanjay Ghemawat, Sunipa Dev, Henryk Michalewski, Xavier Garcia, Vedant Misra, Kevin Robinson, Liam Fedus, Denny Zhou, Daphne Ippolito, David Luan, Hyeontaek Lim, Barret Zoph, Alexander Spiridonov, Ryan Sepassi, David Dohan, Shivani Agrawal, Mark Omernick, Andrew M. Dai, Thanumalayan Sankaranarayana Pillai, Marie Pellat, Aitor Lewkowycz, Erica Moreira, Rewon Child, Oleksandr Polozov, Katherine Lee, Zongwei Zhou, Xuezhi Wang, Brennan Saeta, Mark Diaz, Orhan Firat, Michele Catasta, Jason Wei, Kathy Meier-Hellstern, Douglas Eck, Jeff Dean, Slav Petrov, and Noah Fiedel. Palm: Scaling language modeling with pathways. arXiv preprint arXiv: Arxiv-2204.02311 , 2022.
- [74] Hyung Won Chung, Le Hou, Shayne Longpre, Barret Zoph, Yi Tay, William Fedus, Eric Li, Xuezhi Wang, Mostafa Dehghani, Siddhartha Brahma, Albert Webson, Shixiang Shane Gu, Zhuyun Dai, Mirac Suzgun, Xinyun Chen, Aakanksha Chowdhery, Sharan Narang, Gaurav Mishra, Adams Yu, Vincent Zhao, Yanping Huang, Andrew Dai, Hongkun Yu, Slav Petrov, Ed H. Chi, Jeff Dean, Jacob Devlin, Adam Roberts, Denny Zhou, Quoc V. Le, and Jason Wei. Scaling instruction-finetuned language models. arXiv preprint arXiv: Arxiv-2210.11416 , 2022.
- [75] Jiafei Duan, Samson Yu, Hui Li Tan, Hongyuan Zhu, and Cheston Tan. A survey of embodied AI: from simulators to research tasks. IEEE Trans. Emerg. Top. Comput. Intell. , 6(2):230–244, 2022.
- [76] Dhruv Batra, Angel X. Chang, Sonia Chernova, Andrew J. Davison, Jia Deng, Vladlen Koltun, Sergey Levine, Jitendra Malik, Igor Mordatch, Roozbeh Mottaghi, Manolis Savva, and Hao Su. Rearrangement: A challenge for embodied ai. arXiv preprint arXiv: Arxiv-2011.01975 , 2020.
- [77] Harish Ravichandar, Athanasios S Polydoros, Sonia Chernova, and Aude Billard. Recent advances in robot learning from demonstration. Annual review of control, robotics, and autonomous systems , 3:297–330, 2020.
- [78] Jack Collins, Shelvin Chand, Anthony Vanderkop, and David Howard. A review of physics simulators for robotic applications. IEEE Access , 9:51416–51431, 2021.
- [79] So Yeon Min, Devendra Singh Chaplot, Pradeep Ravikumar, Yonatan Bisk, and R. Salakhutdinov. Film: Following instructions in language with modular methods. International Conference on Learning Representations , 2021.
- [80] Valts Blukis, Chris Paxton, Dieter Fox, Animesh Garg, and Yoav Artzi. A persistent spatial semantic representation for high-level natural language instruction execution. In 5th Annual Conference on Robot Learning , 2021.
- [81] Varun Nair, Elliot Schumacher, Geoffrey Tso, and Anitha Kannan. Dera: Enhancing large language model completions with dialog-enabled resolving agents. arXiv preprint arXiv: Arxiv-2303.17071 , 2023.
- [82] Joon Sung Park, Joseph C. O’Brien, Carrie J. Cai, Meredith Ringel Morris, Percy Liang, and Michael S. Bernstein. Generative agents: Interactive simulacra of human behavior. arXiv preprint arXiv: Arxiv-2304.03442 , 2023.
- [83] Yue Wu, Shrimai Prabhumoye, So Yeon Min, Yonatan Bisk, Ruslan Salakhutdinov, Amos Azaria, Tom Mitchell, and Yuanzhi Li. Spring: Gpt-4 out-performs rl algorithms by studying papers and reasoning. arXiv preprint arXiv: 2305.15486 , 2023.
- [84] Erik Nijkamp, Bo Pang, Hiroaki Hayashi, Lifu Tu, Huan Wang, Yingbo Zhou, Silvio Savarese, and Caiming Xiong. A conversational paradigm for program synthesis. arXiv preprint arXiv: Arxiv-2203.13474 , 2022.
- [85] Hung Le, Yue Wang, Akhilesh Deepak Gotmare, Silvio Savarese, and Steven C. H. Hoi. Coderl: Mastering code generation through pretrained models and deep reinforcement learning. arXiv preprint arXiv: Arxiv-2207.01780 , 2022.
- [86] Xinyun Chen, Chang Liu, and Dawn Song. Execution-guided neural program synthesis. In 7th International Conference on Learning Representations, ICLR 2019, New Orleans, LA, USA, May 6-9, 2019 . OpenReview.net, 2019.
- [87] Xinyun Chen, Dawn Song, and Yuandong Tian. Latent execution for neural program synthesis. arXiv preprint arXiv: Arxiv-2107.00101 , 2021.
- [88] Kevin Ellis, Maxwell I. Nye, Yewen Pu, Felix Sosa, Josh Tenenbaum, and Armando Solar-Lezama. Write, execute, assess: Program synthesis with a REPL. In Hanna M. Wallach, Hugo Larochelle, Alina Beygelzimer, Florence d’Alché-Buc, Emily B. Fox, and Roman Garnett, editors, Advances in Neural Information Processing Systems 32: Annual Conference on Neural Information Processing Systems 2019, NeurIPS 2019, December 8-14, 2019, Vancouver, BC, Canada , pages 9165–9174, 2019.
- [89] Yujia Li, David Choi, Junyoung Chung, Nate Kushman, Julian Schrittwieser, Rémi Leblond, Tom Eccles, James Keeling, Felix Gimeno, Agustin Dal Lago, Thomas Hubert, Peter Choy, Cyprien de Masson d’Autume, Igor Babuschkin, Xinyun Chen, Po-Sen Huang, Johannes Welbl, Sven Gowal, Alexey Cherepanov, James Molloy, Daniel J. Mankowitz, Esme Sutherland Robson, Pushmeet Kohli, Nando de Freitas, Koray Kavukcuoglu, and Oriol Vinyals. Competition-level code generation with alphacode. arXiv preprint arXiv: Arxiv-2203.07814 , 2022.
- [90] Karl Cobbe, Vineet Kosaraju, Mohammad Bavarian, Mark Chen, Heewoo Jun, Lukasz Kaiser, Matthias Plappert, Jerry Tworek, Jacob Hilton, Reiichiro Nakano, Christopher Hesse, and John Schulman. Training verifiers to solve math word problems. arXiv preprint arXiv: Arxiv-2110.14168 , 2021.
- [91] Ansong Ni, Srini Iyer, Dragomir Radev, Ves Stoyanov, Wen tau Yih, Sida I. Wang, and Xi Victoria Lin. Lever: Learning to verify language-to-code generation with execution. arXiv preprint arXiv: Arxiv-2302.08468 , 2023.
- [92] Marta Skreta, Naruki Yoshikawa, Sebastian Arellano-Rubach, Zhi Ji, Lasse Bjørn Kristensen, Kourosh Darvish, Alán Aspuru-Guzik, Florian Shkurti, and Animesh Garg. Errors are useful prompts: Instruction guided task programming with verifier-assisted iterative prompting. arXiv preprint arXiv: Arxiv-2303.14100 , 2023.
Appendix A Method
A.1 voyager algorithm, a.2 prompting.
GPT-4 and GPT-3.5 offer users the ability to designate the role of each prompt message among three options:
System: A high-level instruction that guides the model behavior throughout the conversation. It sets the overall tone and objective for the interaction.
User: A detailed instruction that guides the assistant for the next immediate response.
Assistant: A response message generated the model.
See https://platform.openai.com/docs/guides/chat/introduction for more details.
To save token usage, instead of engaging in multi-round conversations, we concatenate a system prompt and a user prompt to obtain each assistant’s response.
A.3 Automatic Curriculum
A.3.1 components in the prompt.
Directives encouraging diverse behaviors and imposing constraints (so that the proposed task is achievable and verifiable): See Sec. A.3.4 for the full prompt;
The agent’s current state:
Inventory : A dictionary of items with counts, for example, {‘cobblestone’: 4, ‘furnace’: 1, ‘stone_pickaxe’: 1, ‘oak_planks’: 7, ‘dirt’: 6, ‘wooden_pickaxe’: 1, ‘crafting_table’: 1, ‘raw_iron’: 4, ‘coal’: 1};
Equipment : Armors or weapons equipped by the agents;
Nearby blocks : A set of block names within a 32-block distance to the agent, for example, ‘dirt’, ‘water’, ‘spruce_planks’, ‘grass_block’, ‘dirt_path’, ‘sugar_cane’, ‘fern’;
Other blocks that are recently seen : Blocks that are not nearby or in the inventory;
Nearby entities : A set of entity names within a 32-block distance to the agent, for example, ‘pig’, ‘cat’, ‘villager’, ‘zombie’;
A list of chests that are seen by the agent : Chests are external containers where the agent can deposit items. If a chest is not opened before, its content is “Unknown”. Otherwise, the items inside each chest are shown to the agent.
Biome : For example, ‘plains’, ‘flower_forest’, ‘meadow’, ‘river’, ‘beach’, ‘forest’, ‘snowy_slopes’, ‘frozen_peaks’, ‘old_growth_birch_forest’, ‘ocean’, ‘sunflower_plains’, ‘stony_shore’;
Time : One of ‘sunrise’, ‘day’, ‘noon’, ‘sunset’, ‘night’, ‘midnight’;
Health and hunger bars : The max value is 20;
Position : 3D coordinate ( x , y , z ) 𝑥 𝑦 𝑧 (x,y,z) of the agent’s position in the Minecraft world;
Previously completed and failed tasks;
Additional context: See Sec. A.3.2 ;
Chain-of-thought prompting [ 46 ] in response: We request GPT-4 to first reason about the current progress and then suggest the next task.
A.3.2 Additional Context
We leverage GPT-3.5 to self-ask questions to provide additional context. Each question is paired with a concept that is used for retrieving the most relevant document from the wiki knowledge base [ 23 ] . We feed the document content to GPT-3.5 for self-answering questions. In practice, using a wiki knowledge base is optional since GPT-3.5 already possesses a good understanding of Minecraft game mechanics. However, the external knowledge base becomes advantageous if GPT-3.5 is not pre-trained in that specific domain. See Sec. A.3.4 for the full prompt.
A.3.3 Warm-up Schedule
In practice, we adopt a warm-up schedule to gradually incorporate the agent’s state and the additional context into the prompt based on how many tasks the agent has completed. This ensures that the prompt is exposed to increasing amounts of information over the exploration progress and therefore begins with basic skills and progressively advances towards more intricate and diverse ones. The warm-up setting that we use across all the experiments is shown in Table. A.1 .
A.3.4 Full Prompt
A.4 skill library, a.4.1 components in the prompt.
Guidelines for code generation: See Sec A.4.2 for the full prompt;
Control primitive APIs implemented by us: These APIs serve a dual purpose: they demonstrate the usage of Mineflayer APIs, and they can be directly called by GPT-4.
exploreUntil(bot, direction, maxTime = 60, callback) : Allow the agent to explore in a fixed direction for maxTime . The callback is the stopping condition implemented by the agent to determine when to stop exploring;
mineBlock(bot, name, count = 1) : Mine and collect the specified number of blocks within a 32-block distance;
craftItem(bot, name, count = 1) : Craft the item with a crafting table nearby;
placeItem(bot, name, position) : Place the block at the specified position;
smeltItem(bot, itemName, fuelName, count = 1) : Smelt the item with the specified fuel. There must be a furnace nearby;
killMob(bot, mobName, timeout = 300) : Attack the mob and collect its dropped item;
getItemFromChest(bot, chestPosition, itemsToGet) : Move to the chest at the specified position and get items from the chest;
depositItemIntoChest(bot, chestPosition, itemsToDeposit) : Move to the chest at the specified position and deposit items into the chest;
Control primitive APIs provided by Mineflayer:
await bot.pathfinder.goto(goal) : Go to a specific position. See below for how to set the goal;
new GoalNear(x, y, z, range) : Move the bot to a block within the specified range of the specified block;
new GoalXZ(x, z) : For long-range goals that don’t have a specific Y level;
new GoalGetToBlock(x, y, z) : Not get into the block, but get directly adjacent to it. Useful for fishing, farming, filling a bucket, and using a bed.;
new GoalFollow(entity, range) : Follow the specified entity within the specified range;
new GoalPlaceBlock(position, bot.world, {}) : Position the bot in order to place a block;
new GoalLookAtBlock(position, bot.world, {}) : Path towards a position where a face of the block at position is visible;
bot.isABed(bedBlock) : Return true if bedBlock is a bed;
bot.blockAt(position) : Return the block at position ;
await bot.equip(item, destination) : Equip the item in the specified destination. destination must be one of “hand”, “head”, “torso”, “legs”, “feet”, “off-hand”;
await bot.consume() : Consume the item in the bot’s hand. You must equip the item to consume first. Useful for eating food, drinking potions, etc.;
await bot.fish() : Let bot fish. Before calling this function, you must first get to a water block and then equip a fishing rod. The bot will automatically stop fishing when it catches a fish;
await bot.sleep(bedBlock) : Sleep until sunrise. You must get to a bed block first;
await bot.activateBlock(block) : This is the same as right-clicking a block in the game. Useful for buttons, doors, etc. You must get to the block first;
await bot.lookAt(position) : Look at the specified position. You must go near the position before you look at it. To fill a bucket with water, you must look at it first;
await bot.activateItem() : This is the same as right-clicking to use the item in the bot’s hand. Useful for using a bucket, etc. You must equip the item to activate first;
await bot.useOn(entity) : This is the same as right-clicking an entity in the game. Useful for shearing a sheep. You must get to the entity first;
Retrieved skills from the skill library;
Generated code from the last round;
Environment feedback: The chat log in the prompt;
Execution errors;
Critique from the self-verification module;
The agent’s current state: See Sec. A.3.1 for each element of the agent’s state;
Task proposed by the automatic curriculum;
Task context: We prompt GPT-3.5 to ask for general suggestions about how to solve the task. In practice, this part is handled by the automatic curriculum since it has a systematic mechanism for question-answering (Sec. A.3.2 );
Chain-of-thought prompting [ 46 ] in response: We ask GPT-4 to first explain the reason why the code from the last round fails, then give step-by-step plans to finish the task, and finally generate code. See Sec. A.4.2 for the full prompt.
A.4.2 Full Prompt
A.4.3 examples, a.5 self-verification, a.5.1 components in the prompt.
The agent’s state: We exclude other blocks that are recently seen and nearby entities from the agent’s state since they are not useful for assessing the task’s completeness. See Sec. A.3.1 for each element of the agent’s state;
Chain-of-thought prompting [ 46 ] in response: We request GPT-4 to initially reason about the task’s success or failure, then output a boolean variable indicating the task’s outcome, and finally provide a critique to the agent if the task fails.
Few-shot examples for in-context learning [ 36 , 37 , 38 ] .
A.5.2 Full Prompt
A.6 system-level comparison between voyager and prior works.
We make a system-level comparison in Table. A.2 . Voyager stands out as the only method featuring a combination of automatic curriculum, iterative planning, and a skill library. Moreover, it learns to play Minecraft without the need for any gradient update.
Appendix B Experiments
B.1 experimental setup.
Our simulation environment is built upon MineDojo [ 23 ] and utilizes Mineflayer [ 52 ] JavaScript APIs for motor controls (Sec. A.4.2 ). Additionally, we incorporate many bot.chat() into Mineflayer functions to provide abundant environment feedback and implement various condition checks along with try-catch exceptions for continuous execution. If the bot dies, it is resurrected near the closest ground, and its inventory is preserved for uninterrupted exploration. The bot recycles its crafting table and furnace after program execution. For detailed implementations, please refer to our codebase.
B.2 Baselines
ReAct [ 29 ] uses chain-of-thought prompting [ 46 ] by generating both reasoning traces and action plans with LLMs. We provide it with our environment feedback and the agent states as observations. ReAct undergoes one round of code generation from scratch, followed by three rounds of code refinement. This process is then repeated until the maximum prompting iteration is reached.
Reflexion [ 30 ] is built on top of ReAct [ 29 ] with self-reflection to infer more intuitive future actions. We provide it with environment feedback, the agent states, execution errors, and our self-verification module. Similar to ReAct, Reflexion undergoes one round of code generation from scratch, followed by three rounds of code refinement. This process is then repeated until the maximum prompting iteration is reached.
AutoGPT [ 28 ] is a popular software tool that automates NLP tasks by decomposing a high-level goal into multiple subgoals and executing them in a ReAct-style loop. We re-implement AutoGPT by using GPT-4 to do task decomposition and provide it with the agent states, environment feedback, and execution errors as observations for subgoal execution. Compared with Voyager , AutoGPT lacks the skill library for accumulating knowledge, self-verification for assessing task success, and automatic curriculum for open-ended exploration. During each subgoal execution, if no execution error occurs, we consider the subgoal completed and proceed to the next one. Otherwise, we refine the program until three rounds of code refinement (equivalent to four rounds of code generation) are completed, and then move on to the next subgoal. If three consecutive subgoals do not result in acquiring a new item, we replan by rerunning the task decomposition.
The task is “explore the world and get as many items as possible” for all baselines.
B.3 Ablations
We ablate 6 design choices (automatic curriculum, skill library, environment feedback, execution errors, self-verification, and GPT-4 for code generation) in Voyager and study their impact on exploration performance.
Manual Curriculum : We substitute the automatic curriculum with a manually designed curriculum for mining a diamond: “Mine 3 wood log”, “Craft 1 crafting table”, “Craft 1 wooden pickaxe”, “Mine 11 cobblestone”, “Craft 1 stone pickaxe”, “Craft 1 furnace”, “Mine 3 iron ore”, “Smelt 3 iron ore”, “Craft 1 iron pickaxe”, “Mine 1 diamond”. A manual curriculum requires human effort to design and is not scalable for open-ended exploration.
Random Curriculum : We curate 101 items obtained by Voyager and create a random curriculum by randomly selecting one item as the next task.
w/o Skill Library : We remove the skill library, eliminating skill retrieval for code generation.
w/o Environment Feedback : We exclude environment feedback (chat log) from the prompt for code generation.
w/o Execution Errors : We exclude execution errors from the prompt for code generation.
w/o Self-Verification : For each task, we generate code without self-verification and iteratively refine the program for 3 rounds (equivalent to 4 rounds of code generation in total).
GPT-3.5 : We replace GPT-4 with GPT-3.5 for code generation. We retain GPT-4 for the automatic curriculum and the self-verification module.
B.4 Evaluation Results
B.4.1 significantly better exploration.
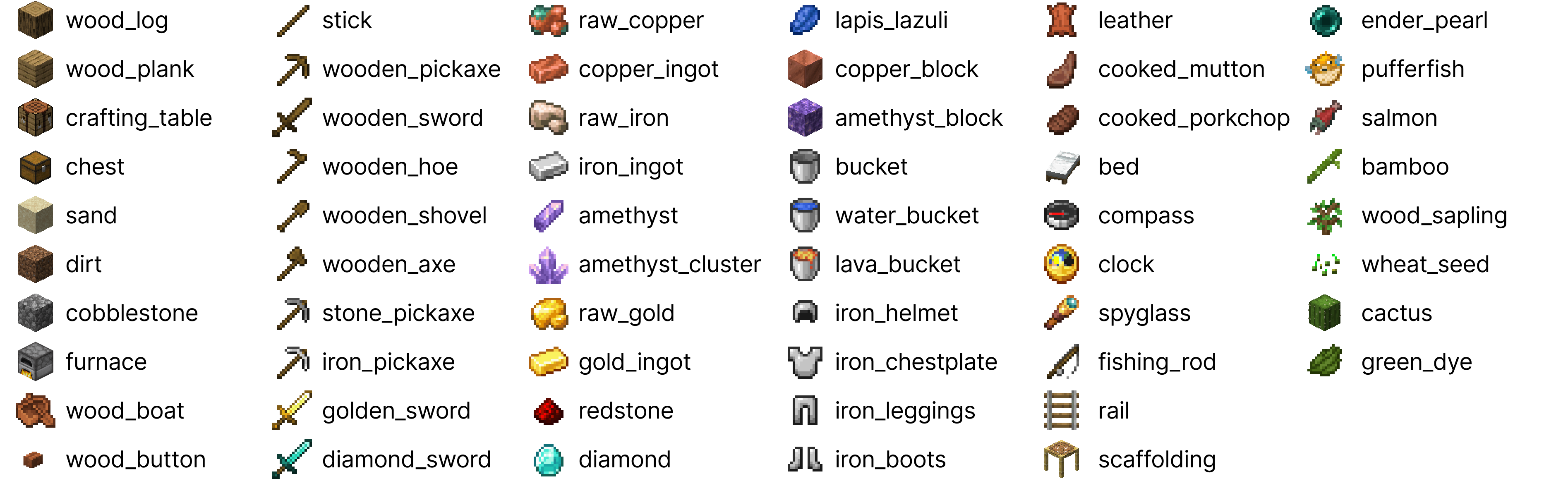
The meaning of each icon in Fig. 1 is shown in Fig. A.1 .
We run three trials for each method. The items collected by Voyager in each trial is
Trial 1 : ‘iron_ingot’, ‘stone_shovel’, ‘iron_leggings’, ‘fishing_rod’, ‘pufferfish’, ‘oak_log’, ‘cooked_mutton’, ‘green_dye’, ‘flint’, ‘chest’, ‘iron_sword’, ‘string’, ‘ender_pearl’, ‘raw_copper’, ‘crafting_table’, ‘cactus’, ‘lapis_lazuli’, ‘iron_pickaxe’, ‘copper_ingot’, ‘stone_pickaxe’, ‘wooden_hoe’, ‘scaffolding’, ‘stick’, ‘porkchop’, ‘copper_block’, ‘gravel’, ‘grass_block’, ‘white_bed’, ‘bone’, ‘dirt’, ‘mutton’, ‘white_wool’, ‘oak_sapling’, ‘coal’, ‘bamboo’, ‘wooden_pickaxe’, ‘rotten_flesh’, ‘cooked_porkchop’, ‘cod’, ‘iron_boots’, ‘lightning_rod’, ‘diorite’, ‘water_bucket’, ‘shears’, ‘furnace’, ‘andesite’, ‘granite’, ‘bucket’, ‘wooden_sword’, ‘sandstone’, ‘iron_helmet’, ‘raw_iron’, ‘sand’, ‘acacia_log’, ‘cooked_cod’, ‘oak_planks’, ‘azure_bluet’, ‘iron_shovel’, ‘acacia_planks’, ‘shield’, ‘iron_axe’, ‘iron_chestplate’, ‘cobblestone’;
Trial 2 : ‘iron_ingot’, ‘tuff’, ‘stone_shovel’, ‘iron_leggings’, ‘fishing_rod’, ‘cooked_mutton’, ‘spruce_planks’, ‘gunpowder’, ‘amethyst_shard’, ‘chest’, ‘string’, ‘cooked_salmon’, ‘iron_sword’, ‘raw_copper’, ‘crafting_table’, ‘torch’, ‘lapis_lazuli’, ‘iron_pickaxe’, ‘copper_ingot’, ‘stone_pickaxe’, ‘wooden_hoe’, ‘stick’, ‘amethyst_block’, ‘salmon’, ‘calcite’, ‘gravel’, ‘white_bed’, ‘bone’, ‘dirt’, ‘mutton’, ‘white_wool’, ‘spyglass’, ‘coal’, ‘wooden_pickaxe’, ‘cod’, ‘iron_boots’, ‘lily_pad’, ‘cobbled_deepslate’, ‘lightning_rod’, ‘snowball’, ‘stone_axe’, ‘smooth_basalt’, ‘diorite’, ‘water_bucket’, ‘furnace’, ‘andesite’, ‘bucket’, ‘granite’, ‘shield’, ‘iron_helmet’, ‘raw_iron’, ‘cobblestone’, ‘spruce_log’, ‘cooked_cod’, ‘tripwire_hook’, ‘stone_hoe’, ‘iron_chestplate’, ‘stone_sword’;
Trial 3 : ‘spruce_planks’, ‘dirt’, ‘shield’, ‘redstone’, ‘clock’, ‘diamond_sword’, ‘iron_chestplate’, ‘stone_pickaxe’, ‘leather’, ‘string’, ‘chicken’, ‘chest’, ‘diorite’, ‘iron_leggings’, ‘black_wool’, ‘cobblestone_wall’, ‘cobblestone’, ‘cooked_chicken’, ‘feather’, ‘stone_sword’, ‘raw_gold’, ‘gravel’, ‘birch_planks’, ‘coal’, ‘cobbled_deepslate’, ‘oak_planks’, ‘iron_pickaxe’, ‘granite’, ‘tuff’, ‘crafting_table’, ‘iron_helmet’, ‘stone_hoe’, ‘iron_ingot’, ‘stone_axe’, ‘birch_boat’, ‘stick’, ‘sand’, ‘bone’, ‘raw_iron’, ‘beef’, ‘rail’, ‘oak_sapling’, ‘kelp’, ‘gold_ingot’, ‘birch_log’, ‘wheat_seeds’, ‘cooked_mutton’, ‘furnace’, ‘arrow’, ‘stone_shovel’, ‘white_wool’, ‘andesite’, ‘jungle_slab’, ‘mutton’, ‘iron_sword’, ‘copper_ingot’, ‘diamond’, ‘torch’, ‘oak_log’, ‘cooked_beef’, ‘copper_block’, ‘flint’, ‘bone_meal’, ‘raw_copper’, ‘wooden_pickaxe’, ‘iron_boots’, ‘wooden_sword’.
The items collected by ReAct [ 29 ] in each trial is
Trial 1 : ‘bamboo’, ‘dirt’, ‘sand’, ‘wheat_seeds’;
Trial 2 : ‘dirt’, ‘rabbit’, ‘spruce_log’, ‘spruce_sapling’;
Trial 3 : ‘dirt’, ‘pointed_dripstone’;
The items collected by Reflexion [ 30 ] in each trial is
Trial 1 : ‘crafting_table’, ‘orange_tulip’, ‘oak_planks’, ‘oak_log’, ‘dirt’;
Trial 2 : ‘spruce_log’, ‘dirt’, ‘clay_ball’, ‘sand’, ‘gravel’;
Trial 3 : ‘wheat_seeds’, ‘oak_log’, ‘dirt’, ‘birch_log’, ‘sand’.
The items collected by AutoGPT [ 28 ] in each trial is
Trial 1 : ‘feather’, ‘oak_log’, ‘leather’, ‘stick’, ‘porkchop’, ‘chicken’, ‘crafting_table’, ‘wheat_seeds’, ‘oak_planks’, ‘dirt’, ‘mutton’;
Trial 2 : ‘wooden_pickaxe’, ‘iron_ingot’, ‘stone’, ‘coal’, ‘spruce_planks’, ‘string’, ‘raw_copper’, ‘crafting_table’, ‘diorite’, ‘andesite’, ‘furnace’, ‘torch’, ‘spruce_sapling’, ‘granite’, ‘iron_pickaxe’, ‘stone_pickaxe’, ‘wooden_axe’, ‘raw_iron’, ‘stick’, ‘spruce_log’, ‘dirt’, ‘cobblestone’;
Trial 3 : ‘wooden_shovel’, ‘wooden_pickaxe’, ‘iron_ingot’, ‘stone’, ‘cod’, ‘coal’, ‘oak_log’, ‘flint’, ‘raw_copper’, ‘crafting_table’, ‘diorite’, ‘furnace’, ‘andesite’, ‘torch’, ‘granite’, ‘lapis_lazuli’, ‘iron_pickaxe’, ‘stone_pickaxe’, ‘raw_iron’, ‘stick’, ‘gravel’, ‘oak_planks’, ‘dirt’, ‘iron_axe’, ‘cobblestone’.
B.4.2 Extensive Map Traversal
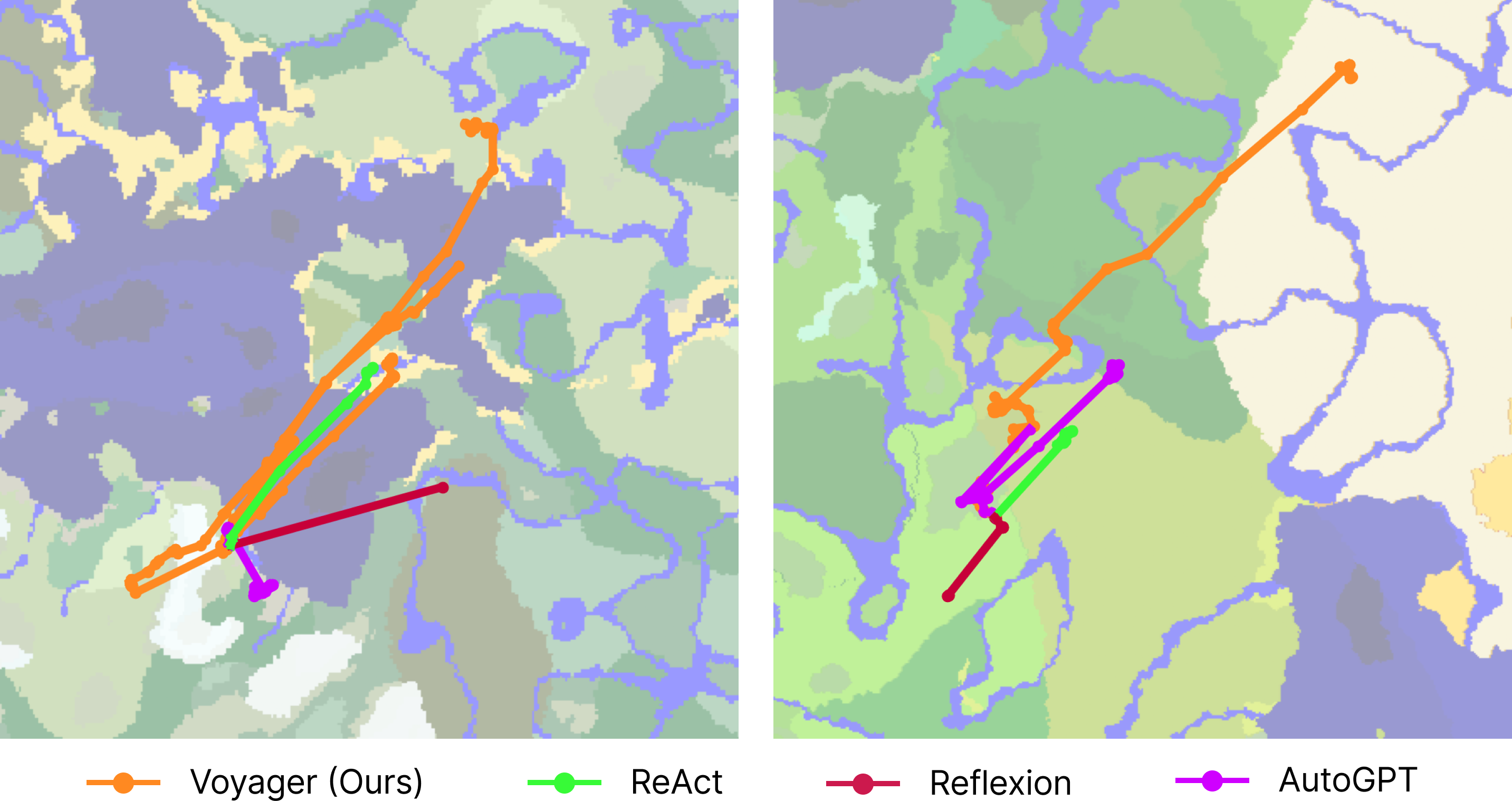
Agent trajectories for map coverage are displayed in Fig. A.2 . Fig. 7 is plotted based on Fig. A.2 by drawing the smallest circle enclosing each trajectory. The terrains traversed by Voyager in each trial is
Trial 1 : ‘meadow’, ‘desert’, ‘river’, ‘savanna’, ‘forest’, ‘plains’, ‘bamboo_jungle’, ‘dripstone_caves’;
Trial 2 : ‘snowy_plains’, ‘frozen_river’, ‘dripstone_caves’, ‘snowy_taiga’, ‘beach’;
Trial 3 : ‘flower_forest’, ‘meadow’, ‘old_growth_birch_forest’, ‘snowy_slopes’, ‘frozen_peaks’, ‘forest’, ‘river’, ‘beach’, ‘ocean’, ‘sunflower_plains’, ‘plains’, ‘stony_shore’.
The terrains traversed by ReAct [ 29 ] in each trial is
Trial 1 : ‘plains’, ‘desert’, ‘jungle’;
Trial 2 : ‘snowy_plains’, ‘snowy_taiga’, ‘snowy_slopes’;
Trial 3 : ‘dark_forest’, ‘dripstone_caves’, ‘grove’, ‘jagged_peaks’.
The terrains traversed by Reflexion [ 30 ] in each trial is
Trial 1 : ‘plains’, ‘flower_forest’;
Trial 2 : ‘snowy_taiga’;
Trial 3 : ‘old_growth_birch_forest’, ‘river’, ‘ocean’, ‘beach’, ‘plains’.
The terrains traversed by AutoGPT [ 28 ] in each trial is
Trial 1 : ‘plains’, ‘dripstone_caves’, ‘savanna’, ‘meadow’;
Trial 3 : ‘plains’, ‘stony_shore’, ‘forest’, ‘ocean’.
B.4.3 Efficient Zero-Shot Generalization to Unseen Tasks
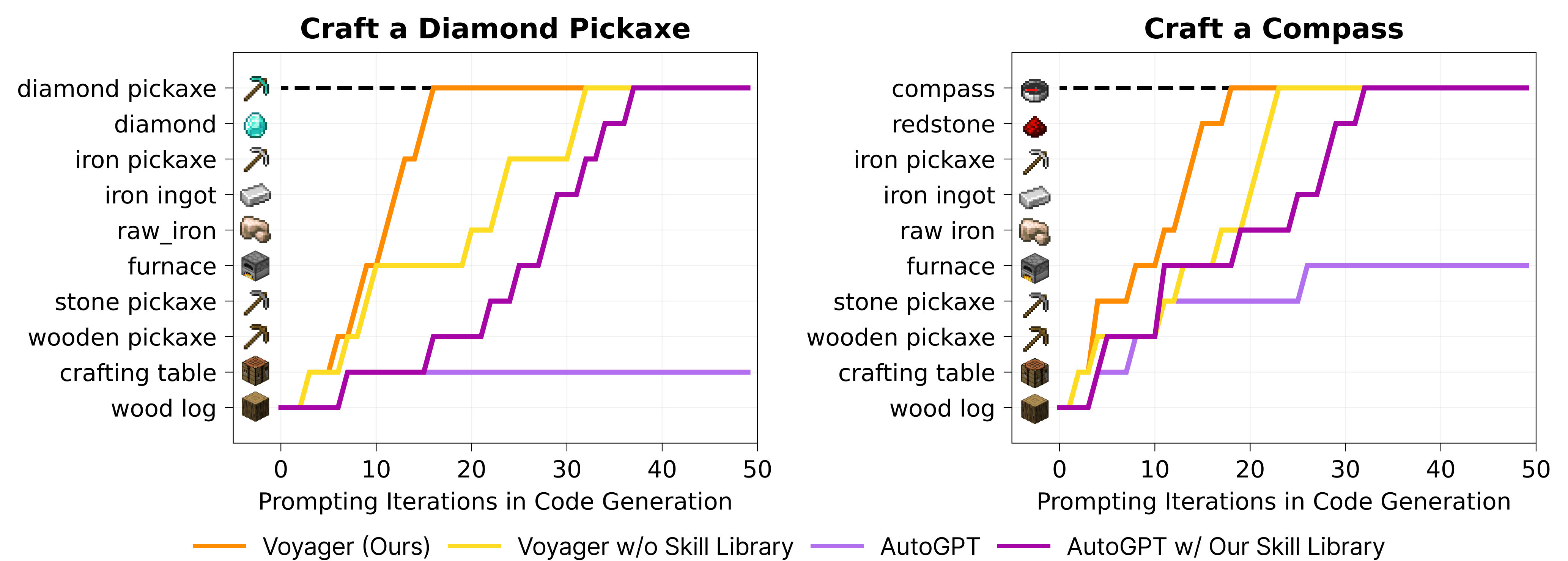
The results of zero-shot generalization to unseen tasks for the other two tasks are presented in Fig. A.3 . Similar to Fig. 8 , Voyager consistently solves all tasks, while the baselines are unable to solve any task within 50 prompting iterations. Our skill library, constructed from lifelong learning, not only enhances Voyager ’s performance but also provides a boost to AutoGPT [ 28 ] .
B.4.4 Accurate Skill Retrieval
We conduct an evaluation of our skill retrieval (309 samples in total) and the results are in Table. A.4 . The top-5 accuracy standing at 96.5% suggests our retrieval process is reliable (note that we include the top-5 relevant skills in the prompt for synthesizing a new skill).
B.4.5 Robust to Model Variations
In the main paper, all of Voyager’s experiments are conducted with gpt-4-0314 . We additionally run new experiments with gpt-4-0613 and find that the performance is roughly the same (Fig. A.4 ). It demonstrates that Voyager is robust to model variations.
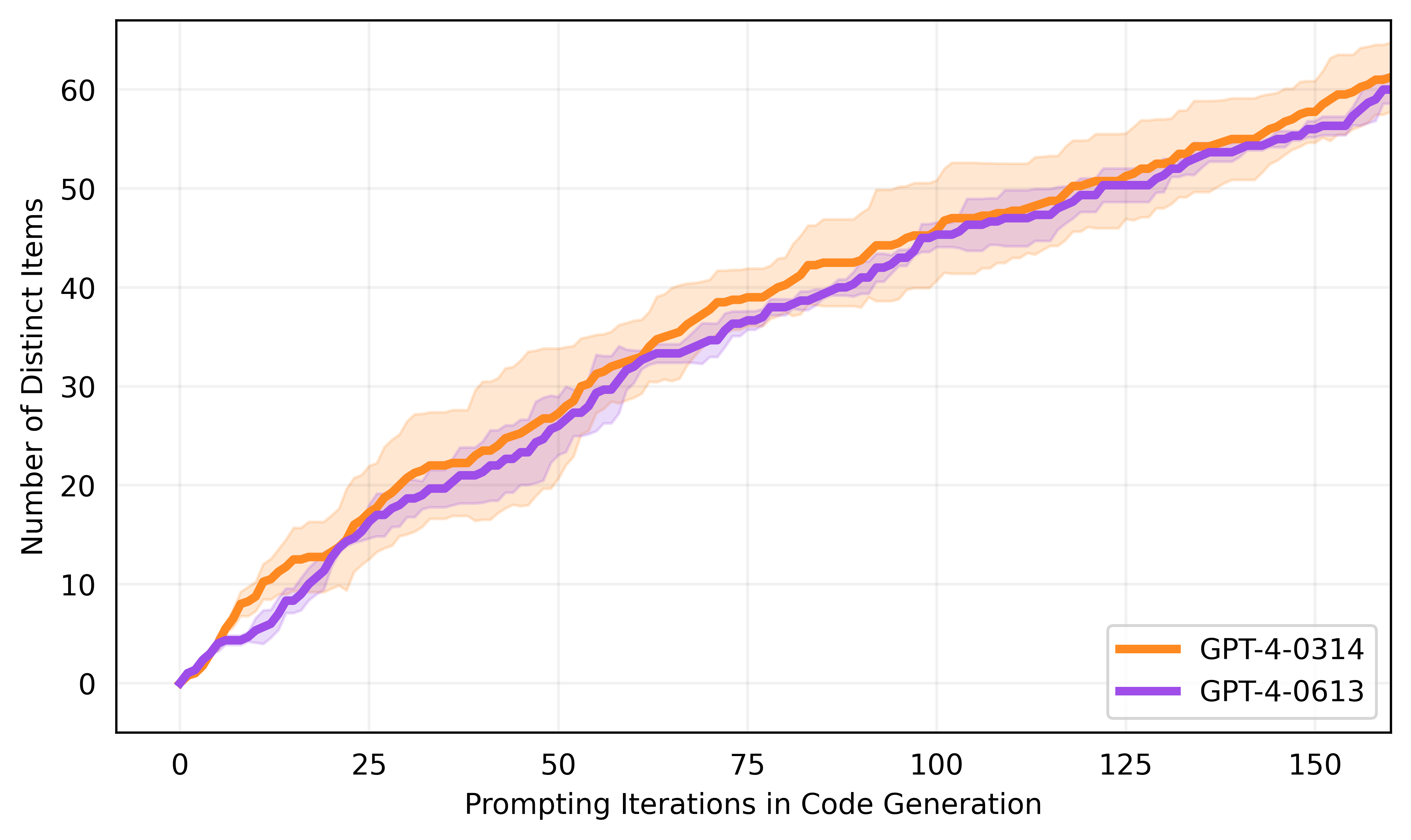
- Certification
- Modern Steel
- Architecture
has been added to your cart!
Quiz: has been added to your profile!
Your cart has been updated!
The coupon code has been applied to your cart!
The coupon code has been removed from your cart!
is not a valid coupon code!
Only one coupon is allowed per order!
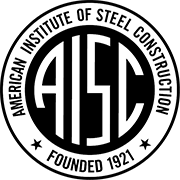
- Awards and Competitions
- IDEAS² Awards
- IDEAS² Awards Archives
NVIDIA Phase II - Voyager
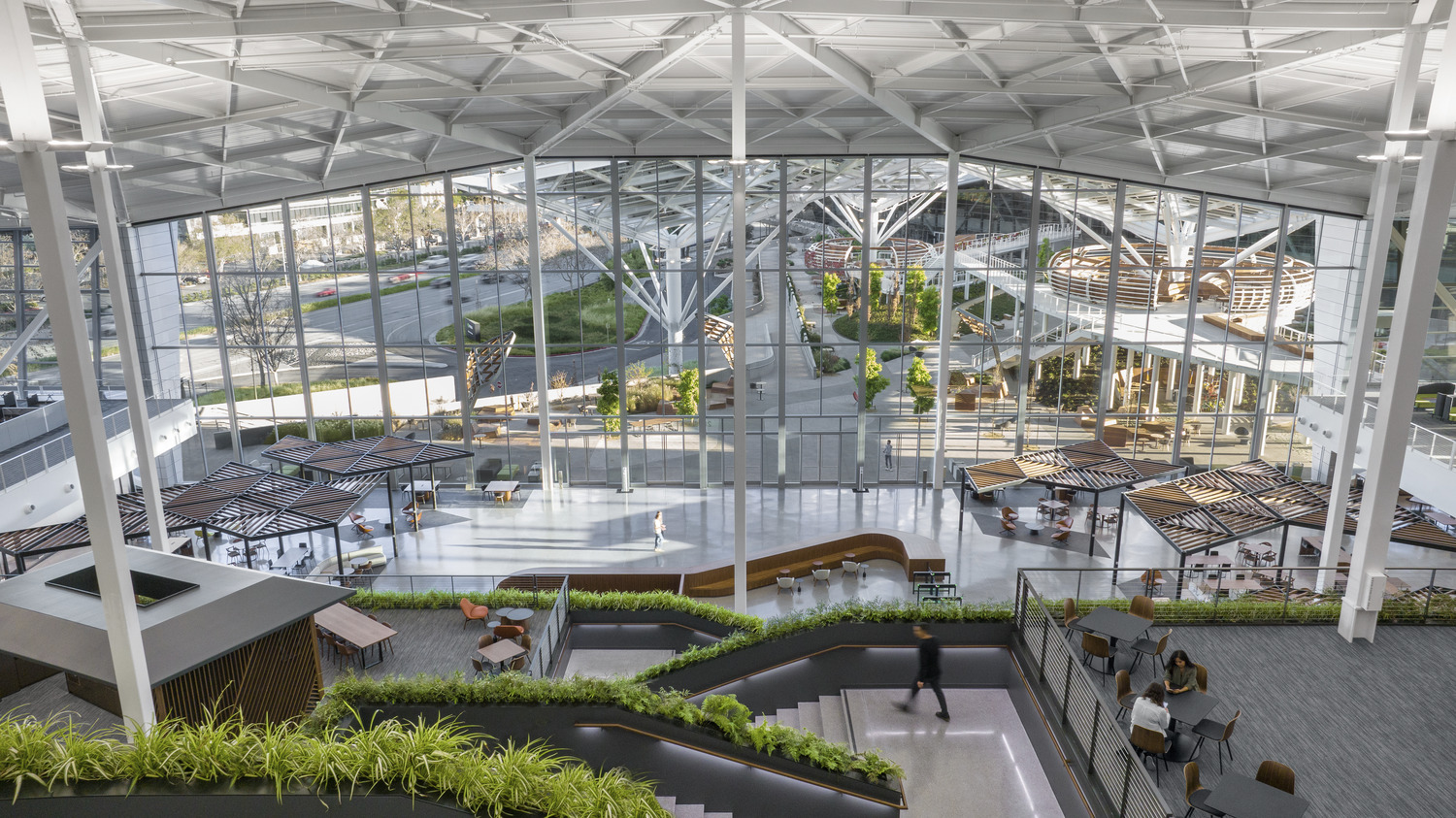
Excellence in Architecture
"The apparent complexity of the structure lent itself to prefabrication and a field assembly that was not dependent on the usual orthogonal cut connections, conventional construction techniques, and the structure’s thinness and the lightness. It can only be done with steel." -- Eddie Jones, FAIA, Founding Principal, Jones Studio, 2024 IDEAS² Awards Judge
Three-dimensional graphics chip manufacturer NVIDIA is in the middle of a multi-step project to overhaul its corporate headquarters in Santa Clara, Calif. The hexagon-shaped Voyager Building is Phase II of the project, which aims to create a workspace that matches NVIDIA’s core beliefs and help employees thrive and create in a high-tech environment. The high, cavernous ceilings allow for large, open spaces that invoke the outdoors right next to the more intimate workspaces.
The Voyager building has a 275,000 sq. ft footprint with 700,000 sq. ft of working space to accommodate more than 3,000 employees. It’s laid out on a 70-ft triangular grid system that adds a signature look and design to every element. The overall building consists of a two-level, below-grade garage podium under a large exterior shell enclosing multiple seismically separated interior office building structures.
The building’s unique design highlights the owner’s desire for a meaningful, collaborative space at the center of the building--named “the mountain”--where a dark gray staircase leads to mezzanine levels. The reception “base camp” area is on one side of the mountain, with more conventional offices, dining area, and meeting spaces on the other. The 60-ft-tall ceiling features numerous triangular skylights, and the undulating roof structure lets in enough natural light to the center atrium to give employees a feeling of being outdoors.
Structural steel was the only viable option to meet the owner’s desire for the open space with long spans and a seemingly floating roof canopy structure. The design also needed to match the structure of the existing Phase I building at the headquarters campus, its equally impressive smaller sibling next door.
The structural steel was incorporated with other materials, such as glass and wood, to open up the workspaces and provide ample light to the open working areas. The open roof structure was left with exposed steel to express the support structure.
The overall building has three structural design elements: the roof, the office buildings, and the parking structure.
The steel roof structure has buckling-restrained braces (BRBs) at the exterior that are seismically separate from the interior steel structures. The roof framing sits on interior columns with a sliding connection at the top of the column, and the roof consists of insulated metal decking.
Due to the limitation in the length of the braces that could be produced, an intermediate beam breaks the lateral elevation into a multi-level brace frame. Columns and beams are designed for the unbraced middle beam out of plan forces. The BRB frames are supported on the concrete podium structure below.
Interior office structures consist of steel framing that rises to four levels at the center. Four independent structures exist under the roof canopy, each entirely seismically independent. The floor consists of concrete-filled metal deck, and the lateral system consists of BRBs.
Voyager’s parking garage is designed on a 62-ft rectangular grid, with the building above built on a 70-ft triangular grid. Matching the framing and translating it to the concrete parking garage was a significant coordination effort among all design team members.
The exterior shell structure is clad in an all-glass façade with a ring of BRB frames and steel columns supporting a horizontal steel truss roofing system. The BRB frames had a maximum brace length of 57 ft with designed stiffness to handle the seismic demand from the trussed roof system and meet the manufacturer’s deflection requirements for the glass façade.
The truss roof structure translates seismic loading from the interior of the building out to the exterior BRB frames, which are supported on the exterior concrete walls and columns. The steel truss roof mimics the equilateral triangular grid system and is supported by up to 60-ft-tall steel columns with a tributary area of 3,100 sq. ft. The undulating roof changes elevation by 30 ft from its lowest to highest points, giving the appearance of rippling water. A cantilever overhang extends more than 30 ft from where the roof meets the exterior façade and is supported by custom tapered W33 steel beams.
The three-level interior office structure was designed to be seismically separate from the exterior shell and was constructed utilizing structural steel and BRB frames, with concrete over metal deck slabs. Seismic isolation was accomplished by providing bearing and slip joints between the interior and exterior beams and columns.
Columns shared by the interior building and roof shell structure were fitted with a bearing pad connection to release any translated seismic loading, allowing the interior and exterior shell structures to move independently and removing any shared seismic loading. The decision to isolate the interior structure from the exterior shell also allowed the interior BRB frames to be downsized, providing reduced steel weights for a more sound and efficient engineering design and reduced cost. Structural engineers also created a custom bearing connection for the roof structure to sit on top of building columns so the exterior brace frames support the lateral load.
The building design also considered the horizontal displacements, including bridges, stairways, and glass panels, which required the structural engineer’s coordination with the glass manufacturers on how much deflection would occur during a seismic event so that the connections that hold the glass in place are designed to accommodate the movement.
The design team worked closely with the fabricator from an early design stage, and the fabricator used state-of-the-art 3D modeling software, which helped the design team resolve difficult aesthetic challenges before they showed up in shop drawings or in the field. That close coordination gave all disciplines time to work through any potential clashes and resolutions early, created opportunities to explore LEED options, and gave the fabricator time to prepare the steel for exposed conditions to ensure a flawless product.
Owner’s representative: NVIDIA, Santa Clara, Calif. General contractor: Devcon Construction, Inc., Milpitas, Calif. Architect: Gensler, San Francisco Structural engineer: IMEG, San Francisco Steel team:
- Fabricator and erector: SME, West Jordan, Utah *AISC full member; AISC-Certified fabricator and erector*
- Detailer: DBM Vircon, Auckland, New Zealand *AISC associate member*
- Location: Santa Clara, CA
- Submitting Firm: IMEG
- Photo Credit: 1, 2, 6, 7, 8 - Jason O'Rear; 3 - IMEG and Devcon; 4, 5 - IMEG
- Search for: Toggle Search
Eureka! NVIDIA Research Breakthrough Puts New Spin on Robot Learning
A new AI agent developed by NVIDIA Research that can teach robots complex skills has trained a robotic hand to perform rapid pen-spinning tricks — for the first time as well as a human can.
The stunning prestidigitation, showcased in the video above, is one of nearly 30 tasks that robots have learned to expertly accomplish thanks to Eureka, which autonomously writes reward algorithms to train bots.
Eureka has also taught robots to open drawers and cabinets, toss and catch balls, and manipulate scissors, among other tasks.
The Eureka research, published today , includes a paper and the project’s AI algorithms, which developers can experiment with using NVIDIA Isaac Gym , a physics simulation reference application for reinforcement learning research. Isaac Gym is built on NVIDIA Omniverse , a development platform for building 3D tools and applications based on the OpenUSD framework. Eureka itself is powered by the GPT-4 large language model .
“Reinforcement learning has enabled impressive wins over the last decade, yet many challenges still exist, such as reward design, which remains a trial-and-error process,” said Anima Anandkumar, senior director of AI research at NVIDIA and an author of the Eureka paper. “Eureka is a first step toward developing new algorithms that integrate generative and reinforcement learning methods to solve hard tasks.”
AI Trains Robots
Eureka-generated reward programs — which enable trial-and-error learning for robots — outperform expert human-written ones on more than 80% of tasks, according to the paper. This leads to an average performance improvement of more than 50% for the bots.
Robot arm taught by Eureka to open a drawer.
The AI agent taps the GPT-4 LLM and generative AI to write software code that rewards robots for reinforcement learning. It doesn’t require task-specific prompting or predefined reward templates — and readily incorporates human feedback to modify its rewards for results more accurately aligned with a developer’s vision.
Using GPU-accelerated simulation in Isaac Gym, Eureka can quickly evaluate the quality of large batches of reward candidates for more efficient training.
Eureka then constructs a summary of the key stats from the training results and instructs the LLM to improve its generation of reward functions. In this way, the AI is self-improving. It’s taught all kinds of robots — quadruped, bipedal, quadrotor, dexterous hands, cobot arms and others — to accomplish all kinds of tasks.
The research paper provides in-depth evaluations of 20 Eureka-trained tasks, based on open-source dexterity benchmarks that require robotic hands to demonstrate a wide range of complex manipulation skills.
The results from nine Isaac Gym environments are showcased in visualizations generated using NVIDIA Omniverse.
Humanoid robot learns a running gait via Eureka.
“Eureka is a unique combination of large language models and NVIDIA GPU-accelerated simulation technologies,” said Linxi “Jim” Fan, senior research scientist at NVIDIA, who’s one of the project’s contributors. “We believe that Eureka will enable dexterous robot control and provide a new way to produce physically realistic animations for artists.”
It’s breakthrough work bound to get developers’ minds spinning with possibilities, adding to recent NVIDIA Research advancements like Voyager , an AI agent built with GPT-4 that can autonomously play Minecraft .
NVIDIA Research comprises hundreds of scientists and engineers worldwide, with teams focused on topics including AI, computer graphics, computer vision, self-driving cars and robotics.
Learn more about Eureka and NVIDIA Research .
Explore generative AI sessions and experiences at NVIDIA GTC , the global conference on AI and accelerated computing, running March 18-21 in San Jose, Calif., and online.
Share on Mastodon
NVIDIA Headquarters, Voyager

Project Location:
Santa Clara, CA
Lighting Designer:
Application:.

White Light
Acesse o melhor conteúdo do seu dia, o único que você precisa.
Inteligência Artificial
Guerra dos chips: nvidia emerge como vencedora na corrida da ia, diz ex-ceo do google, eric schmidt aponta que grandes empresas de tecnologia estão investindo bilhões em centros de dados baseados na nvidia, consolidando a liderança da empresa no setor.
:format(webp))
Para ex-CEO do Google, a Nvidia tem aproveitado bem o momento da inteligência artificial para se expandir. (Jonathan Raa/NurPhoto/Getty Images)
:format(webp))
Redator na Exame
Publicado em 16 de agosto de 2024 às 06h55 .
Última atualização em 16 de agosto de 2024 às 08h43 .
Em uma recente palestra na Universidade de Stanford, Eric Schmidt , ex-CEO do Google , destacou a Nvidia como a principal vencedora da crescente demanda por inteligência artificial (IA). Schmidt revelou que grandes empresas de tecnologia estão se preparando para realizar investimentos bilionários em centros de dados de IA baseados nos chips da Nvidia, com valores estimados entre US$ 20 bilhões (R$ 110 bilhões) e US$ 100 bilhões (R$ 550 bilhões) por empresa . As informações são da CNBC.
Schmidt, que ocupou a posição de CEO do Google de 2001 a 2011 e continuou no conselho da empresa até 2019, discutiu como a Nvidia tem se beneficiado do boom da IA, especialmente após a explosão da IA generativa no final de 2022. Em um vídeo publicado por Stanford , Schmidt destacou o crescimento contínuo da Nvidia, que viu sua receita aumentar mais de 200% durante três trimestres consecutivos, impulsionada pela alta demanda por seus chips de IA.
Mercado está em rápida expansão
O mercado de chips de IA, dominado pela Nvidia, está em rápida expansão, e Schmidt sugeriu que a empresa se beneficiará enormemente do aumento dos investimentos em infraestrutura de IA. Ele também mencionou os esforços de outras empresas, como o Google, que está desenvolvendo os Tensor Processing Units (TPUs) para competir nesse espaço. No entanto, esses chips ainda estão em estágios iniciais, deixando a Nvidia em uma posição privilegiada.
Durante sua fala, Schmidt observou que a capacidade das grandes empresas de investir pesadamente em chips da Nvidia e em centros de dados de IA pode criar uma lacuna tecnológica significativa em relação às empresas menores. Ele indicou que o mercado está cada vez mais concentrado nas mãos de algumas grandes corporações que têm os recursos necessários para acompanhar o ritmo da inovação.
Schmidt também comentou sobre a Meta , que já adquiriu cerca de 600 mil GPUs da Nvidia para sustentar o desenvolvimento de seus modelos de IA. O CEO da Meta, Mark Zuckerberg, afirmou que os próximos modelos de IA da empresa exigirão uma capacidade computacional ainda maior, o que aponta para uma demanda crescente por chips da Nvidia no futuro .
Outro destaque foi a parceria entre Microsoft e OpenAI , que Schmidt inicialmente questionou, mas que agora ele reconhece como um movimento estratégico que pode tornar a Microsoft uma das empresas mais valiosas do mundo. A parceria resultou na construção de um centro de dados de IA, batizado de "Stargate", com um investimento de R$ 546 bilhões (US$ 100 bilhões).
Schmidt concluiu sua análise destacando a liderança contínua da Nvidia no setor de IA, devido em grande parte à sua linguagem de programação CUDA, que é amplamente utilizada em ferramentas de código aberto por desenvolvedores de IA. Ele também mencionou que a AMD, uma das concorrentes da Nvidia, ainda enfrenta dificuldades com seu software de tradução de código CUDA, o que mantém a Nvidia na vanguarda do mercado de IA.
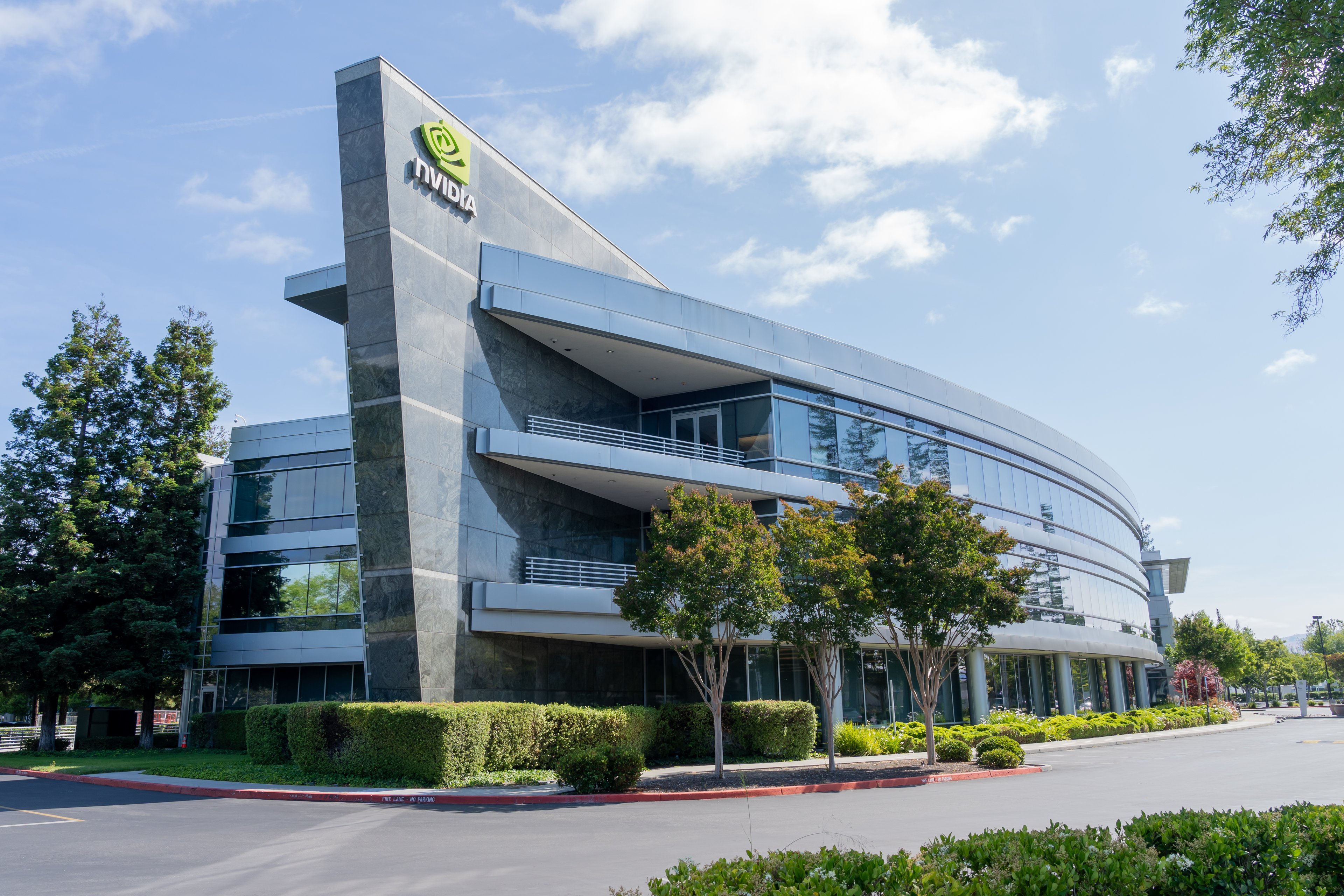
1 /6 A sede da Nvidia em Santa Clara, Califórnia, abriga cerca de 10.000 funcionários e é conhecida por seu design futurista e infraestrutura de ponta, focada em inovação tecnológica. (A sede da Nvidia em Santa Clara, Califórnia, abriga cerca de 10.000 funcionários e é conhecida por seu design futurista e infraestrutura de ponta, focada em inovação tecnológica.)
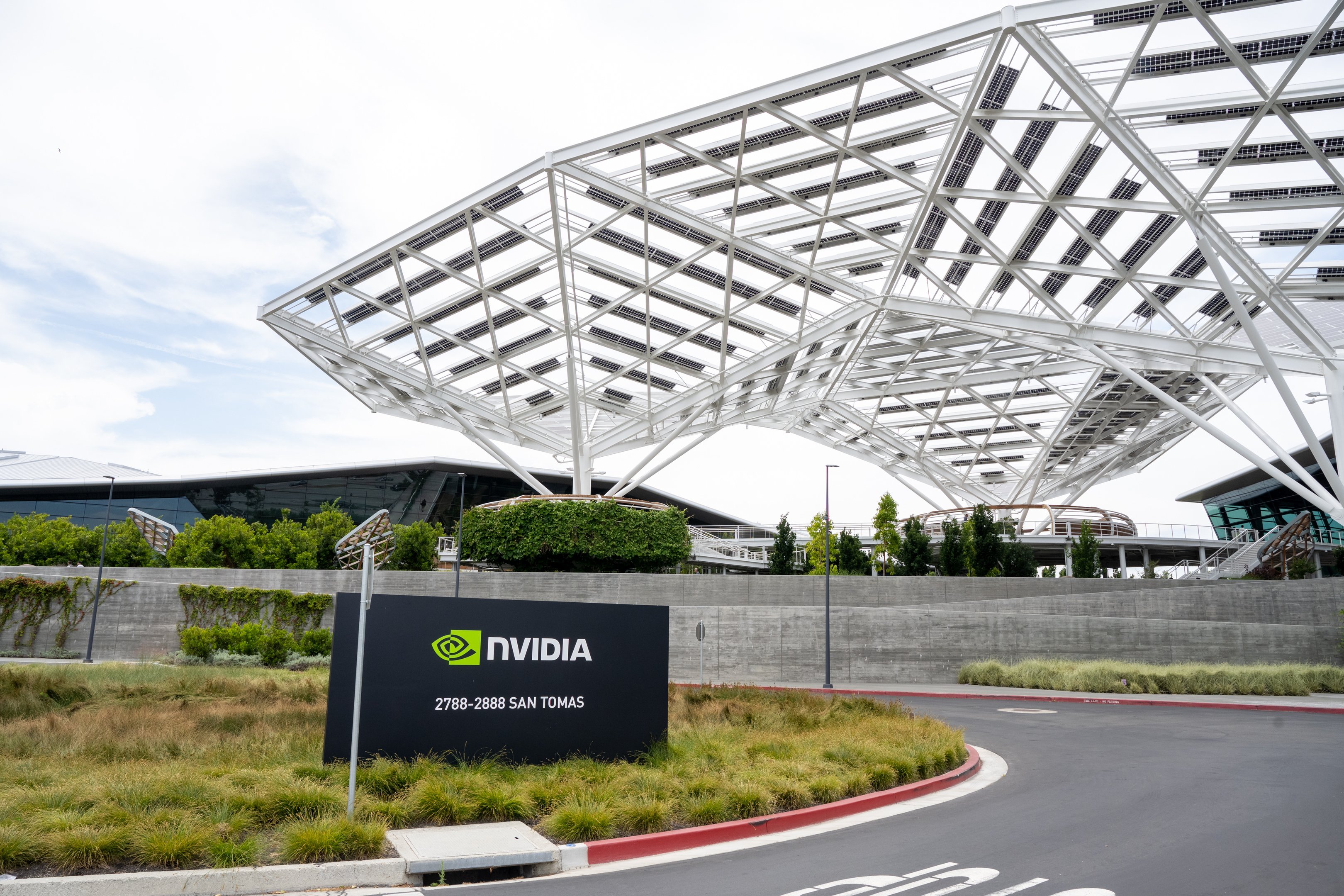
2 /6 Nvidia headquarters in Santa Clara, California, US, on Monday, June 5, 2023. Nvidia Corp., suddenly at the core of the world's most important technology, owns 80% of the market for a particular kind of chip called a data-center accelerator, and the current wait time for one of its AI processors is eight months. Photographer: Marlena Sloss/Bloomberg via Getty Images (O campus inclui laboratórios avançados para pesquisa e desenvolvimento de gráficos computacionais, IA e tecnologias de data center, com mais de 50.000 metros quadrados dedicados à inovação.)
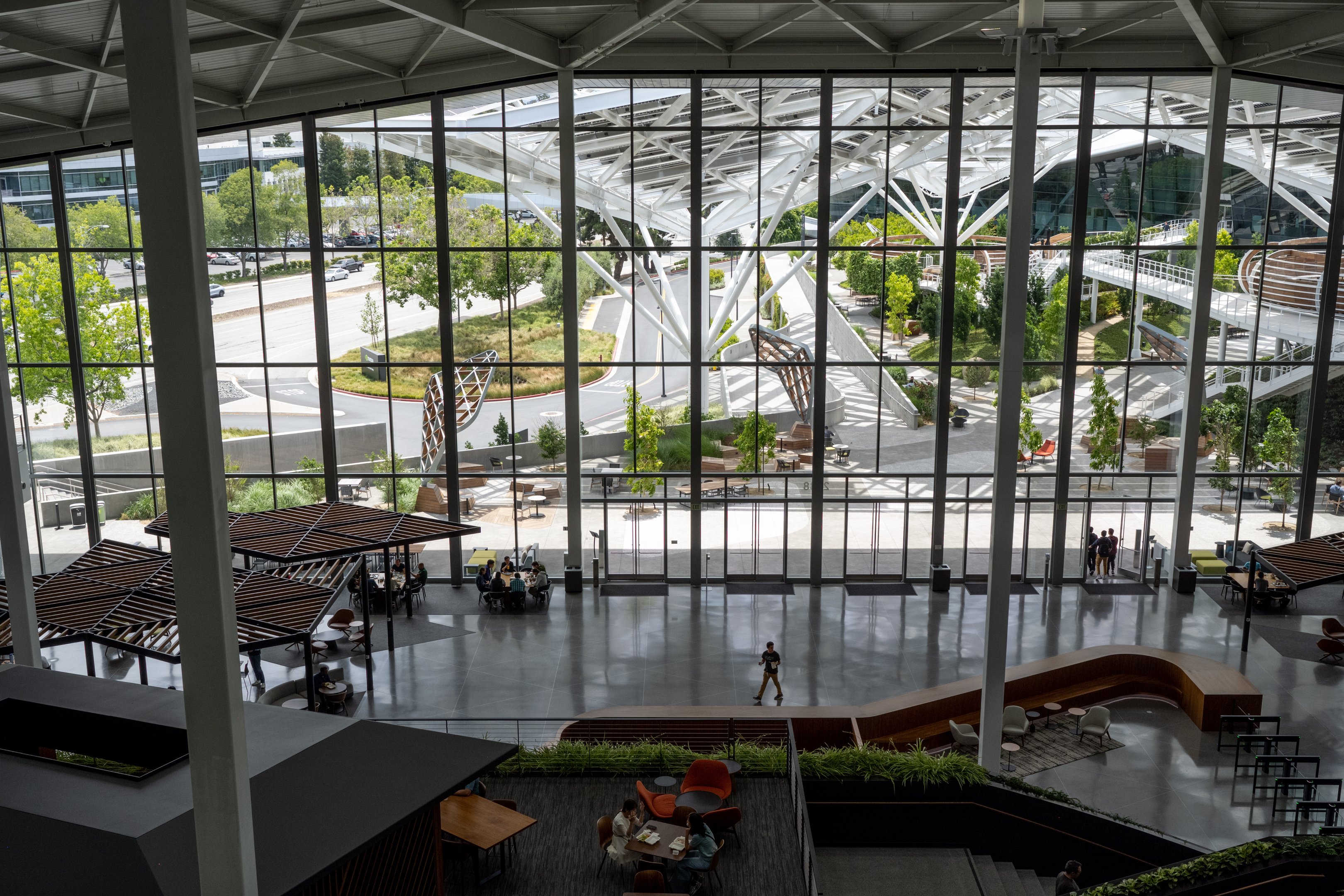
3 /6 Employees inside the Voyager building at Nvidia headquarters in Santa Clara, California, US, on Monday, June 5, 2023. Nvidia Corp., suddenly at the core of the world's most important technology, owns 80% of the market for a particular kind of chip called a data-center accelerator, and the current wait time for one of its AI processors is eight months. Photographer: Marlena Sloss/Bloomberg via Getty Images (Recentemente, a sede passou por expansões significativas, incluindo a construção do prédio Voyager, um edifício de 46.000 metros quadrados projetado para ser um dos mais sustentáveis e tecnologicamente avançados do mundo.)
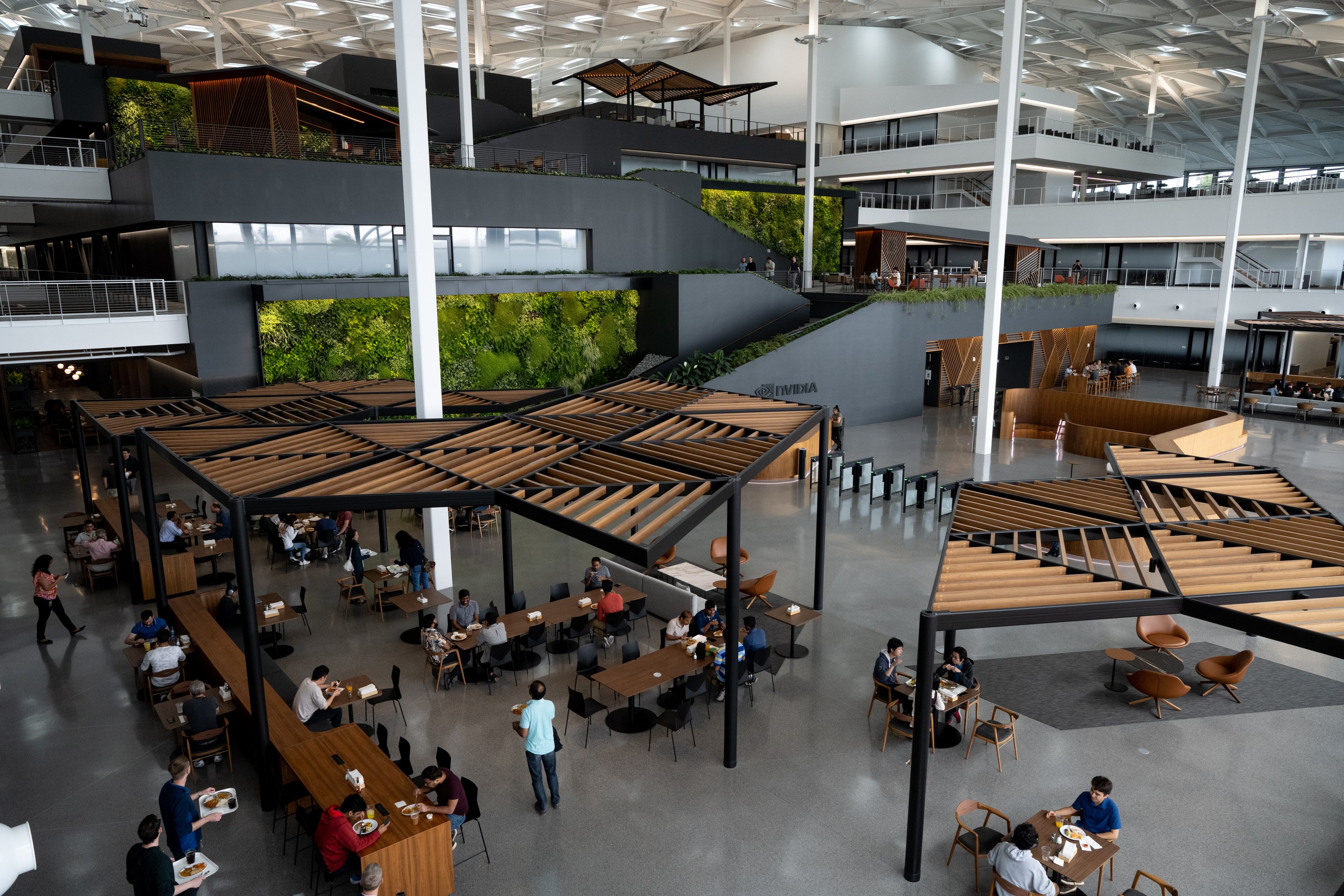
4 /6 Employees inside the Voyager building at Nvidia headquarters in Santa Clara, California, US, on Monday, June 5, 2023. Nvidia Corp., suddenly at the core of the world's most important technology, owns 80% of the market for a particular kind of chip called a data-center accelerator, and the current wait time for one of its AI processors is eight months. Photographer: Marlena Sloss/Bloomberg via Getty Images (A Nvidia é famosa por suas iniciativas verdes, como o uso de energia solar que cobre 75% das necessidades do campus, alinhando-se com seu compromisso com o meio ambiente.)
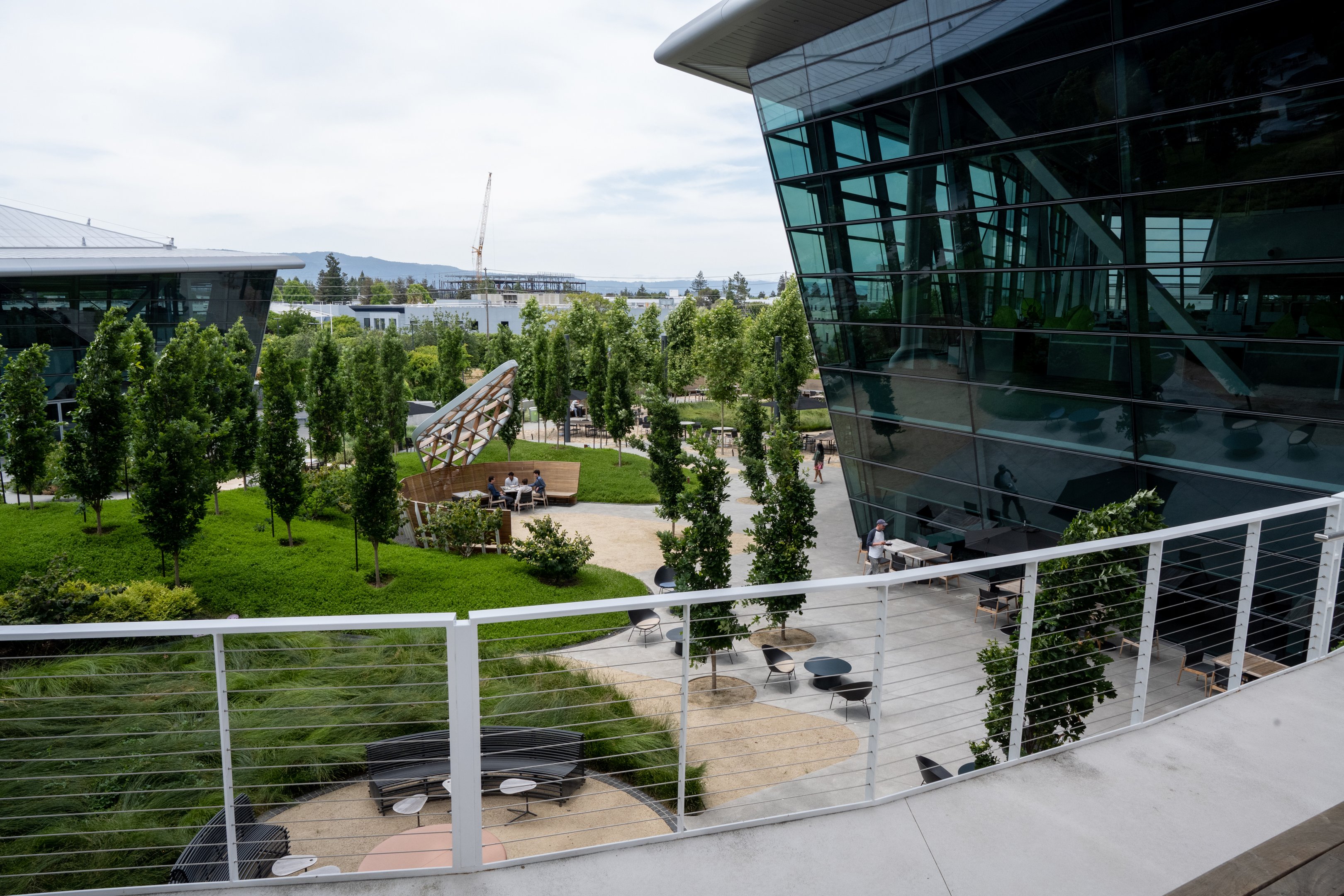
5 /6 Employees at the Voyager Park and Walkway at Nvidia Headquarters in Santa Clara, California, US, on Monday, June 5, 2023. Nvidia Corp., suddenly at the core of the world's most important technology, owns 80% of the market for a particular kind of chip called a data-center accelerator, and the current wait time for one of its AI processors is eight months. Photographer: Marlena Sloss/Bloomberg via Getty Images (O campus possui diversas áreas de lazer e bem-estar, incluindo duas academias, três espaços de meditação e jardins que ocupam mais de 10.000 metros quadrados, promovendo um ambiente de trabalho equilibrado e saudável.)
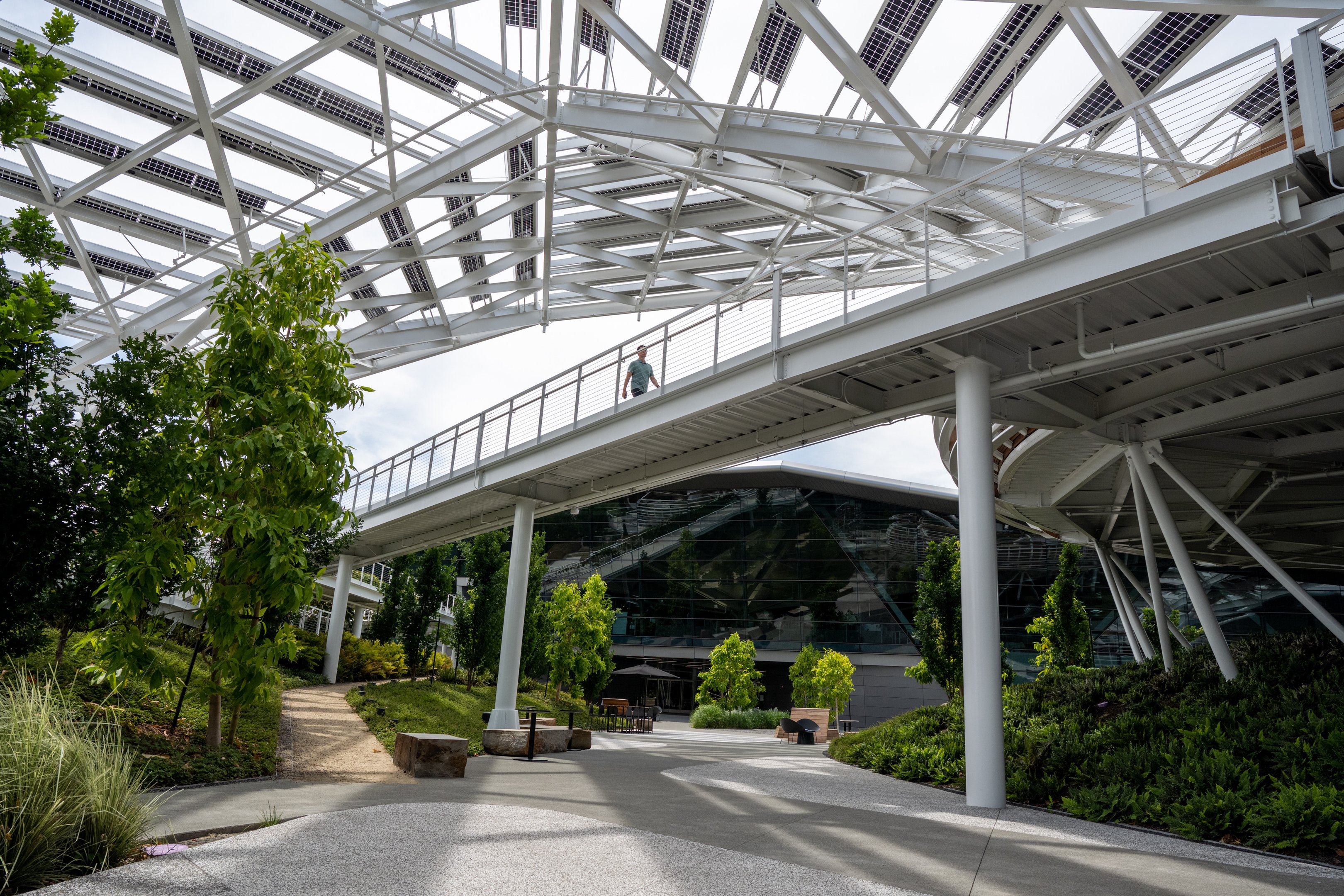
6 /6 The Voyager Park and Walkway at Nvidia Headquarters in Santa Clara, California, US, on Monday, June 5, 2023. Nvidia Corp., suddenly at the core of the world's most important technology, owns 80% of the market for a particular kind of chip called a data-center accelerator, and the current wait time for one of its AI processors is eight months. Photographer: Marlena Sloss/Bloomberg via Getty Images (A sede também inclui um centro de visitantes onde os entusiastas da tecnologia podem conhecer mais sobre as inovações e produtos da Nvidia, recebendo mais de 5.000 visitantes anualmente, tornando-se um ponto de interesse na região.)
Mais de Inteligência Artificial
:format(webp))
Os méritos e as limitações do recém-lançado Plano Brasileiro de Inteligência Artificial
Apesar de previsto um investimento de R$ 23 bilhões até 2028, o Plano Brasileiro de Inteligência Artificial ainda enfrenta lacunas significativas e pode repetir erros do passado
:format(webp))
Elon Musk acelera corrida de IA com lançamento do Grok-2, rival do ChatGPT
Novo modelo da xAI é classificado entre os cinco principais chatbots globais e será oferecido a assinantes da plataforma X.
:format(webp))
Apple cria projeto de "robô de mesa" mirando novas receitas
O dispositivo poderá inclinar a tela para cima e para baixo e girá-la em 360 graus, funcionando como uma versão Apple do Echo Show 10, da Amazon, e o Portal, da Meta, que foi descontinuado
:format(webp))
Como ganhar tempo no trabalho usando inteligência artificial
Cinco automações que podem reduzir o número de tarefas repetitivas feitas durante o dia e liberar tempo para atividades criativas
Mais na Exame
:format(webp))
Melhores e Maiores 2024: Operadoras de planos de saúde aumentam lucro líquido em 191%
:format(webp))
7 frases capacitistas que você deve evitar – e como substituí-las
:format(webp))
B3 divulga 2ª prévia do Ibovespa para janela de setembro a dezembro de 2024; veja como ficou
:format(webp))
Grupo Globo fecha negócio com MGM Resorts e entrará no mercado de apostas esportivas em 2025
- Today's news
- Reviews and deals
- Climate change
- 2024 election
- Newsletters
- Fall allergies
- Health news
- Mental health
- Sexual health
- Family health
- So mini ways
- Unapologetically
- Buying guides
Entertainment
- How to Watch
- My watchlist
- Stock market
- Biden economy
- Personal finance
- Stocks: most active
- Stocks: gainers
- Stocks: losers
- Trending tickers
- World indices
- US Treasury bonds
- Top mutual funds
- Highest open interest
- Highest implied volatility
- Currency converter
- Basic materials
- Communication services
- Consumer cyclical
- Consumer defensive
- Financial services
- Industrials
- Real estate
- Mutual funds
- Credit cards
- Balance transfer cards
- Cash back cards
- Rewards cards
- Travel cards
- Online checking
- High-yield savings
- Money market
- Home equity loan
- Personal loans
- Student loans
- Options pit
- Fantasy football
- Pro Pick 'Em
- College Pick 'Em
- Fantasy baseball
- Fantasy hockey
- Fantasy basketball
- Download the app
- Daily fantasy
- Scores and schedules
- GameChannel
- World Baseball Classic
- Premier League
- CONCACAF League
- Champions League
- Motorsports
- Horse racing
New on Yahoo
- Privacy Dashboard
Watch How NASA Sends Communicates With Voyager 2
Credit: Space.com / animations: NASA/JPL-Caltech/Goddard / produced & edited by Steve Spaleta
Recommended Stories
What one ai ceo learned by working 20 feet from apple's steve jobs.
Steve Jobs has left a lasting impression within the tech community.
Ikea expands its inventory drone fleet
One of the biggest questions looming over the drone space is how to best use the tech. Inspection has become a key driver, as the autonomous copters are deployed to dangerous or remote spaces like power plants and oil rigs. Ingka Group, which owns and operates hundreds of Ikea locations comprising around 90% of its retail sales, is perhaps the most prominent name embracing the emerging space.
'Great space-saver': This 2-tier under-sink organizer is down to $28 at Amazon
The bottom pull-out tray means you'll be able to grab whatever you need, wherever it is.
Yankees OF Alex Verdugo is reportedly allergic to his own tattoos, batting gloves
Not ideal for the Yankees outfielder.
Does my credit card have a routing number?
Credit card numbers don't have routing numbers. Instead, your account number, expiration date, and CVV code are used to identify your account.
The Democratic National Convention is giving influencers media credentials for the first time. Why both campaigns are pivoting to social-first strategies.
200 content creators have been given press credentials to the DNC next week.
What to do if you're stung by jellyfish, stingrays or other dangerous sea creatures
Worried about getting stung by a jellyfish at the beach? Here's how to navigate the worst-case scenario.
Online publishers face a dilemma: Allow AI scraping from Google or lose search visibility
Online publications increasingly face a lose-lose dilemma: allow Google to use their published content to produce inline AI-generated search “answers” or lose visibility in the company’s search engine.
Two action movie simulators Action Hero and Vendetta Forever are headed to VR
Two new action sim games are coming to VR headsets later this year including Action Hero and Vendetta Forever.
These 10 under-$30 finds will take your closet from chaotic to copacetic
With space-saving velvet hangers, roomy storage bins and some extra shelf space, tidying up becomes way less tedious.
Google brings the AI feature that told Americans to eat rocks to six more countries
AI Overviews are now available in India, Japan, Mexico, Indonesia, Brazil and the United Kingdom.
Stoke Space's initial launch plans at Cape Canaveral take shape
Stoke Space is nothing if not ambitious. The five-year-old launch startup has generated a lot of hype due to its bold plans to develop the first fully reusable rocket, with both the booster and second stage vertically returning to Earth. Stoke plans on redeveloping the historic Launch Complex 14, which was home to John Glenn’s historic mission and other NASA programs, in time for its first launch in 2025.
4 thoughts about the 2024 Genesis G90
The 2024 Genesis G90 full-size luxury flagship sedan excels in nearly every way, and it does so for less than the competition.
Capital One Platinum Secured Credit Card review: Build credit with no annual fee
When used responsibly, the Capital One Platinum Secured Credit Card can help you build credit with no annual fee.
Our favorite cheap wireless earbuds drop to just $49
Our pick for the best budget wireless earbuds have dropped back down to their all-time-low price. You can pick up a set of Soundcore by Anker Space A40 earbuds for $49.
Apple opens up NFC transactions to developers, but says there will be 'associated fees'
NFC, or near field communication, is the short-range wireless technology that powers Apple Pay and Wallet. Apple's exclusive access to the iPhone's NFC capabilities had been under investigation by the European Commission for years for restricting competition in the mobile payments space, leading Apple to ultimately open up its tap-and-go technology to third parties in the region. Now, Apple is broadening access to other markets, as well.
Best credit cards for back-to-school shopping at Walmart (August 2024)
You can conquer back-to-school shopping at Walmart with top rewards cards like the Capital One Venture Rewards, Amex Blue Cash Everyday, and Wells Fargo Active Cash.
How to reserve a table at the hottest restaurant with your credit card
Your credit card can help you reserve a table for your next night out — even at notoriously difficult places to get in. Here’s how.
Scientists find evidence of liquid water deep underneath the Martian surface
Water exists on Mars, according to a team of geophysicists, and not just as ice on its poles or as vapor in its atmosphere.
Google introduces new AI-powered features for photo editing and image generation
At Made by Google 2024 on Tuesday, the company launched its new Pixel 9 series of phones. The company is adding features for photo editing, plus new apps for storing and searching through screenshots on-device and an AI-powered studio for image generation. Add Me lets the person taking a group photo be a part of it.

IMAGES
VIDEO
COMMENTS
voyager.mp4. We introduce Voyager, the first LLM-powered embodied lifelong learning agent in Minecraft that continuously explores the world, acquires diverse skills, and makes novel discoveries without human intervention. Voyager consists of three key components: 1) an automatic curriculum that maximizes exploration, 2) an ever-growing skill ...
Voyager is an open-ended AI bot that can autonomously play Minecraft using Chat GPT-4, a large language model. Learn how Voyager leverages GPT-4 to write code, debug errors and develop skills in the game.
See how the graphics and AI company designed a 750,000-square-foot building with a mountain, a volcano and a trellis. The Voyager building is part of Nvidia's Santa Clara campus and aims to give employees a good place to work.
Voyager is a lifelong learning agent that explores, acquires skills, and makes discoveries in Minecraft using GPT-4 as a blackbox query. It uses an automatic curriculum, a skill library, and an iterative prompting mechanism to generate executable code for embodied control.
Nvidia recently opened up its 750,000 sq ft Voyager building. Consumer electronics news site CNet enjoyed access to the "colossal new building" which forms a major part of Nvidia's Santa Clara HQ.
NVIDIA AI. Follow @DrJimFan. Hello there! I am a Senior Research Scientist at NVIDIA and Lead of AI Agents Initiative. My mission is to build generally capable agents across physical worlds (robotics) and virtual worlds (games, simulation) . I share insights about AI research & industry extensively on Twitter/X and LinkedIn.
Take this journey through Voyager, the latest addition to our campus. Check out the features and facilities within—and possibly catch a sneak peek at what we're working on. Also, see photos: https://nvda.ws/39BNX9s
Learn how Voyager, a lifelong learning agent in Minecraft, explores the world, acquires skills, and makes discoveries using large language models.
NVIDIA's newest addition to their headquarters, deemed Voyager, shares its wild, distinctly NVIDIA design inspired by the Pacific Northwest's lush, mountainous terrain. The 750,000 sq ft building is designed to give employees an enjoyable and collaborative place to work. During the development of plans and during construction, Kier + Wright worked closely with NVIDIA, the […]
Nvidia - Voyager contains 170,000 square feet of facade area and is 68 feet high. It was completed in 2022 and is located in Santa Clara, CA. ... The 4-story, 68′ tall Voyager structure has a sloped glass curtain wall perimeter to allow abundant natural light, expansive views, and live plants to build a strong connection to nature, blurring ...
Abstract. We introduce Voyager, the first LLM-powered embodied lifelong learning agent in Minecraft that continuously explores the world, acquires diverse skills, and makes novel discoveries without human intervention. Voyager consists of three key components: 1) an automatic curriculum that maximizes exploration, 2) an ever-growing skill library of executable code for storing and retrieving ...
The hexagon-shaped Voyager Building is Phase II of the project, which aims to create a workspace that matches NVIDIA's core beliefs and help employees thrive and create in a high-tech environment. The high, cavernous ceilings allow for large, open spaces that invoke the outdoors right next to the more intimate workspaces.
Nvidia's headquarters in Santa Clara, California just got a massive new building called Voyager to join the Endeavor — and yes, those are Star Trek references. The graphics and AI company ...
Voyager is an agent that learns skills in Minecraft using GPT-4 and a skill library. It explores the world, acquires diverse skills, and makes novel discoveries without human intervention.
It's breakthrough work bound to get developers' minds spinning with possibilities, adding to recent NVIDIA Research advancements like Voyager, an AI agent built with GPT-4 that can autonomously play Minecraft. NVIDIA Research comprises hundreds of scientists and engineers worldwide, with teams focused on topics including AI, computer ...
Nvidia Voyager. Meet Voyager, 750,000 square feet of workplace, hospitality, nature and the future to welcome Nvidia employees. Project design by Gensler, exterior landscape by Hood Design Studio, and project team members Saris Regis, Devcon Construction and One Workplace helped to give every employee comfort and a view.
Voyager will be 750,000 square feet and situated next to the Endeavor in Santa Clara. This puts the combined buildings' square-footage at 1.25 million, which is a little less than half of Apple's new HQ in Cupertino. Nvidia is still planning the building's staffing, but it expects the building will house its growing engineer teams.
NVIDIA Voyager is a 750,000 square foot building that opened in February 2022. It's part of NVIDIA's Santa Clara headquarters, which includes the 500,000 square foot Endeavor building. The two buildings make up 1.25 million square feet of NVIDIA's headquarters.
Located in Santa Clara, NVIDIA II is the second phase of construction at NVIDIA's California-based headquarters. Designed in a futuristic style, Phase II, called Voyager, is approximately 750,000 square feet and designed to demonstrate design from the inside out. The building's sculptural roof is complex and consists of a series of ...
Novokuznetsk (Russian: Новокузнецк, IPA: [nəvəkʊzˈnʲɛt͡sk], lit. ' new smith's '; Shor: Аба-тура, romanized: Aba-tura) is a city in Kemerovo Oblast (Kuzbass) in southwestern Siberia, Russia.It is the second-largest city in the oblast, after the administrative center Kemerovo.Population: 537,480 (2021 Census); [9] 547,904 (2010 Russian census); [10] 549,870 (2002 Census ...
3/6 Employees inside the Voyager building at Nvidia headquarters in Santa Clara, California, US, on Monday, June 5, 2023. Nvidia Corp., suddenly at the core of the world's most important technology, owns 80% of the market for a particular kind of chip called a data-center accelerator, and the current wait time for one of its AI processors is eight months.
Watch How NASA Sends Communicates With Voyager 2. Space. August 17, 2024 at 9:00 AM. Link Copied. ... Stocks soar, Nvidia surges 12%, as Fed, Powell pave way for September rate cut.
This chapter presents history, economic statistics, and federal government directories of Kemerovo Oblast. Kemerovo Oblast, known as the Kuzbass, is situated in southern central Russia.
Kemerovo Oblast — Kuzbass, also known simply as Kemerovo Oblast (Russian: Ке́меровская о́бласть) or Kuzbass (Кузба́сс), after the Kuznetsk Basin, is a federal subject of Russia (an oblast). Kemerovo is the administrative center and largest city of the oblast. Kemerovo Oblast is one of Russia's most urbanized regions, with over 70% of the population living in its ...
Kemerovo Oblast—Kuzbass is situated in southern central Russia. Krasnoyarsk Krai and Khakasiya lie to the east, Tomsk Oblast to the north, Novosibirsk Oblast to the west, and Altai Krai and the Republic of Altai to the south-west.
Voyager will be using Palantir's Foundry platform. This marks the second strategic space partnership for the company, which also signed an agreement with Starlab Space on June 20, 2024 ...